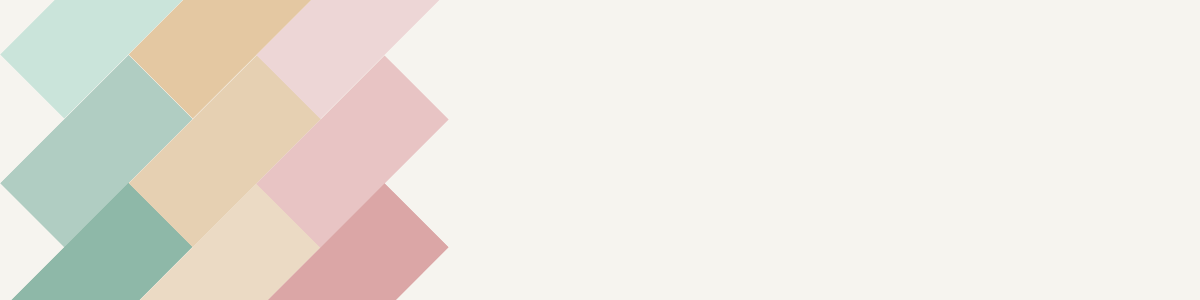
Slide
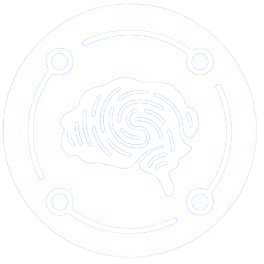
Centre Interdisciplinaire
de Recherche et d’Innovation
en Cybersécurité et Société
de Recherche et d’Innovation
en Cybersécurité et Société
1.
Nouboukpo, A.; Allaoui, M. L.; Allili, M. S.
Multi-scale spatial consistency for deep semi-supervised skin lesion segmentation Journal Article
In: Engineering Applications of Artificial Intelligence, vol. 135, 2024, ISSN: 09521976 (ISSN), (Publisher: Elsevier Ltd).
Abstract | Links | BibTeX | Tags: Deep learning, Dermatology, Image segmentation, Lesion segmentations, Medical imaging, Multi-scales, Semi-supervised, Semi-supervised learning, Skin lesion, Skin lesion segmentation, Spatial consistency, Spatially constrained mixture model, Spatially-constrained mixture models, Supervised learning, UNets, Unlabeled data
@article{nouboukpo_multi-scale_2024,
title = {Multi-scale spatial consistency for deep semi-supervised skin lesion segmentation},
author = {A. Nouboukpo and M. L. Allaoui and M. S. Allili},
url = {https://www.scopus.com/inward/record.uri?eid=2-s2.0-85195700182&doi=10.1016%2fj.engappai.2024.108681&partnerID=40&md5=e1cc2b6a1bb0aed530e8c04583c76167},
doi = {10.1016/j.engappai.2024.108681},
issn = {09521976 (ISSN)},
year = {2024},
date = {2024-01-01},
journal = {Engineering Applications of Artificial Intelligence},
volume = {135},
abstract = {This paper introduces a novel semi-supervised framework, the Multiscale Spatial Consistency Network (MSCNet), for robust semi-supervised skin lesion segmentation. MSCNet uses local and global spatial consistency to leverage a minimal set of labeled data, supplemented by a large number of unlabeled data, to improve segmentation. The model is is based on a single Encoder–Decoder (ED) network, augmented with a Spatially-Constrained Mixture Model (SCMM) to enforce spatial coherence in predictions. To encode the local spatial consistency, a hierarchical superpixel structure is used capture local region context (LRC), bolstering the model capacity to discern fine-grained lesion details. Global consistency is enforced through the SCMM module, which uses a larger context for lesion/background discrimination. In addition, it enables efficient leveraging of the unlabeled data through pseudo-label generation. Experiments demonstrate that the MSCNet outperforms existing state-of-the-art methods in segmenting complex lesions. The MSCNet has an excellent generalization capability, offering a promising direction for semi-supervised medical image segmentation, particularly in scenarios with limited annotated data. The code is available at https://github.com/AdamaTG/MSCNet. © 2024 Elsevier Ltd},
note = {Publisher: Elsevier Ltd},
keywords = {Deep learning, Dermatology, Image segmentation, Lesion segmentations, Medical imaging, Multi-scales, Semi-supervised, Semi-supervised learning, Skin lesion, Skin lesion segmentation, Spatial consistency, Spatially constrained mixture model, Spatially-constrained mixture models, Supervised learning, UNets, Unlabeled data},
pubstate = {published},
tppubtype = {article}
}
This paper introduces a novel semi-supervised framework, the Multiscale Spatial Consistency Network (MSCNet), for robust semi-supervised skin lesion segmentation. MSCNet uses local and global spatial consistency to leverage a minimal set of labeled data, supplemented by a large number of unlabeled data, to improve segmentation. The model is is based on a single Encoder–Decoder (ED) network, augmented with a Spatially-Constrained Mixture Model (SCMM) to enforce spatial coherence in predictions. To encode the local spatial consistency, a hierarchical superpixel structure is used capture local region context (LRC), bolstering the model capacity to discern fine-grained lesion details. Global consistency is enforced through the SCMM module, which uses a larger context for lesion/background discrimination. In addition, it enables efficient leveraging of the unlabeled data through pseudo-label generation. Experiments demonstrate that the MSCNet outperforms existing state-of-the-art methods in segmenting complex lesions. The MSCNet has an excellent generalization capability, offering a promising direction for semi-supervised medical image segmentation, particularly in scenarios with limited annotated data. The code is available at https://github.com/AdamaTG/MSCNet. © 2024 Elsevier Ltd