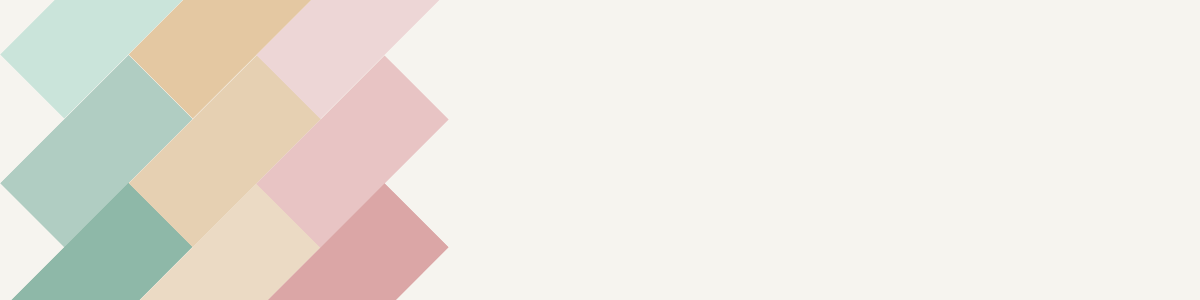
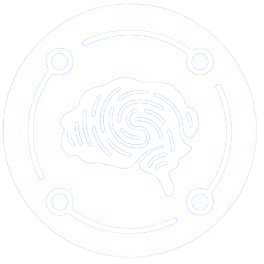
de Recherche et d’Innovation
en Cybersécurité et Société
Allili, M. S.; Ziou, D.
Likelihood-based feature relevance for figure-ground segmentation in images and videos Journal Article
In: Neurocomputing, vol. 167, pp. 658–670, 2015, ISSN: 09252312, (Publisher: Elsevier).
Abstract | Links | BibTeX | Tags: accuracy, algorithm, article, calculation, Feature relevance, Figure-ground segmentations, Gaussian mixture model (GMMs), Image analysis, Image Enhancement, image quality, Image segmentation, Level Set, linear system, mathematical analysis, mathematical model, Negative examples, priority journal, Video cameras, videorecording
@article{allili_likelihood-based_2015,
title = {Likelihood-based feature relevance for figure-ground segmentation in images and videos},
author = {M. S. Allili and D. Ziou},
url = {https://www.scopus.com/inward/record.uri?eid=2-s2.0-84952631642&doi=10.1016%2fj.neucom.2015.04.015&partnerID=40&md5=833948d0784e0dc42c2245b9343971dd},
doi = {10.1016/j.neucom.2015.04.015},
issn = {09252312},
year = {2015},
date = {2015-01-01},
journal = {Neurocomputing},
volume = {167},
pages = {658–670},
abstract = {We propose an efficient method for image/video figure-ground segmentation using feature relevance (FR) and active contours. Given a set of positive and negative examples of a specific foreground (an object of interest (OOI) in an image or a tracked objet in a video), we first learn the foreground distribution model and its characteristic features that best discriminate it from its contextual background. For this goal, an objective function based on feature likelihood ratio is proposed for supervised FR computation. FR is then incorporated in foreground segmentation of new images and videos using level sets and energy minimization. We show the effectiveness of our approach on several examples of image/video figure-ground segmentation. © 2015 Elsevier B.V.},
note = {Publisher: Elsevier},
keywords = {accuracy, algorithm, article, calculation, Feature relevance, Figure-ground segmentations, Gaussian mixture model (GMMs), Image analysis, Image Enhancement, image quality, Image segmentation, Level Set, linear system, mathematical analysis, mathematical model, Negative examples, priority journal, Video cameras, videorecording},
pubstate = {published},
tppubtype = {article}
}
Allili, M. S.; Ziou, D.; Bouguila, N.; Boutemedjet, S.
Image and video segmentation by combining unsupervised generalized Gaussian mixture modeling and feature selection Journal Article
In: IEEE Transactions on Circuits and Systems for Video Technology, vol. 20, no. 10, pp. 1373–1377, 2010, ISSN: 10518215.
Abstract | Links | BibTeX | Tags: Clustering model, Feature extraction, Feature selection, Gaussian distribution, Generalized Gaussian, Heavy-tailed, High dimensional spaces, Image and video segmentation, Image segmentation, image/video segmentation, Minimum message lengths, Real-world image, Video cameras
@article{allili_image_2010,
title = {Image and video segmentation by combining unsupervised generalized Gaussian mixture modeling and feature selection},
author = {M. S. Allili and D. Ziou and N. Bouguila and S. Boutemedjet},
url = {https://www.scopus.com/inward/record.uri?eid=2-s2.0-77957964550&doi=10.1109%2fTCSVT.2010.2077483&partnerID=40&md5=d888c7fe52eff37a5744bccd6a4d3d9e},
doi = {10.1109/TCSVT.2010.2077483},
issn = {10518215},
year = {2010},
date = {2010-01-01},
journal = {IEEE Transactions on Circuits and Systems for Video Technology},
volume = {20},
number = {10},
pages = {1373–1377},
abstract = {In this letter, we propose a clustering model that efficiently mitigates image and video under/over-segmentation by combining generalized Gaussian mixture modeling and feature selection. The model has flexibility to accurately represent heavy-tailed image/video histograms, while automatically discarding uninformative features, leading to better discrimination and localization of regions in high-dimensional spaces. Experimental results on a database of real-world images and videos showed us the effectiveness of the proposed approach. © 2010 IEEE.},
keywords = {Clustering model, Feature extraction, Feature selection, Gaussian distribution, Generalized Gaussian, Heavy-tailed, High dimensional spaces, Image and video segmentation, Image segmentation, image/video segmentation, Minimum message lengths, Real-world image, Video cameras},
pubstate = {published},
tppubtype = {article}
}
Allili, M. S.; Ziou, D.
Object tracking in videos using adaptive mixture models and active contours Journal Article
In: Neurocomputing, vol. 71, no. 10-12, pp. 2001–2011, 2008, ISSN: 09252312.
Abstract | Links | BibTeX | Tags: Active contours, algorithm, Algorithms, article, controlled study, Image analysis, Image processing, imaging system, Level set method, Mathematical models, motion analysis system, Object recognition, priority journal, Set theory, statistical model, Video cameras, Video sequences, videorecording, visual information
@article{allili_object_2008,
title = {Object tracking in videos using adaptive mixture models and active contours},
author = {M. S. Allili and D. Ziou},
url = {https://www.scopus.com/inward/record.uri?eid=2-s2.0-44649197137&doi=10.1016%2fj.neucom.2007.10.019&partnerID=40&md5=a2aef677fae1b220f68c9fd720be3fd5},
doi = {10.1016/j.neucom.2007.10.019},
issn = {09252312},
year = {2008},
date = {2008-01-01},
journal = {Neurocomputing},
volume = {71},
number = {10-12},
pages = {2001–2011},
abstract = {In this paper, we propose a novel object tracking algorithm for video sequences, based on active contours. The tracking is based on matching the object appearance model between successive frames of the sequence using active contours. We formulate the tracking as a minimization of an objective function incorporating region, boundary and shape information. Further, in order to handle variation in object appearance due to self-shadowing, changing illumination conditions and camera geometry, we propose an adaptive mixture model for the object representation. The implementation of the method is based on the level set method. We validate our approach on tracking examples using real video sequences, with comparison to two recent state-of-the-art methods. © 2008 Elsevier B.V. All rights reserved.},
keywords = {Active contours, algorithm, Algorithms, article, controlled study, Image analysis, Image processing, imaging system, Level set method, Mathematical models, motion analysis system, Object recognition, priority journal, Set theory, statistical model, Video cameras, Video sequences, videorecording, visual information},
pubstate = {published},
tppubtype = {article}
}