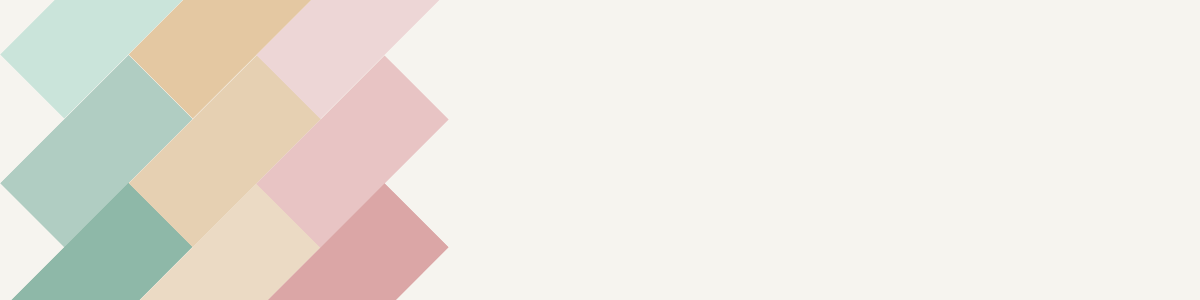
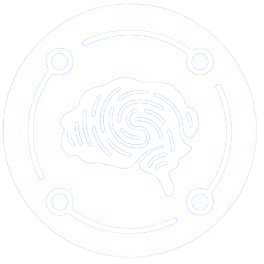
de Recherche et d’Innovation
en Cybersécurité et Société
Ouyed, O.; Allili, M. S.
Group-of-features relevance in multinomial kernel logistic regression and application to human interaction recognition Journal Article
In: Expert Systems with Applications, vol. 148, 2020, ISSN: 09574174, (Publisher: Elsevier Ltd).
Abstract | Links | BibTeX | Tags: Arts computing, Computationally efficient, Gradient descent, Gradient methods, Group sparsities, Group-of-features relevance, Human interaction recognition, Multinomial kernels, regression analysis, Relevance weights, State-of-art methods
@article{ouyed_group–features_2020,
title = {Group-of-features relevance in multinomial kernel logistic regression and application to human interaction recognition},
author = {O. Ouyed and M. S. Allili},
url = {https://www.scopus.com/inward/record.uri?eid=2-s2.0-85078662750&doi=10.1016%2fj.eswa.2020.113247&partnerID=40&md5=8738cfe1a8a6ded1d5f247b8c62de724},
doi = {10.1016/j.eswa.2020.113247},
issn = {09574174},
year = {2020},
date = {2020-01-01},
journal = {Expert Systems with Applications},
volume = {148},
abstract = {We propose an approach for human interaction recognition (HIR) in videos using multinomial kernel logistic regression with group-of-features relevance (GFR-MKLR). Our approach couples kernel and group sparsity modelling to ensure highly precise interaction classification. The group structure in GFR-MKLR is chosen to reflect a representation of interactions at the level of gestures, which ensures more robustness to intra-class variability due to occlusions and changes in subject appearance, body size and viewpoint. The groups consist of motion features extracted from tracking interacting persons joints over time. We encode group sparsity in GFR-MKLR through relevance weights reflecting each group (gesture) discrimination capability between different interaction categories. These weights are automatically estimated during GFR-MKLR training using gradient descent minimisation. Our model is computationally efficient and can be trained on a small training dataset while maintaining a good generalization and interpretation capabilities. Experiments on the well-known UT-Interaction dataset have demonstrated the performance of our approach by comparison with state-of-art methods. © 2020 Elsevier Ltd},
note = {Publisher: Elsevier Ltd},
keywords = {Arts computing, Computationally efficient, Gradient descent, Gradient methods, Group sparsities, Group-of-features relevance, Human interaction recognition, Multinomial kernels, regression analysis, Relevance weights, State-of-art methods},
pubstate = {published},
tppubtype = {article}
}
Nouboukpo, A.; Allili, M. S.
Spatially-coherent segmentation using hierarchical gaussian mixture reduction based on cauchy-schwarz divergence Journal Article
In: Lecture Notes in Computer Science (including subseries Lecture Notes in Artificial Intelligence and Lecture Notes in Bioinformatics), vol. 11662 LNCS, pp. 388–396, 2019, ISSN: 03029743, (ISBN: 9783030272012 Publisher: Springer Verlag).
Abstract | Links | BibTeX | Tags: Cauchy-Schwarz divergence, Foreground segmentation, Gaussian distribution, Gaussian Mixture Model, Gaussian mixture reduction, Image analysis, Image segmentation, Mixture reductions, Reduction algorithms, Reduction techniques, State-of-art methods
@article{nouboukpo_spatially-coherent_2019,
title = {Spatially-coherent segmentation using hierarchical gaussian mixture reduction based on cauchy-schwarz divergence},
author = {A. Nouboukpo and M. S. Allili},
editor = {Campilho A. Yu A. Karray F.},
url = {https://www.scopus.com/inward/record.uri?eid=2-s2.0-85071452890&doi=10.1007%2f978-3-030-27202-9_35&partnerID=40&md5=2689080f7b2410040a038f080ef93bfa},
doi = {10.1007/978-3-030-27202-9_35},
issn = {03029743},
year = {2019},
date = {2019-01-01},
journal = {Lecture Notes in Computer Science (including subseries Lecture Notes in Artificial Intelligence and Lecture Notes in Bioinformatics)},
volume = {11662 LNCS},
pages = {388–396},
abstract = {Gaussian mixture models (GMM) are widely used for image segmentation. The bigger the number in the mixture, the higher will be the data likelihood. Unfortunately, too many GMM components leads to model overfitting and poor segmentation. Thus, there has been a growing interest in GMM reduction algorithms that rely on component fusion while preserving the structure of data. In this work, we present an algorithm based on a closed-form Cauchy-Schwarz divergence for GMM reduction. Contrarily to previous GMM reduction techniques which a single GMM, our approach can lead to multiple small GMMs describing more accurately the structure of the data. Experiments on image foreground segmentation demonstrate the effectiveness of our proposed model compared to state-of-art methods. © Springer Nature Switzerland AG 2019.},
note = {ISBN: 9783030272012
Publisher: Springer Verlag},
keywords = {Cauchy-Schwarz divergence, Foreground segmentation, Gaussian distribution, Gaussian Mixture Model, Gaussian mixture reduction, Image analysis, Image segmentation, Mixture reductions, Reduction algorithms, Reduction techniques, State-of-art methods},
pubstate = {published},
tppubtype = {article}
}