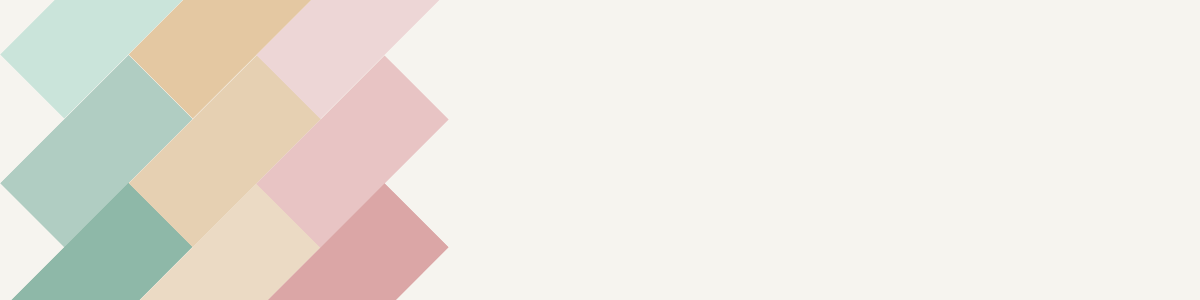
Slide
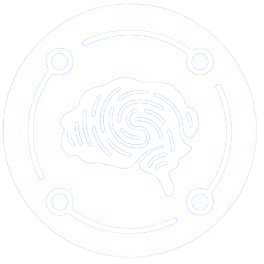
Centre Interdisciplinaire
de Recherche et d’Innovation
en Cybersécurité et Société
de Recherche et d’Innovation
en Cybersécurité et Société
1.
Monthuy-Blanc, J.; Faghihi, U.; Fardshad, M. N. G.; Corno, G.; Iceta, S.; St-Pierre, M. -J.; Bouchard, S.
When Eating Intuitively Is Not Always a Positive Response: Using Machine Learning to Better Unravel Eaters Profiles Journal Article
In: Journal of Clinical Medicine, vol. 12, no. 16, 2023, ISSN: 20770383, (Publisher: Multidisciplinary Digital Publishing Institute (MDPI)).
Abstract | Links | BibTeX | Tags: adult, aged, article, body dissatisfaction, bulimia, causal reasoning, Cluster Analysis, controlled study, coronavirus disease 2019, feeding behavior, female, health survey, human, intuitive eating, Machine learning, major clinical study, male, online analysis, pandemic, self report
@article{monthuy-blanc_when_2023,
title = {When Eating Intuitively Is Not Always a Positive Response: Using Machine Learning to Better Unravel Eaters Profiles},
author = {J. Monthuy-Blanc and U. Faghihi and M. N. G. Fardshad and G. Corno and S. Iceta and M. -J. St-Pierre and S. Bouchard},
url = {https://www.scopus.com/inward/record.uri?eid=2-s2.0-85169079324&doi=10.3390%2fjcm12165172&partnerID=40&md5=2241ae85a40c73e19f30c5c3d10b514a},
doi = {10.3390/jcm12165172},
issn = {20770383},
year = {2023},
date = {2023-01-01},
journal = {Journal of Clinical Medicine},
volume = {12},
number = {16},
abstract = {Background: The aim of the present study was to identify eaters profiles using the latest advantages of Machine Learning approach to cluster analysis. Methods: A total of 317 participants completed an online-based survey including self-reported measures of body image dissatisfaction, bulimia, restraint, and intuitive eating. Analyses were conducted in two steps: (a) identifying an optimal number of clusters, and (b) validating the clustering model of eaters profile using a procedure inspired by the Causal Reasoning approach. Results: This study reveals a 7-cluster model of eaters profiles. The characteristics, needs, and strengths of each eater profile are discussed along with the presentation of a continuum of eaters profiles. Conclusions: This conceptualization of eaters profiles could guide the direction of health education and treatment interventions targeting perceptual and eating dimensions. © 2023 by the authors.},
note = {Publisher: Multidisciplinary Digital Publishing Institute (MDPI)},
keywords = {adult, aged, article, body dissatisfaction, bulimia, causal reasoning, Cluster Analysis, controlled study, coronavirus disease 2019, feeding behavior, female, health survey, human, intuitive eating, Machine learning, major clinical study, male, online analysis, pandemic, self report},
pubstate = {published},
tppubtype = {article}
}
Background: The aim of the present study was to identify eaters profiles using the latest advantages of Machine Learning approach to cluster analysis. Methods: A total of 317 participants completed an online-based survey including self-reported measures of body image dissatisfaction, bulimia, restraint, and intuitive eating. Analyses were conducted in two steps: (a) identifying an optimal number of clusters, and (b) validating the clustering model of eaters profile using a procedure inspired by the Causal Reasoning approach. Results: This study reveals a 7-cluster model of eaters profiles. The characteristics, needs, and strengths of each eater profile are discussed along with the presentation of a continuum of eaters profiles. Conclusions: This conceptualization of eaters profiles could guide the direction of health education and treatment interventions targeting perceptual and eating dimensions. © 2023 by the authors.