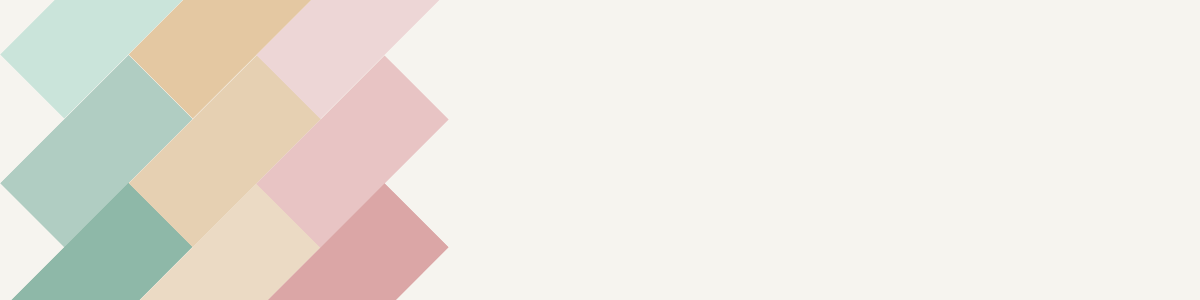
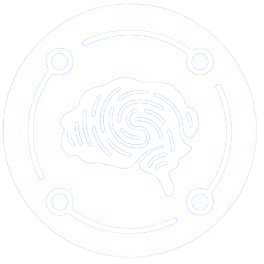
de Recherche et d’Innovation
en Cybersécurité et Société
Bienvenu
If needed, this section can contain some or all of the following:
- A large, engaging image of the university, department, or an abstract representation of the academic field can set a professional and inspiring tone.
- A brief welcome message or introduction that explains what visitors will find on the page. This could be a short paragraph detailing the purpose of the page, such as highlighting the academic and research achievements of the faculty.
- Key facts, achievements or statistics about the professor or department. For instance, number of published papers, years of experience, key projects, or awards won.
- Interactive timeline that highlights major milestones, such as significant publications, awards, and other achievements.
- A short video where the professor introduces themselves and talk about their work and interests providing a personal touch, and making the page more engaging and approachable.
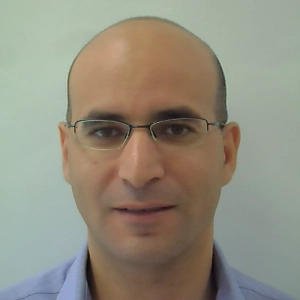
Mohand Said Allili
Professeur
Université du Québec en Outaouais (UQO)
Département d'informatique et d'ingénierie
Mohand Said Allili est professeur titulaire au Département d'Informatique et d'Ingénierie de l'Université du Québec en Outaouais où il dirige le Laboratoire de recherche en Imagerie, Vision et Intelligence Artificielle (LARIVIA). Ses activités de recherche tournent autour de la vision artificielle, de l’apprentissage par ordinateur et le traitement de données multimédias, avec des applications dans l’analyse sémantique des images médicales et aériennes, et la cybersécurité.
Productions incluses dans la recherche:
AUT (Autres), BRE (Brevet), CAC (Publications arbitrées dans des actes de colloque), CNA (Communication non arbitrée), COC (Contribution à un ouvrage collectif), COF (Communication arbitrée), CRE, GRO, LIV (Livre), RAC (Revue avec comité de lecture), RAP (Rapport de recherche), RSC (Revue sans comité de lecture).
Année : 1975 à 2024
Publications sélectionnées
2024 |
Amirkhani, D.; Allili, M. S.; Hebbache, L.; Hammouche, N.; Lapointe, J. Visual Concrete Bridge Defect Classification and Detection Using Deep Learning: A Systematic Review Article de journal Dans: IEEE Transactions on Intelligent Transportation Systems, p. 1–23, 2024, ISSN: 15249050, (Publisher: Institute of Electrical and Electronics Engineers Inc.). @article{amirkhani_visual_2024, Visual inspection is an important process for maintaining bridges in road transportation systems, and preventing catastrophic events and tragedies. In this process, accurate and automatic concrete defect classification and detection are major components to ensure early identification of any issue that can compromise the bridge safety and integrity. While a tremendous body of research has been proposed in the last decades for addressing these problems, the advent of deep learning unleashed huge opportunities for building more accurate and efficient methods. Our aim in this survey is to study the recent progress of vision-based concrete bridge defect classification and detection in the deep learning era. Our review encompasses major aspects underlying typical frameworks, which include concrete defect taxonomy, public datasets and evaluation metrics. We provide also a taxonomy of deep-learning-based classification and detection algorithms with a detailed discussion of their advantages and limitations. We also benchmark baseline models for classification and detection, using two popular datasets. We finally discuss important challenges of concrete defect classification and detection, and promising research avenues to build better models and integrate them in real-world visual inspection systems, which warrant further scientific investigation. IEEE |
Allaoui, M. L.; Allili, M. S. MEDiXNet: A Robust Mixture of Expert Dermatological Imaging Networks for Skin Lesion Segmentation Proceedings Article Dans: IEEE Comput. Soc. Conf. Comput. Vis. Pattern Recogn., IEEE Computer Society, 2024, ISBN: 19457928 (ISSN); 979-835031333-8 (ISBN), (Journal Abbreviation: IEEE Comput. Soc. Conf. Comput. Vis. Pattern Recogn.). @inproceedings{allaoui_medixnet_2024, Skin lesion segmentation in dermatological imaging is crucial for early skin cancer detection. However, it is challenging due to variation in lesion appearance, blurred boundaries, and the presence of artifacts. Existing segmentation methods often fall short in accurately addressing these issues. We present MEDiXNet, a novel deep learning model combining expert networks with the Adaptive Salient Region Attention Module (ASRAM) to specifically tackle these challenges. Tailored for varying lesion types, MEDiXNet leverages ASRAM to enhance focus on critical regions, substantially improving segmentation accuracy. Tested on the ISIC datasets, it achieved a 94% Dice coefficient, surpassing state-of-the-art methods. MEDiXNet's innovative approach represents a significant advancement in dermatological imaging, promising to elevate the precision of skin cancer diagnostics. © 2024 IEEE. |
Nouboukpo, A.; Allaoui, M. L.; Allili, M. S. Multi-scale spatial consistency for deep semi-supervised skin lesion segmentation Article de journal Dans: Engineering Applications of Artificial Intelligence, vol. 135, 2024, ISSN: 09521976 (ISSN), (Publisher: Elsevier Ltd). @article{nouboukpo_multi-scale_2024, This paper introduces a novel semi-supervised framework, the Multiscale Spatial Consistency Network (MSCNet), for robust semi-supervised skin lesion segmentation. MSCNet uses local and global spatial consistency to leverage a minimal set of labeled data, supplemented by a large number of unlabeled data, to improve segmentation. The model is is based on a single Encoder–Decoder (ED) network, augmented with a Spatially-Constrained Mixture Model (SCMM) to enforce spatial coherence in predictions. To encode the local spatial consistency, a hierarchical superpixel structure is used capture local region context (LRC), bolstering the model capacity to discern fine-grained lesion details. Global consistency is enforced through the SCMM module, which uses a larger context for lesion/background discrimination. In addition, it enables efficient leveraging of the unlabeled data through pseudo-label generation. Experiments demonstrate that the MSCNet outperforms existing state-of-the-art methods in segmenting complex lesions. The MSCNet has an excellent generalization capability, offering a promising direction for semi-supervised medical image segmentation, particularly in scenarios with limited annotated data. The code is available at https://github.com/AdamaTG/MSCNet. © 2024 Elsevier Ltd |
Some Heading
If needed, this section can contain some or all of the following:
- Recent news, updates, or upcoming events related to the professor or their department, such as guest lectures, seminars, and conferences.
- Social media feed.
- A quote from the professor about their philosophy on education and research or a testimonial from a peer or student adding a personal and inspirational element to the page, placing this information just above the share icons can give visitors current and relevant reasons to engage and share.
- Call to Action to attend or participate in some even.
- Contact Form
- Subscribe form