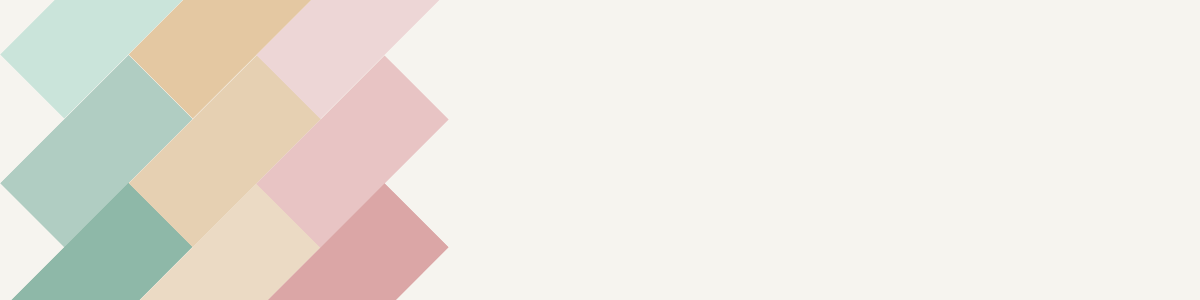
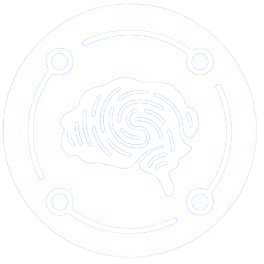
de Recherche et d’Innovation
en Cybersécurité et Société
Allili, M. S.; Bouguila, N.; Ziou, D.
Online video foreground segmentation using general Gaussian mixture modeling Proceedings Article
In: ICSPC 2007 Proceedings - 2007 IEEE International Conference on Signal Processing and Communications, pp. 959–962, Dubai, 2007, ISBN: 978-1-4244-1236-5.
Abstract | Links | BibTeX | Tags: Bayesian approaches, Bayesian networks, Finite mixture models, Gaussian, Gaussian mixture modeling, Illumination changes, Image segmentation, Mixture of general gaussians (MoGG), Mixtures, MML, On-line estimations, Online videos, Parameter estimation, Signal processing, Trellis codes, Video foreground segmentation
@inproceedings{allili_online_2007,
title = {Online video foreground segmentation using general Gaussian mixture modeling},
author = {M. S. Allili and N. Bouguila and D. Ziou},
url = {https://www.scopus.com/inward/record.uri?eid=2-s2.0-60349106169&doi=10.1109%2fICSPC.2007.4728480&partnerID=40&md5=85c72d00cc58f61baf5ff006dc44957f},
doi = {10.1109/ICSPC.2007.4728480},
isbn = {978-1-4244-1236-5},
year = {2007},
date = {2007-01-01},
booktitle = {ICSPC 2007 Proceedings - 2007 IEEE International Conference on Signal Processing and Communications},
pages = {959–962},
address = {Dubai},
abstract = {In this paper, we propose a robust video foreground modeling by using a finite mixture model of general Gaussian distributions (GGD). The model has a flexibility to model the video background in the presence of sudden illumination changes and shadows, allowing for an efficient foreground segmentation. In a first part of the present work, we propose a derivation of the online estimation of the parameters of the mixture of GGDs and we propose a Bayesian approach for the selection of the number of classes. In a second part, we show experiments of video foreground segmentation demonstrating the performance of the proposed model. © 2007 IEEE.},
keywords = {Bayesian approaches, Bayesian networks, Finite mixture models, Gaussian, Gaussian mixture modeling, Illumination changes, Image segmentation, Mixture of general gaussians (MoGG), Mixtures, MML, On-line estimations, Online videos, Parameter estimation, Signal processing, Trellis codes, Video foreground segmentation},
pubstate = {published},
tppubtype = {inproceedings}
}
Allili, M. S.; Bouguila, N.; Ziou, D.
A robust video foreground segmentation by using generalized gaussian mixture modeling Proceedings Article
In: Proceedings - Fourth Canadian Conference on Computer and Robot Vision, CRV 2007, pp. 503–509, Montreal, QC, 2007, ISBN: 0769527868 (ISBN); 978-076952786-4 (ISBN), (Journal Abbreviation: Proc. Fourth Can. Conf. Comput. Robot Vis.).
Abstract | Links | BibTeX | Tags: Bayesian networks, Gaussian mixtures, Image segmentation, Mathematical models, Mixture of general gaussians (MoGG), MML, Video foreground segmentation, Video signal processing
@inproceedings{allili_robust_2007,
title = {A robust video foreground segmentation by using generalized gaussian mixture modeling},
author = {M. S. Allili and N. Bouguila and D. Ziou},
url = {https://www.scopus.com/inward/record.uri?eid=2-s2.0-34548763871&doi=10.1109%2fCRV.2007.7&partnerID=40&md5=e720d61af262e01677a3e23d8c4e6ad0},
doi = {10.1109/CRV.2007.7},
isbn = {0769527868 (ISBN); 978-076952786-4 (ISBN)},
year = {2007},
date = {2007-01-01},
booktitle = {Proceedings - Fourth Canadian Conference on Computer and Robot Vision, CRV 2007},
pages = {503–509},
address = {Montreal, QC},
abstract = {In this paper, we propose a robust video foreground modeling by using a finite mixture model of generalized Gaussian distributions (GDD). The model has a flexibility to model the video background in the presence of sudden illumination changes and shadows, allowing for an efficient foreground segmentation. In a first part of the present work, we propose a derivation of the online estimation of the parameters of the mixture of GDDS and we propose a Bayesian approach for the selection of the number of classes. In a second part, we show experiments of video foreground segmentation demonstrating the performance of the proposed model. © 2007 IEEE.},
note = {Journal Abbreviation: Proc. Fourth Can. Conf. Comput. Robot Vis.},
keywords = {Bayesian networks, Gaussian mixtures, Image segmentation, Mathematical models, Mixture of general gaussians (MoGG), MML, Video foreground segmentation, Video signal processing},
pubstate = {published},
tppubtype = {inproceedings}
}