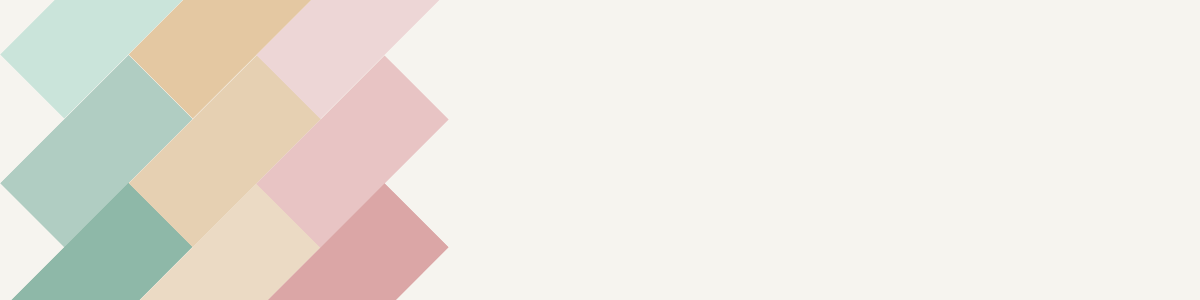
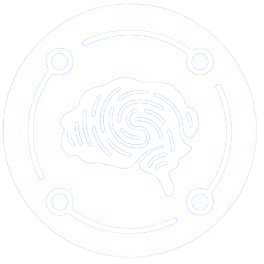
de Recherche et d’Innovation
en Cybersécurité et Société
Ouyed, O.; Allili, M. S.
Recognizing human interactions using group feature relevance in multinomial kernel logistic regression Proceedings Article
In: K., Rus V. Brawner (Ed.): Proceedings of the 31st International Florida Artificial Intelligence Research Society Conference, FLAIRS 2018, pp. 541–545, AAAI press, 2018, ISBN: 978-1-57735-796-4.
Abstract | Links | BibTeX | Tags: Art methods, Artificial intelligence, Feature relevance, Group sparsities, Human interactions, Image features, Kernel logistic regression, Multinomial kernels, regression analysis, Sparse models
@inproceedings{ouyed_recognizing_2018,
title = {Recognizing human interactions using group feature relevance in multinomial kernel logistic regression},
author = {O. Ouyed and M. S. Allili},
editor = {Rus V. Brawner K.},
url = {https://www.scopus.com/inward/record.uri?eid=2-s2.0-85067977954&partnerID=40&md5=1c8d720de570fc565bca3741c107bc9a},
isbn = {978-1-57735-796-4},
year = {2018},
date = {2018-01-01},
booktitle = {Proceedings of the 31st International Florida Artificial Intelligence Research Society Conference, FLAIRS 2018},
pages = {541–545},
publisher = {AAAI press},
abstract = {We propose a supervised approach incorporating group feature sparsity in multi-class kernel logistic regression (GFR-MKLR). The need for group sparsity arises in several practical situations where a subset of a set of factors can explain a predicted variable and each factor consists of a group of variables. We apply our approach for predicting human interactions based on body parts motion (e.g., hands, legs, head, etc.) where image features are organised in groups corresponding to body parts. Our approach, leads to sparse models by assigning weights to groups of features having the highest discrimination between different types of interactions. Experiments conducted on the UT-Interaction dataset have demonstrated the performance of our method with regard to stat-of-art methods. Copyright © 2017, Association for the Advancement of Artificial Intelligence (www.aaai.org). All rights reserved.},
keywords = {Art methods, Artificial intelligence, Feature relevance, Group sparsities, Human interactions, Image features, Kernel logistic regression, Multinomial kernels, regression analysis, Sparse models},
pubstate = {published},
tppubtype = {inproceedings}
}
Bacha, S.; Allili, M. S.; Benblidia, N.
Event recognition in photo albums using probabilistic graphical model and feature relevance Proceedings Article
In: Proceedings - International Conference on Pattern Recognition, pp. 2819–2823, Institute of Electrical and Electronics Engineers Inc., 2016, ISBN: 10514651 (ISSN); 978-150904847-2 (ISBN), (Journal Abbreviation: Proc. Int. Conf. Pattern Recognit.).
Abstract | Links | BibTeX | Tags: Discriminative power, Event recognition, Feature relevance, Graphic methods, Image features, Object detection, Photo album, Probabilistic graphical models, Probabilistic graphical models (PGM), Semantic event, Semantics, Speech recognition
@inproceedings{bacha_event_2016-1,
title = {Event recognition in photo albums using probabilistic graphical model and feature relevance},
author = {S. Bacha and M. S. Allili and N. Benblidia},
url = {https://www.scopus.com/inward/record.uri?eid=2-s2.0-85019068722&doi=10.1109%2fICPR.2016.7900063&partnerID=40&md5=7aaf779f00590113afa31fde63016619},
doi = {10.1109/ICPR.2016.7900063},
isbn = {10514651 (ISSN); 978-150904847-2 (ISBN)},
year = {2016},
date = {2016-01-01},
booktitle = {Proceedings - International Conference on Pattern Recognition},
volume = {0},
pages = {2819–2823},
publisher = {Institute of Electrical and Electronics Engineers Inc.},
abstract = {The exponential use of digital cameras has raised a new problem: how to store/retrieve images/albums in very large photo databases that correspond to special events. In this paper, we propose a new probabilistic graphical model (PGM) to recognize events in photo albums stored by users. The PGM combines high-level image features consisting of scenes and objects detected in images. To consider the discriminative power of features, our model integrates the object/scene relevance for more precise prediction of semantic events in photo albums. Experimental results carried out on the challenging PEC dataset with 807 photo albums are presented. © 2016 IEEE.},
note = {Journal Abbreviation: Proc. Int. Conf. Pattern Recognit.},
keywords = {Discriminative power, Event recognition, Feature relevance, Graphic methods, Image features, Object detection, Photo album, Probabilistic graphical models, Probabilistic graphical models (PGM), Semantic event, Semantics, Speech recognition},
pubstate = {published},
tppubtype = {inproceedings}
}