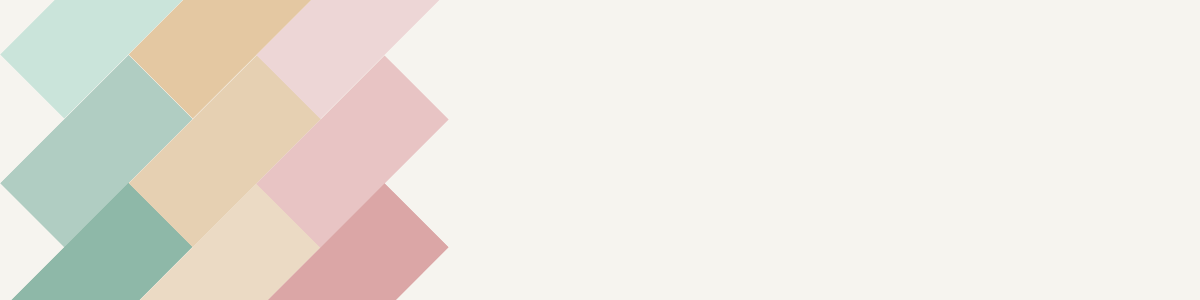
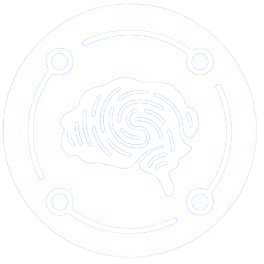
de Recherche et d’Innovation
en Cybersécurité et Société
Boulmerka, A.; Allili, M. S.
Background modeling in videos revisited using finite mixtures of generalized Gaussians and spatial information Proceedings Article
In: Proceedings - International Conference on Image Processing, ICIP, pp. 3660–3664, IEEE Computer Society, 2015, ISBN: 15224880 (ISSN); 978-147998339-1 (ISBN), (Journal Abbreviation: Proc. Int. Conf. Image Process. ICIP).
Abstract | Links | BibTeX | Tags: Background subtraction, Mixture models, spatial information modeling, temporal co-occurrence
@inproceedings{boulmerka_background_2015,
title = {Background modeling in videos revisited using finite mixtures of generalized Gaussians and spatial information},
author = {A. Boulmerka and M. S. Allili},
url = {https://www.scopus.com/inward/record.uri?eid=2-s2.0-84956615464&doi=10.1109%2fICIP.2015.7351487&partnerID=40&md5=e6700460a4cd3a6db31f012307db11f4},
doi = {10.1109/ICIP.2015.7351487},
isbn = {15224880 (ISSN); 978-147998339-1 (ISBN)},
year = {2015},
date = {2015-01-01},
booktitle = {Proceedings - International Conference on Image Processing, ICIP},
volume = {2015-December},
pages = {3660–3664},
publisher = {IEEE Computer Society},
abstract = {This paper presents a new statistical approach combining temporal and spatial information for robust background subtraction (BS) in videos. Temporal information couples finite mixtures of generalized Gaussians (MoGG) and temporal cooccurrence analysis of forground/background data. Spatial information combines multi-scale correlation analysis and histogram matching. Our approach fuses both information to perform efficient BS in the presence of shadows, illumination changes and various complex background dynamics. Comparison with recent state-of-the-art methods on standard datasets has demonstrated the performance of our method in terms of precision and computational efficiency. © 2015 IEEE.},
note = {Journal Abbreviation: Proc. Int. Conf. Image Process. ICIP},
keywords = {Background subtraction, Mixture models, spatial information modeling, temporal co-occurrence},
pubstate = {published},
tppubtype = {inproceedings}
}
Allili, M. S.; Ziou, D.
Using feature selection for object segmentation and tracking Proceedings Article
In: Proceedings - Fourth Canadian Conference on Computer and Robot Vision, CRV 2007, pp. 191–198, Montreal, QC, 2007, ISBN: 0-7695-2786-8 978-0-7695-2786-4.
Abstract | Links | BibTeX | Tags: Active contours, Algorithms, Feature extraction, Feature relevance, Image segmentation, Maximum likelihood, Mixture models, Negative examples, Object of interest (OOI), Optimization, Target tracking
@inproceedings{allili_using_2007,
title = {Using feature selection for object segmentation and tracking},
author = {M. S. Allili and D. Ziou},
url = {https://www.scopus.com/inward/record.uri?eid=2-s2.0-34548781938&doi=10.1109%2fCRV.2007.67&partnerID=40&md5=3fb26f3fcc7a6f55f705255758fef582},
doi = {10.1109/CRV.2007.67},
isbn = {0-7695-2786-8 978-0-7695-2786-4},
year = {2007},
date = {2007-01-01},
booktitle = {Proceedings - Fourth Canadian Conference on Computer and Robot Vision, CRV 2007},
pages = {191–198},
address = {Montreal, QC},
abstract = {Most image segmentation algorithms in the past are based on optimizing an objective function that aims to achieve the similarity between several low-level features to build a partition of the image into homogeneous regions. In the present paper, we propose to incorporate the relevance (selection) of the grouping features to enforce the segmentation toward the capturing of objects of interest. The relevance of the features is determined through a set of positive and negative examples of a specific object defined a priori by the user. The calculation of the relevance of the features is performed by maximizing an objective function defined on the mixture likelihoods of the positive and negative object examples sets. The incorporation of the features relevance in the object segmentation is formulated through an energy functional which is minimized by using level set active contours. We show the efficiency of the approach on several examples of object of interest segmentation and tracking where the features relevance was used. © 2007 IEEE.},
keywords = {Active contours, Algorithms, Feature extraction, Feature relevance, Image segmentation, Maximum likelihood, Mixture models, Negative examples, Object of interest (OOI), Optimization, Target tracking},
pubstate = {published},
tppubtype = {inproceedings}
}
Allili, M. S.; Ziou, D.
Object contour tracking in videos by using adaptive mixture models and shape priors Proceedings Article
In: Proceedings of the International Symposium CompIMAGE 2006 - Computational Modelling of Objects Represented in Images: Fundamentals, Methods and Applications, pp. 47–52, Coimbra, 2007, ISBN: 978-0-415-43349-5.
Abstract | Links | BibTeX | Tags: Active contours, Best fits, Current frames, Image matching, Maximum likelihood, Mixture models, Mixtures, Multi class, Non-static backgrounds, Object contours, Object tracking algorithms, Real video sequences, Robust tracking, Shape informations, Shape priors, Video recording, Video sequences
@inproceedings{allili_object_2007-1,
title = {Object contour tracking in videos by using adaptive mixture models and shape priors},
author = {M. S. Allili and D. Ziou},
url = {https://www.scopus.com/inward/record.uri?eid=2-s2.0-60949085472&partnerID=40&md5=ba63e1abbabcfdd48583b41f700508ef},
isbn = {978-0-415-43349-5},
year = {2007},
date = {2007-01-01},
booktitle = {Proceedings of the International Symposium CompIMAGE 2006 - Computational Modelling of Objects Represented in Images: Fundamentals, Methods and Applications},
pages = {47–52},
address = {Coimbra},
abstract = {In this paper, we propose a novel object tracking algorithm in video sequences. The method is based on object mixture matching between successive frames of the sequence by using active contours. Only the segmentation of the objects in the first frame is required for initialization. The evolution of the object contour on a current frame aims to find the maximum fidelity of the mixture likelihood for the same object between successive frames while having the best fit of the mixture parameters to the homogenous parts of the objects. To permit for a precise and robust tracking, region, boundary and shape information are coupled in the model. The method permits for tracking multi-class objects on cluttered and non-static backgrounds. We validate our approach on examples of tracking performed on real video sequences. © 2007 Taylor & Francis Group.},
keywords = {Active contours, Best fits, Current frames, Image matching, Maximum likelihood, Mixture models, Mixtures, Multi class, Non-static backgrounds, Object contours, Object tracking algorithms, Real video sequences, Robust tracking, Shape informations, Shape priors, Video recording, Video sequences},
pubstate = {published},
tppubtype = {inproceedings}
}
Allili, M. S.; Ziou, D.
Active contours for video object tracking using region, boundary and shape information Journal Article
In: Signal, Image and Video Processing, vol. 1, no. 2, pp. 101–117, 2007, ISSN: 18631711 (ISSN).
Abstract | Links | BibTeX | Tags: Color and infrared, KL-distance, Level sets, Mixture models, Region/Boundary/Shape information, Tracking
@article{allili_active_2007,
title = {Active contours for video object tracking using region, boundary and shape information},
author = {M. S. Allili and D. Ziou},
url = {https://www.scopus.com/inward/record.uri?eid=2-s2.0-34250211807&doi=10.1007%2fs11760-007-0021-8&partnerID=40&md5=a92c80484411a508c57587d27b7d357b},
doi = {10.1007/s11760-007-0021-8},
issn = {18631711 (ISSN)},
year = {2007},
date = {2007-01-01},
journal = {Signal, Image and Video Processing},
volume = {1},
number = {2},
pages = {101–117},
abstract = {In this paper, we propose a robust model for tracking in video sequences with non-static backgrounds. The object boundaries are tracked on each frame of the sequence by minimizing an energy functional that combines region, boundary and shape information. The region information is formulated by minimizing the symmetric Kullback-Leibler (KL) distance between the local and global statistics of the objects versus the background. The boundary information is formulated using a color and texture edge map of the video frames. The shape information is calculated adaptively to the dynamic of the moving objects and permits tracking that is robust to background distractions and occlusions. Minimization of the energy functional is implemented using the level set method. We show the effectiveness of the approach for object tracking in color, infrared (IR), and fused color-infrared sequences. © Springer-Verlag London Limited 2007.},
keywords = {Color and infrared, KL-distance, Level sets, Mixture models, Region/Boundary/Shape information, Tracking},
pubstate = {published},
tppubtype = {article}
}