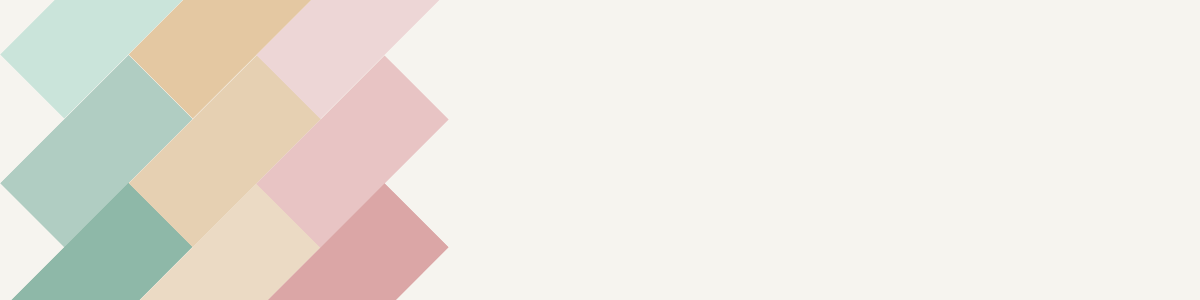
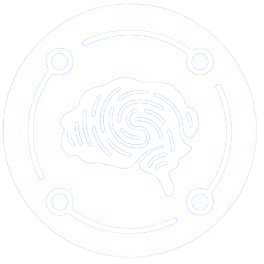
de Recherche et d’Innovation
en Cybersécurité et Société
Filali, I.; Allili, M. S.; Benblidia, N.
Multi-graph based salient object detection Journal Article
In: Lecture Notes in Computer Science (including subseries Lecture Notes in Artificial Intelligence and Lecture Notes in Bioinformatics), vol. 9730, pp. 318–324, 2016, ISSN: 03029743, (ISBN: 9783319415000 Publisher: Springer Verlag).
Abstract | Links | BibTeX | Tags: Graphic methods, Image analysis, Image segmentation, Multi-layer graphs, Multi-scale image decomposition, Multiscale segmentation, Natural images, Object detection, Object recognition, Objective functions, Saliency map, Salient object detection, Salient objects
@article{filali_multi-graph_2016,
title = {Multi-graph based salient object detection},
author = {I. Filali and M. S. Allili and N. Benblidia},
editor = {Karray F. Campilho A. Campilho A.},
url = {https://www.scopus.com/inward/record.uri?eid=2-s2.0-84978804496&doi=10.1007%2f978-3-319-41501-7_36&partnerID=40&md5=eb519756d2e72245e4131d5dc0b416b5},
doi = {10.1007/978-3-319-41501-7_36},
issn = {03029743},
year = {2016},
date = {2016-01-01},
journal = {Lecture Notes in Computer Science (including subseries Lecture Notes in Artificial Intelligence and Lecture Notes in Bioinformatics)},
volume = {9730},
pages = {318–324},
abstract = {We propose a multi-layer graph based approach for salient object detection in natural images. Starting from a set of multi-scale image decomposition using superpixels, we propose an objective function optimized on a multi-layer graph structure to diffuse saliency from image borders to salient objects. After isolating the object kernel, we enhance the accuracy of our saliency maps through an objectness-like based refinement approach. Beside its simplicity, our algorithm yields very accurate salient objects with clear boundaries. Experiments have shown that our approach outperforms several recent methods dealing with salient object detection. © Springer International Publishing Switzerland 2016.},
note = {ISBN: 9783319415000
Publisher: Springer Verlag},
keywords = {Graphic methods, Image analysis, Image segmentation, Multi-layer graphs, Multi-scale image decomposition, Multiscale segmentation, Natural images, Object detection, Object recognition, Objective functions, Saliency map, Salient object detection, Salient objects},
pubstate = {published},
tppubtype = {article}
}
Bacha, S.; Allili, M. S.; Benblidia, N.
Event recognition in photo albums using probabilistic graphical models and feature relevance Journal Article
In: Journal of Visual Communication and Image Representation, vol. 40, no. Part B, pp. 546–558, 2016, ISSN: 10473203 (ISSN), (Publisher: Academic Press Inc.).
Abstract | Links | BibTeX | Tags: Event prediction, Event recognition, Feature relevance, Graphic methods, Object/scene relevance, Photo album, Photo albums, Probabilistic graphical models, Probabilistic graphical models (PGM), Speech recognition, Visual feature
@article{bacha_event_2016,
title = {Event recognition in photo albums using probabilistic graphical models and feature relevance},
author = {S. Bacha and M. S. Allili and N. Benblidia},
url = {https://www.scopus.com/inward/record.uri?eid=2-s2.0-84992111208&doi=10.1016%2fj.jvcir.2016.07.021&partnerID=40&md5=20ebb156819c8fcae6e28949046ceb6e},
doi = {10.1016/j.jvcir.2016.07.021},
issn = {10473203 (ISSN)},
year = {2016},
date = {2016-01-01},
booktitle = {Proceedings - International Conference on Pattern Recognition},
journal = {Journal of Visual Communication and Image Representation},
volume = {40},
number = {Part B},
pages = {546–558},
publisher = {Institute of Electrical and Electronics Engineers Inc.},
abstract = {This paper proposes a method for event recognition in photo albums which aims at predicting the event categories of groups of photos. We propose a probabilistic graphical model (PGM) for event prediction based on high-level visual features consisting of objects and scenes, which are extracted directly from images. For better discrimination between different event categories, we develop a scheme to integrate feature relevance in our model which yields a more powerful inference when album images exhibit a large number of objects and scenes. It allows also to mitigate the influence of non-informative images usually contained in the albums. The performance of the proposed method is validated using extensive experiments on the recently-proposed PEC dataset containing over 61 000 images. Our method obtained the highest accuracy which outperforms previous work. © 2016 Elsevier Inc.},
note = {Publisher: Academic Press Inc.},
keywords = {Event prediction, Event recognition, Feature relevance, Graphic methods, Object/scene relevance, Photo album, Photo albums, Probabilistic graphical models, Probabilistic graphical models (PGM), Speech recognition, Visual feature},
pubstate = {published},
tppubtype = {article}
}
Bacha, S.; Allili, M. S.; Benblidia, N.
Event recognition in photo albums using probabilistic graphical model and feature relevance Proceedings Article
In: Proceedings - International Conference on Pattern Recognition, pp. 2819–2823, Institute of Electrical and Electronics Engineers Inc., 2016, ISBN: 10514651 (ISSN); 978-150904847-2 (ISBN), (Journal Abbreviation: Proc. Int. Conf. Pattern Recognit.).
Abstract | Links | BibTeX | Tags: Discriminative power, Event recognition, Feature relevance, Graphic methods, Image features, Object detection, Photo album, Probabilistic graphical models, Probabilistic graphical models (PGM), Semantic event, Semantics, Speech recognition
@inproceedings{bacha_event_2016-1,
title = {Event recognition in photo albums using probabilistic graphical model and feature relevance},
author = {S. Bacha and M. S. Allili and N. Benblidia},
url = {https://www.scopus.com/inward/record.uri?eid=2-s2.0-85019068722&doi=10.1109%2fICPR.2016.7900063&partnerID=40&md5=7aaf779f00590113afa31fde63016619},
doi = {10.1109/ICPR.2016.7900063},
isbn = {10514651 (ISSN); 978-150904847-2 (ISBN)},
year = {2016},
date = {2016-01-01},
booktitle = {Proceedings - International Conference on Pattern Recognition},
volume = {0},
pages = {2819–2823},
publisher = {Institute of Electrical and Electronics Engineers Inc.},
abstract = {The exponential use of digital cameras has raised a new problem: how to store/retrieve images/albums in very large photo databases that correspond to special events. In this paper, we propose a new probabilistic graphical model (PGM) to recognize events in photo albums stored by users. The PGM combines high-level image features consisting of scenes and objects detected in images. To consider the discriminative power of features, our model integrates the object/scene relevance for more precise prediction of semantic events in photo albums. Experimental results carried out on the challenging PEC dataset with 807 photo albums are presented. © 2016 IEEE.},
note = {Journal Abbreviation: Proc. Int. Conf. Pattern Recognit.},
keywords = {Discriminative power, Event recognition, Feature relevance, Graphic methods, Image features, Object detection, Photo album, Probabilistic graphical models, Probabilistic graphical models (PGM), Semantic event, Semantics, Speech recognition},
pubstate = {published},
tppubtype = {inproceedings}
}
Boulmerka, A.; Allili, M. Saïd; Ait-Aoudia, S.
A generalized multiclass histogram thresholding approach based on mixture modelling Journal Article
In: Pattern Recognition, vol. 47, no. 3, pp. 1330–1348, 2014, ISSN: 00313203.
Abstract | Links | BibTeX | Tags: Arbitrary number, Conditional distribution, Gaussian distribution, Gaussian noise (electronic), Generalized Gaussian Distributions, Graphic methods, Histogram thresholding, Image segmentation, Minimum error thresholding, Mixture-modelling, Mixtures, State-of-the-art techniques, Statistical methods, Thresholding, Thresholding methods
@article{boulmerka_generalized_2014,
title = {A generalized multiclass histogram thresholding approach based on mixture modelling},
author = {A. Boulmerka and M. Saïd Allili and S. Ait-Aoudia},
url = {https://www.scopus.com/inward/record.uri?eid=2-s2.0-84888328869&doi=10.1016%2fj.patcog.2013.09.004&partnerID=40&md5=d8b872bd0abe9e6c4d52439f8ec360bc},
doi = {10.1016/j.patcog.2013.09.004},
issn = {00313203},
year = {2014},
date = {2014-01-01},
journal = {Pattern Recognition},
volume = {47},
number = {3},
pages = {1330–1348},
abstract = {This paper presents a new approach to multi-class thresholding-based segmentation. It considerably improves existing thresholding methods by efficiently modeling non-Gaussian and multi-modal class-conditional distributions using mixtures of generalized Gaussian distributions (MoGG). The proposed approach seamlessly: (1) extends the standard Otsu's method to arbitrary numbers of thresholds and (2) extends the Kittler and Illingworth minimum error thresholding to non-Gaussian and multi-modal class-conditional data. MoGGs enable efficient representation of heavy-tailed data and multi-modal histograms with flat or sharply shaped peaks. Experiments on synthetic data and real-world image segmentation show the performance of the proposed approach with comparison to recent state-of-the-art techniques. © 2013 Elsevier Ltd. All rights reserved.},
keywords = {Arbitrary number, Conditional distribution, Gaussian distribution, Gaussian noise (electronic), Generalized Gaussian Distributions, Graphic methods, Histogram thresholding, Image segmentation, Minimum error thresholding, Mixture-modelling, Mixtures, State-of-the-art techniques, Statistical methods, Thresholding, Thresholding methods},
pubstate = {published},
tppubtype = {article}
}