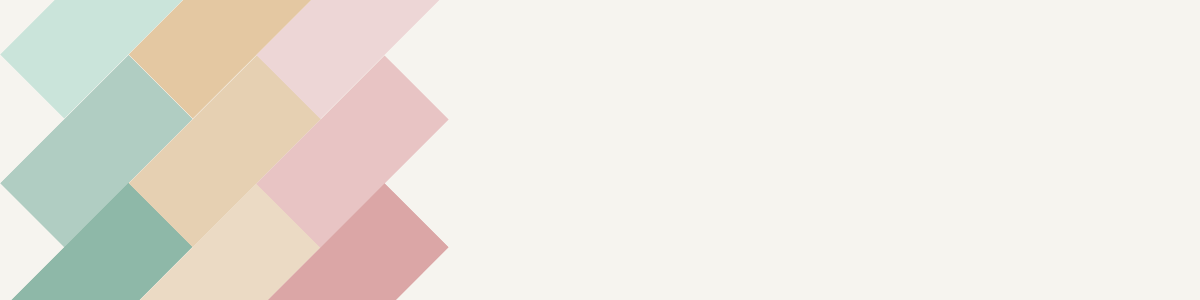
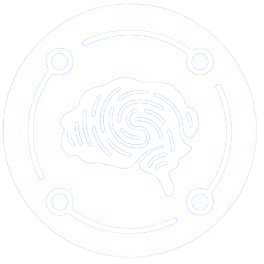
de Recherche et d’Innovation
en Cybersécurité et Société
Saidani, N.; Adi, K.; Allili, M. S.
Semantic Representation Based on Deep Learning for Spam Detection Journal Article
In: Lecture Notes in Computer Science (including subseries Lecture Notes in Artificial Intelligence and Lecture Notes in Bioinformatics), vol. 12056 LNCS, pp. 72–81, 2020, ISSN: 03029743, (ISBN: 9783030453701 Publisher: Springer).
Abstract | Links | BibTeX | Tags: Conceptual views, Deep learning, E-mail spam, Electronic mail, Email content, Learning techniques, Second level, Semantic analysis, Semantic representation, Semantics, Spam detection
@article{saidani_semantic_2020,
title = {Semantic Representation Based on Deep Learning for Spam Detection},
author = {N. Saidani and K. Adi and M. S. Allili},
editor = {Barbeau M. Laborde R. Benzekri A.},
url = {https://www.scopus.com/inward/record.uri?eid=2-s2.0-85083960781&doi=10.1007%2f978-3-030-45371-8_5&partnerID=40&md5=95eba44c33557354be0900bfd2565ca9},
doi = {10.1007/978-3-030-45371-8_5},
issn = {03029743},
year = {2020},
date = {2020-01-01},
journal = {Lecture Notes in Computer Science (including subseries Lecture Notes in Artificial Intelligence and Lecture Notes in Bioinformatics)},
volume = {12056 LNCS},
pages = {72–81},
abstract = {This paper addresses the email spam filtering problem by proposing an approach based on two levels text semantic analysis. In the first level, a deep learning technique, based on Word2Vec is used to categorize emails by specific domains (e.g., health, education, finance, etc.). This enables a separate conceptual view for spams in each domain. In the second level, we extract a set of latent topics from email contents and represent them by rules to summarize the email content into compact topics discriminating spam from legitimate emails in an efficient way. The experimental study shows promising results in term of the precision of the spam detection. © 2020, Springer Nature Switzerland AG.},
note = {ISBN: 9783030453701
Publisher: Springer},
keywords = {Conceptual views, Deep learning, E-mail spam, Electronic mail, Email content, Learning techniques, Second level, Semantic analysis, Semantic representation, Semantics, Spam detection},
pubstate = {published},
tppubtype = {article}
}
Saidani, N.; Adi, K.; Allili, M. S.
A semantic-based classification approach for an enhanced spam detection Journal Article
In: Computers and Security, vol. 94, 2020, ISSN: 01674048 (ISSN), (Publisher: Elsevier Ltd).
Abstract | Links | BibTeX | Tags: Classification, Classification approach, Conceptual views, Domain-specific analysis, Electronic mail, Email content, Multilevel analysis, Semantic analysis, Semantic content, Semantic features, Semantic levels, Semantics, Spam detection
@article{saidani_semantic-based_2020,
title = {A semantic-based classification approach for an enhanced spam detection},
author = {N. Saidani and K. Adi and M. S. Allili},
url = {https://www.scopus.com/inward/record.uri?eid=2-s2.0-85084283123&doi=10.1016%2fj.cose.2020.101716&partnerID=40&md5=539ac0fc0a7144fe983f514175a138e2},
doi = {10.1016/j.cose.2020.101716},
issn = {01674048 (ISSN)},
year = {2020},
date = {2020-01-01},
journal = {Computers and Security},
volume = {94},
abstract = {In this paper, we explore the use of a text semantic analysis to improve the accuracy of spam detection. We propose a method based on two semantic level analysis. In the first level, we categorize emails by specific domains (e.g., Health, Education, Finance, etc.) to enable a separate conceptual view for spams in each domain. In the second level, we combine a set of manually-specified and automatically-extracted semantic features for spam detection in each domain. These features are meant to summarize the email content into compact topics discriminating spam from non-spam emails in an efficient way. We show that the proposed method enables a better spam detection compared to existing methods based on bag-of-words (BoW) and semantic content, and leads to more interpretable results. © 2020},
note = {Publisher: Elsevier Ltd},
keywords = {Classification, Classification approach, Conceptual views, Domain-specific analysis, Electronic mail, Email content, Multilevel analysis, Semantic analysis, Semantic content, Semantic features, Semantic levels, Semantics, Spam detection},
pubstate = {published},
tppubtype = {article}
}