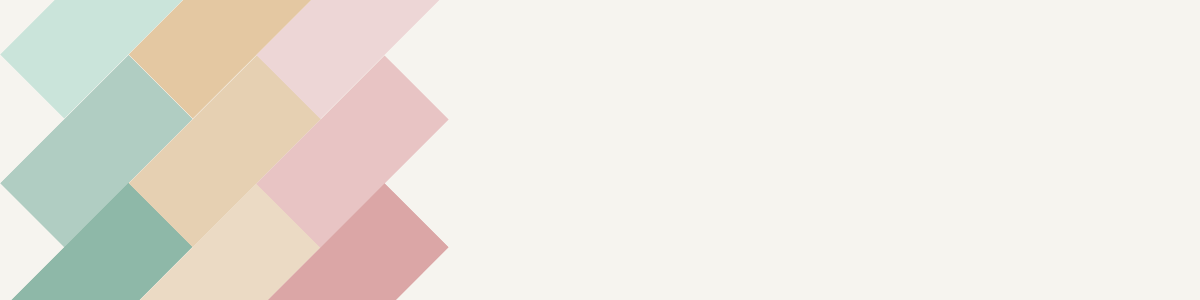
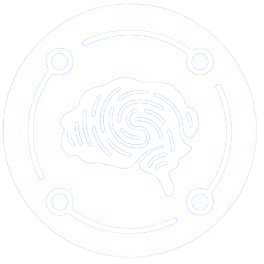
de Recherche et d’Innovation
en Cybersécurité et Société
Allili, M. S.; Bouguila, N.; Ziou, D.
Finite general Gaussian mixture modeling and application to image and video foreground segmentation Journal Article
In: Journal of Electronic Imaging, vol. 17, no. 1, 2008, ISSN: 10179909.
Abstract | Links | BibTeX | Tags: Finite mixture models, Foreground segmentation, Gaussian distribution, Gaussian mixture modeling, Gaussian mixtures, Gaussians, General Gaussian distribution, Image segmentation, Information theory, Information-theoretic approach, Maximum likelihood estimation, Mixture model, Mixtures, Noisy data, Overfitting
@article{allili_finite_2008,
title = {Finite general Gaussian mixture modeling and application to image and video foreground segmentation},
author = {M. S. Allili and N. Bouguila and D. Ziou},
url = {https://www.scopus.com/inward/record.uri?eid=2-s2.0-78149489170&doi=10.1117%2f1.2898125&partnerID=40&md5=8a9b3060dda2366f17b22a06606a9f09},
doi = {10.1117/1.2898125},
issn = {10179909},
year = {2008},
date = {2008-01-01},
journal = {Journal of Electronic Imaging},
volume = {17},
number = {1},
abstract = {We propose a new finite mixture model based on the formalism of general Gaussian distribution (GGD). Because it has the flexibility to adapt to the shape of the data better than the Gaussian, the GGD is less prone to overfitting the number of mixture classes when dealing with noisy data. In the first part of this work, we propose a derivation of the maximum likelihood estimation for the parameters of the new mixture model, and elaborate an information-theoretic approach for the selection of the number of classes. In the second part, we validate the proposed model by comparing it to the Gaussian mixture in applications related to image and video foreground segmentation © 2008 SPIE and IS&T.},
keywords = {Finite mixture models, Foreground segmentation, Gaussian distribution, Gaussian mixture modeling, Gaussian mixtures, Gaussians, General Gaussian distribution, Image segmentation, Information theory, Information-theoretic approach, Maximum likelihood estimation, Mixture model, Mixtures, Noisy data, Overfitting},
pubstate = {published},
tppubtype = {article}
}
Allili, M. S.; Bouguila, N.; Ziou, D.
Online video foreground segmentation using general Gaussian mixture modeling Proceedings Article
In: ICSPC 2007 Proceedings - 2007 IEEE International Conference on Signal Processing and Communications, pp. 959–962, Dubai, 2007, ISBN: 978-1-4244-1236-5.
Abstract | Links | BibTeX | Tags: Bayesian approaches, Bayesian networks, Finite mixture models, Gaussian, Gaussian mixture modeling, Illumination changes, Image segmentation, Mixture of general gaussians (MoGG), Mixtures, MML, On-line estimations, Online videos, Parameter estimation, Signal processing, Trellis codes, Video foreground segmentation
@inproceedings{allili_online_2007,
title = {Online video foreground segmentation using general Gaussian mixture modeling},
author = {M. S. Allili and N. Bouguila and D. Ziou},
url = {https://www.scopus.com/inward/record.uri?eid=2-s2.0-60349106169&doi=10.1109%2fICSPC.2007.4728480&partnerID=40&md5=85c72d00cc58f61baf5ff006dc44957f},
doi = {10.1109/ICSPC.2007.4728480},
isbn = {978-1-4244-1236-5},
year = {2007},
date = {2007-01-01},
booktitle = {ICSPC 2007 Proceedings - 2007 IEEE International Conference on Signal Processing and Communications},
pages = {959–962},
address = {Dubai},
abstract = {In this paper, we propose a robust video foreground modeling by using a finite mixture model of general Gaussian distributions (GGD). The model has a flexibility to model the video background in the presence of sudden illumination changes and shadows, allowing for an efficient foreground segmentation. In a first part of the present work, we propose a derivation of the online estimation of the parameters of the mixture of GGDs and we propose a Bayesian approach for the selection of the number of classes. In a second part, we show experiments of video foreground segmentation demonstrating the performance of the proposed model. © 2007 IEEE.},
keywords = {Bayesian approaches, Bayesian networks, Finite mixture models, Gaussian, Gaussian mixture modeling, Illumination changes, Image segmentation, Mixture of general gaussians (MoGG), Mixtures, MML, On-line estimations, Online videos, Parameter estimation, Signal processing, Trellis codes, Video foreground segmentation},
pubstate = {published},
tppubtype = {inproceedings}
}