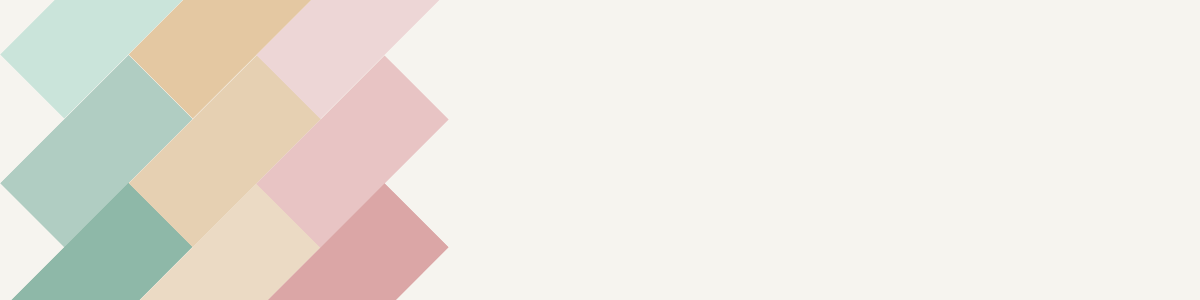
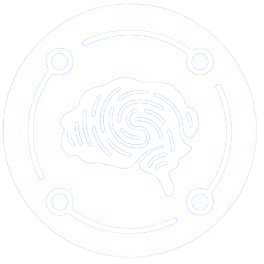
de Recherche et d’Innovation
en Cybersécurité et Société
Filali, I.; Allili, M. S.; Benblidia, N.
Multi-graph based salient object detection Journal Article
In: Lecture Notes in Computer Science (including subseries Lecture Notes in Artificial Intelligence and Lecture Notes in Bioinformatics), vol. 9730, pp. 318–324, 2016, ISSN: 03029743, (ISBN: 9783319415000 Publisher: Springer Verlag).
Abstract | Links | BibTeX | Tags: Graphic methods, Image analysis, Image segmentation, Multi-layer graphs, Multi-scale image decomposition, Multiscale segmentation, Natural images, Object detection, Object recognition, Objective functions, Saliency map, Salient object detection, Salient objects
@article{filali_multi-graph_2016,
title = {Multi-graph based salient object detection},
author = {I. Filali and M. S. Allili and N. Benblidia},
editor = {Karray F. Campilho A. Campilho A.},
url = {https://www.scopus.com/inward/record.uri?eid=2-s2.0-84978804496&doi=10.1007%2f978-3-319-41501-7_36&partnerID=40&md5=eb519756d2e72245e4131d5dc0b416b5},
doi = {10.1007/978-3-319-41501-7_36},
issn = {03029743},
year = {2016},
date = {2016-01-01},
journal = {Lecture Notes in Computer Science (including subseries Lecture Notes in Artificial Intelligence and Lecture Notes in Bioinformatics)},
volume = {9730},
pages = {318–324},
abstract = {We propose a multi-layer graph based approach for salient object detection in natural images. Starting from a set of multi-scale image decomposition using superpixels, we propose an objective function optimized on a multi-layer graph structure to diffuse saliency from image borders to salient objects. After isolating the object kernel, we enhance the accuracy of our saliency maps through an objectness-like based refinement approach. Beside its simplicity, our algorithm yields very accurate salient objects with clear boundaries. Experiments have shown that our approach outperforms several recent methods dealing with salient object detection. © Springer International Publishing Switzerland 2016.},
note = {ISBN: 9783319415000
Publisher: Springer Verlag},
keywords = {Graphic methods, Image analysis, Image segmentation, Multi-layer graphs, Multi-scale image decomposition, Multiscale segmentation, Natural images, Object detection, Object recognition, Objective functions, Saliency map, Salient object detection, Salient objects},
pubstate = {published},
tppubtype = {article}
}
Allili, M. S.; Ziou, D.
Object of interest segmentation and tracking by using feature selection and active contours Proceedings Article
In: Proceedings of the IEEE Computer Society Conference on Computer Vision and Pattern Recognition, Minneapolis, MN, 2007, ISBN: 1-4244-1180-7 978-1-4244-1180-1, (ISSN: 10636919).
Abstract | Links | BibTeX | Tags: Feature extraction, Image acquisition, Image segmentation, Object recognition, Object segmentation, Objective functions, Optimization
@inproceedings{allili_object_2007,
title = {Object of interest segmentation and tracking by using feature selection and active contours},
author = {M. S. Allili and D. Ziou},
url = {https://www.scopus.com/inward/record.uri?eid=2-s2.0-34948855864&doi=10.1109%2fCVPR.2007.383449&partnerID=40&md5=2429a266190c72bb8fb8d3776c444906},
doi = {10.1109/CVPR.2007.383449},
isbn = {1-4244-1180-7 978-1-4244-1180-1},
year = {2007},
date = {2007-01-01},
booktitle = {Proceedings of the IEEE Computer Society Conference on Computer Vision and Pattern Recognition},
address = {Minneapolis, MN},
abstract = {Most image segmentation algorithms in the past are based on optimizing an objective function that aims to achieve the similarity between several low-level features to build a partition of the image into homogeneous regions. In the present paper, we propose to incorporate the relevance (selection) of the grouping features to enforce the segmentation toward the capturing of objects of interest. The relevance of the features is determined through a set of positive and negative examples of a specific object defined a priori by the user. The calculation of the relevance of the features is performed by maximizing an objective function defined on the mixture likelihoods of the positive and negative object examples sets. The incorporation of the features relevance in the object segmentation is formulated through an energy functional which is minimized by using level set active contours. We show the efficiency of the approach on several examples of object of interest segmentation and tracking where the features relevance is used. © 2007 IEEE.},
note = {ISSN: 10636919},
keywords = {Feature extraction, Image acquisition, Image segmentation, Object recognition, Object segmentation, Objective functions, Optimization},
pubstate = {published},
tppubtype = {inproceedings}
}