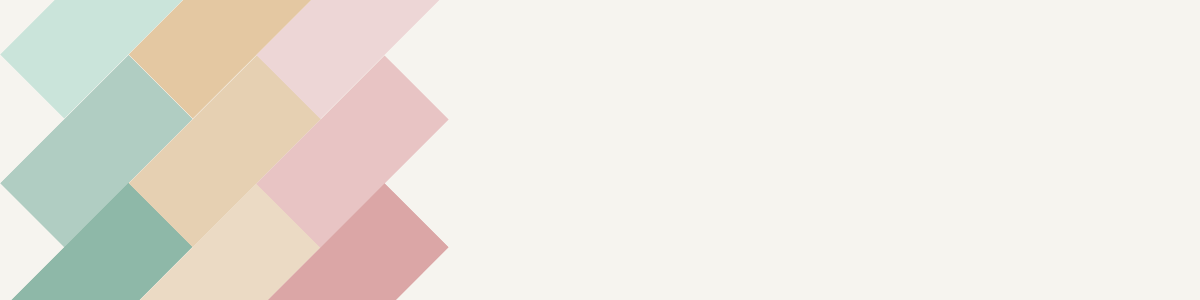
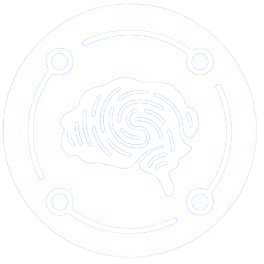
de Recherche et d’Innovation
en Cybersécurité et Société
Hebbache, L.; Amirkhani, D.; Allili, M. S.; Hammouche, N.; Lapointe, J. -F.
Leveraging Saliency in Single-Stage Multi-Label Concrete Defect Detection Using Unmanned Aerial Vehicle Imagery Journal Article
In: Remote Sensing, vol. 15, no. 5, 2023, ISSN: 20724292, (Publisher: MDPI).
Abstract | Links | BibTeX | Tags: Aerial vehicle, Aircraft detection, Antennas, Computational efficiency, Concrete defects, Deep learning, Defect detection, extraction, Feature extraction, Features extraction, Image acquisition, Image Enhancement, Multi-labels, One-stage concrete defect detection, Saliency, Single stage, Unmanned aerial vehicles (UAV), Unmanned areal vehicle imagery
@article{hebbache_leveraging_2023,
title = {Leveraging Saliency in Single-Stage Multi-Label Concrete Defect Detection Using Unmanned Aerial Vehicle Imagery},
author = {L. Hebbache and D. Amirkhani and M. S. Allili and N. Hammouche and J. -F. Lapointe},
url = {https://www.scopus.com/inward/record.uri?eid=2-s2.0-85149966766&doi=10.3390%2frs15051218&partnerID=40&md5=7bf1cb3353270c696c07ff24dc24655d},
doi = {10.3390/rs15051218},
issn = {20724292},
year = {2023},
date = {2023-01-01},
journal = {Remote Sensing},
volume = {15},
number = {5},
abstract = {Visual inspection of concrete structures using Unmanned Areal Vehicle (UAV) imagery is a challenging task due to the variability of defects’ size and appearance. This paper proposes a high-performance model for automatic and fast detection of bridge concrete defects using UAV-acquired images. Our method, coined the Saliency-based Multi-label Defect Detector (SMDD-Net), combines pyramidal feature extraction and attention through a one-stage concrete defect detection model. The attention module extracts local and global saliency features, which are scaled and integrated with the pyramidal feature extraction module of the network using the max-pooling, multiplication, and residual skip connections operations. This has the effect of enhancing the localisation of small and low-contrast defects, as well as the overall accuracy of detection in varying image acquisition ranges. Finally, a multi-label loss function detection is used to identify and localise overlapping defects. The experimental results on a standard dataset and real-world images demonstrated the performance of SMDD-Net with regard to state-of-the-art techniques. The accuracy and computational efficiency of SMDD-Net make it a suitable method for UAV-based bridge structure inspection. © 2023 by the authors.},
note = {Publisher: MDPI},
keywords = {Aerial vehicle, Aircraft detection, Antennas, Computational efficiency, Concrete defects, Deep learning, Defect detection, extraction, Feature extraction, Features extraction, Image acquisition, Image Enhancement, Multi-labels, One-stage concrete defect detection, Saliency, Single stage, Unmanned aerial vehicles (UAV), Unmanned areal vehicle imagery},
pubstate = {published},
tppubtype = {article}
}
Abdollahzadeh, S.; Proulx, P. -L.; Allili, M. S.; Lapointe, J. -F.
Safe Landing Zones Detection for UAVs Using Deep Regression Proceedings Article
In: Proceedings - 2022 19th Conference on Robots and Vision, CRV 2022, pp. 213–218, Institute of Electrical and Electronics Engineers Inc., 2022, ISBN: 978-1-66549-774-9.
Abstract | Links | BibTeX | Tags: Aerial vehicle, Air navigation, Aircraft detection, Antennas, Automatic unmanned aerial vehicle navigation, Deep learning, Deep regression, Landing, Landing zones, Safe landing, Safe landing zone, Semantic segmentation, Semantics, Unmanned aerial vehicles (UAV), Urban areas, Vehicle navigation, Zone detection
@inproceedings{abdollahzadeh_safe_2022,
title = {Safe Landing Zones Detection for UAVs Using Deep Regression},
author = {S. Abdollahzadeh and P. -L. Proulx and M. S. Allili and J. -F. Lapointe},
url = {https://www.scopus.com/inward/record.uri?eid=2-s2.0-85138466098&doi=10.1109%2fCRV55824.2022.00035&partnerID=40&md5=9183f6cd002c8a9068716faf66da72ec},
doi = {10.1109/CRV55824.2022.00035},
isbn = {978-1-66549-774-9},
year = {2022},
date = {2022-01-01},
booktitle = {Proceedings - 2022 19th Conference on Robots and Vision, CRV 2022},
pages = {213–218},
publisher = {Institute of Electrical and Electronics Engineers Inc.},
abstract = {Finding safe landing zones (SLZ) in urban areas and natural scenes is one of the many challenges that must be overcome in automating Unmanned Aerial Vehicles (UAV) navigation. Using passive vision sensors to achieve this objective is a very promising avenue due to their low cost and the potential they provide for performing simultaneous terrain analysis and 3D reconstruction. In this paper, we propose using a deep learning approach on UAV imagery to assess the SLZ. The model is built on a semantic segmentation architecture whereby thematic classes of the terrain are mapped into safety scores for UAV landing. Contrary to past methods, which use hard classification into safe/unsafe landing zones, our approach provides a continuous safety map that is more practical for an emergency landing. Experiments on public datasets have shown promising results. © 2022 IEEE.},
keywords = {Aerial vehicle, Air navigation, Aircraft detection, Antennas, Automatic unmanned aerial vehicle navigation, Deep learning, Deep regression, Landing, Landing zones, Safe landing, Safe landing zone, Semantic segmentation, Semantics, Unmanned aerial vehicles (UAV), Urban areas, Vehicle navigation, Zone detection},
pubstate = {published},
tppubtype = {inproceedings}
}
Davoust, A.; Gavigan, P.; Ruiz-Martin, C.; Trabes, G.; Esfandiari, B.; Wainer, G.; James, J.
An architecture for integrating BDI agents with a simulation environment Journal Article
In: Lecture Notes in Computer Science (including subseries Lecture Notes in Artificial Intelligence and Lecture Notes in Bioinformatics), vol. 12058 LNAI, pp. 67–84, 2020, ISSN: 03029743, (ISBN: 9783030514167 Publisher: Springer).
Abstract | Links | BibTeX | Tags: Antennas, Architecture, Autonomous agents, Belief-desire-intentions, Impedance mismatch, Modelling and simulations, Multi agent systems, Open source architecture, Real time simulations, Separation of concerns, Simulated environment, Simulation environment
@article{davoust_architecture_2020,
title = {An architecture for integrating BDI agents with a simulation environment},
author = {A. Davoust and P. Gavigan and C. Ruiz-Martin and G. Trabes and B. Esfandiari and G. Wainer and J. James},
editor = {Lesperance Y. Bordini R.H. Dennis L.A.},
url = {https://www.scopus.com/inward/record.uri?eid=2-s2.0-85088750329&doi=10.1007%2f978-3-030-51417-4_4&partnerID=40&md5=2f742500bcd9cac1bf054bbc8802e39c},
doi = {10.1007/978-3-030-51417-4_4},
issn = {03029743},
year = {2020},
date = {2020-01-01},
journal = {Lecture Notes in Computer Science (including subseries Lecture Notes in Artificial Intelligence and Lecture Notes in Bioinformatics)},
volume = {12058 LNAI},
pages = {67–84},
abstract = {We present Simulated Autonomous Vehicle Infrastructure (SAVI), an open source architecture for integrating Belief-Desire-Intention (BDI) agents with a simulation platform. This allows for separation of concerns between the development of complex multi-agent behaviours and simulated environments to test them in. We identify and address the impedance mismatch between modelling and simulation, where time is explicitly modelled and differs from “wall clock” time, and BDI systems, where time is not explicitly managed. Our approach avoids linking the environment’s simulation time step to the agents’ reasoning cycles, relying instead on real time simulation where possible, and ensuring that the reasoning module does not get ahead of the simulation. This contributes to a realistic approximation of a real environment for the simulated BDI agents. This is accomplished by running the simulation cycles and the agent reasoning cycles each in their own threads of execution, and managing a single point of contact between these threads. Finally, we illustrate the use of our architecture with a case study involving the simulation of Unmanned Aerial Vehicles (UAVs) following birds. © Springer Nature Switzerland AG 2020.},
note = {ISBN: 9783030514167
Publisher: Springer},
keywords = {Antennas, Architecture, Autonomous agents, Belief-desire-intentions, Impedance mismatch, Modelling and simulations, Multi agent systems, Open source architecture, Real time simulations, Separation of concerns, Simulated environment, Simulation environment},
pubstate = {published},
tppubtype = {article}
}