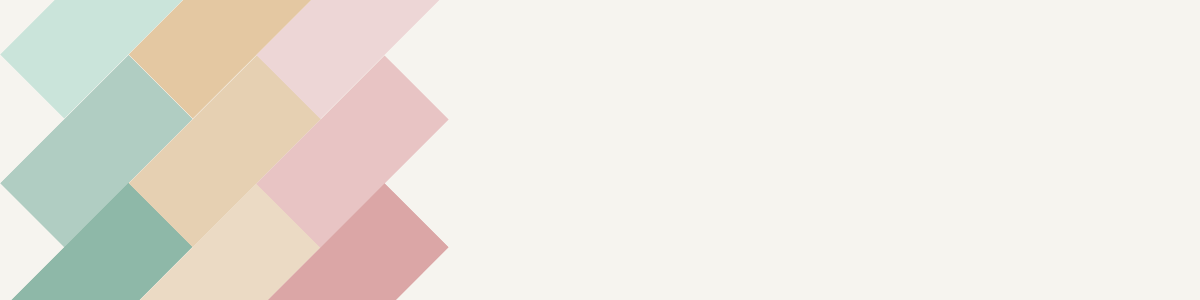
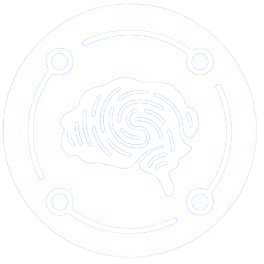
de Recherche et d’Innovation
en Cybersécurité et Société
Yapi, D.; Nouboukpo, A.; Allili, M. S.; Member, IEEE
Mixture of multivariate generalized Gaussians for multi-band texture modeling and representation Journal Article
In: Signal Processing, vol. 209, 2023, ISSN: 01651684, (Publisher: Elsevier B.V.).
Abstract | Links | BibTeX | Tags: Color texture retrieval, Content-based, Content-based color-texture retrieval, Convolution, convolutional neural network, Gaussians, Image retrieval, Image texture, Mixture of multivariate generalized gaussians, Multi-scale Decomposition, Subbands, Texture representation, Textures
@article{yapi_mixture_2023,
title = {Mixture of multivariate generalized Gaussians for multi-band texture modeling and representation},
author = {D. Yapi and A. Nouboukpo and M. S. Allili and IEEE Member},
url = {https://www.scopus.com/inward/record.uri?eid=2-s2.0-85151300047&doi=10.1016%2fj.sigpro.2023.109011&partnerID=40&md5=3bf98e9667eb7b60cb3f59ed1dcb029c},
doi = {10.1016/j.sigpro.2023.109011},
issn = {01651684},
year = {2023},
date = {2023-01-01},
journal = {Signal Processing},
volume = {209},
abstract = {We present a unified statistical model for multivariate and multi-modal texture representation. This model is based on the formalism of finite mixtures of multivariate generalized Gaussians (MoMGG) which enables to build a compact and accurate representation of texture images using multi-resolution texture transforms. The MoMGG model enables to describe the joint statistics of subbands in different scales and orientations, as well as between adjacent locations within the same subband, providing a precise description of the texture layout. It can also combine different multi-scale transforms to build a richer and more representative texture signature for image similarity measurement. We tested our model on both traditional texture transforms (e.g., wavelets, contourlets, maximum response filter) and convolution neural networks (CNNs) features (e.g., ResNet, SqueezeNet). Experiments on color-texture image retrieval have demonstrated the performance of our approach comparatively to state-of-the-art methods. © 2023},
note = {Publisher: Elsevier B.V.},
keywords = {Color texture retrieval, Content-based, Content-based color-texture retrieval, Convolution, convolutional neural network, Gaussians, Image retrieval, Image texture, Mixture of multivariate generalized gaussians, Multi-scale Decomposition, Subbands, Texture representation, Textures},
pubstate = {published},
tppubtype = {article}
}
Laib, L.; Allili, M. S.; Ait-Aoudia, S.
A probabilistic topic model for event-based image classification and multi-label annotation Journal Article
In: Signal Processing: Image Communication, vol. 76, pp. 283–294, 2019, ISSN: 09235965 (ISSN), (Publisher: Elsevier B.V.).
Abstract | Links | BibTeX | Tags: Annotation performance, Classification (of information), Convolution, Convolution neural network, Convolutional neural nets, Event classification, Event recognition, Image annotation, Image Enhancement, Latent Dirichlet allocation, Multi-label annotation, Neural networks, Probabilistic topic models, Semantics, Statistics, Topic Modeling
@article{laib_probabilistic_2019,
title = {A probabilistic topic model for event-based image classification and multi-label annotation},
author = {L. Laib and M. S. Allili and S. Ait-Aoudia},
url = {https://www.scopus.com/inward/record.uri?eid=2-s2.0-85067936924&doi=10.1016%2fj.image.2019.05.012&partnerID=40&md5=a617885b93f3a931c6b6ce1a165f940b},
doi = {10.1016/j.image.2019.05.012},
issn = {09235965 (ISSN)},
year = {2019},
date = {2019-01-01},
journal = {Signal Processing: Image Communication},
volume = {76},
pages = {283–294},
abstract = {We propose an enhanced latent topic model based on latent Dirichlet allocation and convolutional neural nets for event classification and annotation in images. Our model builds on the semantic structure relating events, objects and scenes in images. Based on initial labels extracted from convolution neural networks (CNNs), and possibly user-defined tags, we estimate the event category and final annotation of an image through a refinement process based on the expectation–maximization (EM)algorithm. The EM steps allow to progressively ascertain the class category and refine the final annotation of the image. Our model can be thought of as a two-level annotation system, where the first level derives the image event from CNN labels and image tags and the second level derives the final annotation consisting of event-related objects/scenes. Experimental results show that the proposed model yields better classification and annotation performance in the two standard datasets: UIUC-Sports and WIDER. © 2019 Elsevier B.V.},
note = {Publisher: Elsevier B.V.},
keywords = {Annotation performance, Classification (of information), Convolution, Convolution neural network, Convolutional neural nets, Event classification, Event recognition, Image annotation, Image Enhancement, Latent Dirichlet allocation, Multi-label annotation, Neural networks, Probabilistic topic models, Semantics, Statistics, Topic Modeling},
pubstate = {published},
tppubtype = {article}
}