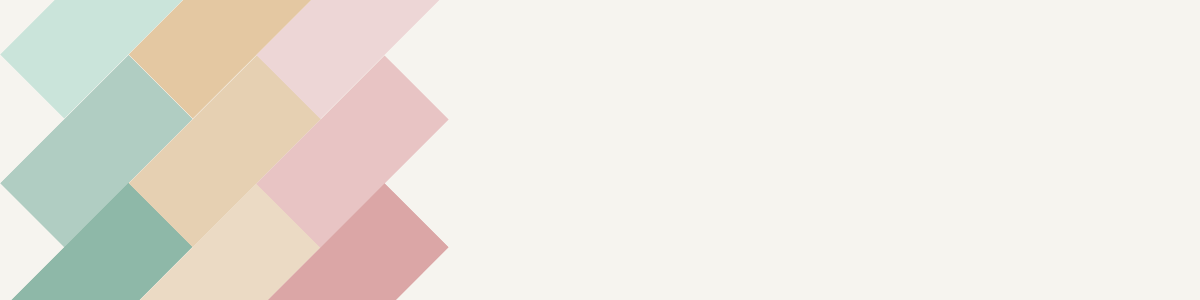
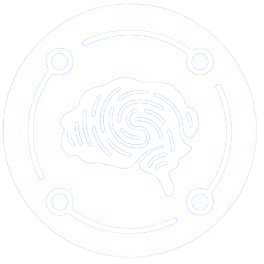
de Recherche et d’Innovation
en Cybersécurité et Société
Murtaza, S. S.; Khreich, W.; Hamou-Lhadj, A.; Gagnon, S.
A trace abstraction approach for host-based anomaly detection Proceedings Article
In: 2015 IEEE Symposium on Computational Intelligence for Security and Defense Applications, CISDA 2015 - Proceedings, pp. 170–177, Institute of Electrical and Electronics Engineers Inc., 2015, ISBN: 978-146737557-3 (ISBN), (Journal Abbreviation: IEEE Symp. Comput. Intell. Secur. Def. Appl., CISDA - Proc.).
Abstract | Links | BibTeX | Tags: Abstracting, Abstraction techniques, Alarm systems, Anomaly detection, Anomaly detection systems, Anomaly detector, Artificial intelligence, Chemical detection, Computer programming languages, Distributed computer systems, Errors, Hidden Markov models, Host-based Anomaly Detection System, Markov processes, Signal detection, Software dependability, Software security, System call traces, Time delay, Time delay embedding, Trace analysis, Trace Analysis and Abstraction
@inproceedings{murtaza_trace_2015,
title = {A trace abstraction approach for host-based anomaly detection},
author = {S. S. Murtaza and W. Khreich and A. Hamou-Lhadj and S. Gagnon},
url = {https://www.scopus.com/inward/record.uri?eid=2-s2.0-84953310135&doi=10.1109%2fCISDA.2015.7208644&partnerID=40&md5=30e47f711b04bc6c44be9f6daea5ebf1},
doi = {10.1109/CISDA.2015.7208644},
isbn = {978-146737557-3 (ISBN)},
year = {2015},
date = {2015-01-01},
booktitle = {2015 IEEE Symposium on Computational Intelligence for Security and Defense Applications, CISDA 2015 - Proceedings},
pages = {170–177},
publisher = {Institute of Electrical and Electronics Engineers Inc.},
abstract = {High false alarm rates and execution times are among the key issues in host-based anomaly detection systems. In this paper, we investigate the use of trace abstraction techniques for reducing the execution time of anomaly detectors while keeping the same accuracy. The key idea is to represent system call traces as traces of kernel module interactions and use the resulting abstract traces as input to known anomaly detection techniques, such as STIDE (the Sequence Time-Delay Embedding) and HMM (Hidden Markov Models). We performed experiments on three datasets, namely, the traditional UNM dataset as well as two modern datasets, Firefox and ADFA-LD. The results show that kernel module traces can lead to similar or fewer false alarms and considerably smaller execution times compared to raw system call traces for host-based anomaly detection systems. © 2015 IEEE.},
note = {Journal Abbreviation: IEEE Symp. Comput. Intell. Secur. Def. Appl., CISDA - Proc.},
keywords = {Abstracting, Abstraction techniques, Alarm systems, Anomaly detection, Anomaly detection systems, Anomaly detector, Artificial intelligence, Chemical detection, Computer programming languages, Distributed computer systems, Errors, Hidden Markov models, Host-based Anomaly Detection System, Markov processes, Signal detection, Software dependability, Software security, System call traces, Time delay, Time delay embedding, Trace analysis, Trace Analysis and Abstraction},
pubstate = {published},
tppubtype = {inproceedings}
}
Pedrocca, P. J.; Allili, M. S.
Real-time people detection in videos using geometrical features and adaptive boosting Journal Article
In: Lecture Notes in Computer Science (including subseries Lecture Notes in Artificial Intelligence and Lecture Notes in Bioinformatics), vol. 6753 LNCS, no. PART 1, pp. 314–324, 2011, ISSN: 03029743, (ISBN: 9783642215926 Place: Burnaby, BC).
Abstract | Links | BibTeX | Tags: Adaboost learning, Adaptive boosting, Change detection algorithms, Feature analysis, Feature extraction, Geometrical features, Geometry, Image analysis, Object recognition, Pedestrian detection, People detection, Real world videos, Signal detection, Video sequences
@article{pedrocca_real-time_2011,
title = {Real-time people detection in videos using geometrical features and adaptive boosting},
author = {P. J. Pedrocca and M. S. Allili},
url = {https://www.scopus.com/inward/record.uri?eid=2-s2.0-79960336661&doi=10.1007%2f978-3-642-21593-3_32&partnerID=40&md5=47ca975800e68648e02f76eba89a7457},
doi = {10.1007/978-3-642-21593-3_32},
issn = {03029743},
year = {2011},
date = {2011-01-01},
journal = {Lecture Notes in Computer Science (including subseries Lecture Notes in Artificial Intelligence and Lecture Notes in Bioinformatics)},
volume = {6753 LNCS},
number = {PART 1},
pages = {314–324},
abstract = {In this paper, we propose a new approach for detecting people in video sequences based on geometrical features and AdaBoost learning. Unlike its predecessors, our approach uses features calculated directly from silhouettes produced by change detection algorithms. Moreover, feature analysis is done part by part for each silhouette, making our approach efficiently applicable for partially-occluded pedestrians and groups of people detection. Experiments on real-world videos showed us the performance of the proposed approach for real-time pedestrian detection. © 2011 Springer-Verlag.},
note = {ISBN: 9783642215926
Place: Burnaby, BC},
keywords = {Adaboost learning, Adaptive boosting, Change detection algorithms, Feature analysis, Feature extraction, Geometrical features, Geometry, Image analysis, Object recognition, Pedestrian detection, People detection, Real world videos, Signal detection, Video sequences},
pubstate = {published},
tppubtype = {article}
}