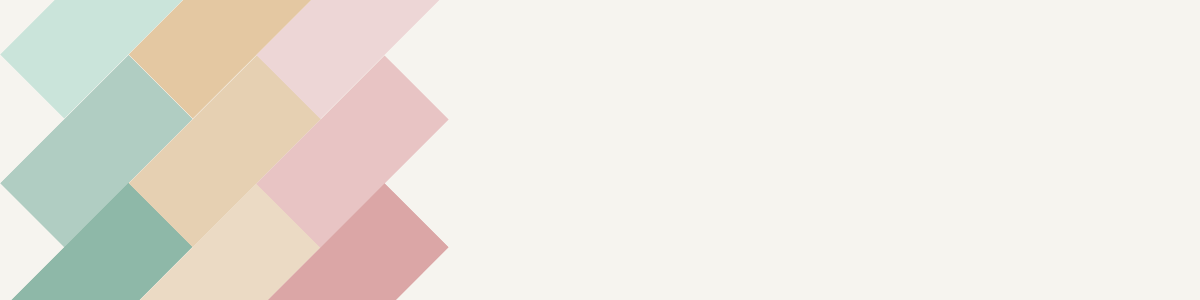
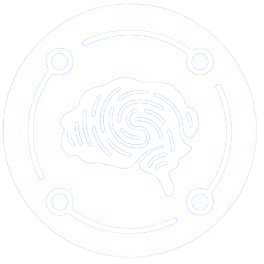
de Recherche et d’Innovation
en Cybersécurité et Société
Joudeh, I. O.; Cretu, A. -M.; Bouchard, S.
Predicting the Arousal and Valence Values of Emotional States Using Learned, Predesigned, and Deep Visual Features † Journal Article
In: Sensors, vol. 24, no. 13, 2024, ISSN: 14248220 (ISSN), (Publisher: Multidisciplinary Digital Publishing Institute (MDPI)).
Abstract | Links | BibTeX | Tags: adult, Affective interaction, Arousal, artificial neural network, Cognitive state, Cognitive/emotional state, Collaborative interaction, computer, Convolutional neural networks, correlation coefficient, Deep learning, emotion, Emotional state, Emotions, female, Forecasting, Helmet mounted displays, human, Humans, Learning algorithms, Learning systems, Long short-term memory, Machine learning, Machine-learning, male, Mean square error, Neural networks, physiology, Regression, Root mean squared errors, Video recording, virtual reality, Visual feature, visual features
@article{joudeh_predicting_2024,
title = {Predicting the Arousal and Valence Values of Emotional States Using Learned, Predesigned, and Deep Visual Features †},
author = {I. O. Joudeh and A. -M. Cretu and S. Bouchard},
url = {https://www.scopus.com/inward/record.uri?eid=2-s2.0-85198382238&doi=10.3390%2fs24134398&partnerID=40&md5=cefa8b2e2c044d02f99662af350007db},
doi = {10.3390/s24134398},
issn = {14248220 (ISSN)},
year = {2024},
date = {2024-01-01},
journal = {Sensors},
volume = {24},
number = {13},
abstract = {The cognitive state of a person can be categorized using the circumplex model of emotional states, a continuous model of two dimensions: arousal and valence. The purpose of this research is to select a machine learning model(s) to be integrated into a virtual reality (VR) system that runs cognitive remediation exercises for people with mental health disorders. As such, the prediction of emotional states is essential to customize treatments for those individuals. We exploit the Remote Collaborative and Affective Interactions (RECOLA) database to predict arousal and valence values using machine learning techniques. RECOLA includes audio, video, and physiological recordings of interactions between human participants. To allow learners to focus on the most relevant data, features are extracted from raw data. Such features can be predesigned, learned, or extracted implicitly using deep learners. Our previous work on video recordings focused on predesigned and learned visual features. In this paper, we extend our work onto deep visual features. Our deep visual features are extracted using the MobileNet-v2 convolutional neural network (CNN) that we previously trained on RECOLA’s video frames of full/half faces. As the final purpose of our work is to integrate our solution into a practical VR application using head-mounted displays, we experimented with half faces as a proof of concept. The extracted deep features were then used to predict arousal and valence values via optimizable ensemble regression. We also fused the extracted visual features with the predesigned visual features and predicted arousal and valence values using the combined feature set. In an attempt to enhance our prediction performance, we further fused the predictions of the optimizable ensemble model with the predictions of the MobileNet-v2 model. After decision fusion, we achieved a root mean squared error (RMSE) of 0.1140, a Pearson’s correlation coefficient (PCC) of 0.8000, and a concordance correlation coefficient (CCC) of 0.7868 on arousal predictions. We achieved an RMSE of 0.0790, a PCC of 0.7904, and a CCC of 0.7645 on valence predictions. © 2024 by the authors.},
note = {Publisher: Multidisciplinary Digital Publishing Institute (MDPI)},
keywords = {adult, Affective interaction, Arousal, artificial neural network, Cognitive state, Cognitive/emotional state, Collaborative interaction, computer, Convolutional neural networks, correlation coefficient, Deep learning, emotion, Emotional state, Emotions, female, Forecasting, Helmet mounted displays, human, Humans, Learning algorithms, Learning systems, Long short-term memory, Machine learning, Machine-learning, male, Mean square error, Neural networks, physiology, Regression, Root mean squared errors, Video recording, virtual reality, Visual feature, visual features},
pubstate = {published},
tppubtype = {article}
}
Laib, L.; Allili, M. S.; Ait-Aoudia, S.
A probabilistic topic model for event-based image classification and multi-label annotation Journal Article
In: Signal Processing: Image Communication, vol. 76, pp. 283–294, 2019, ISSN: 09235965 (ISSN), (Publisher: Elsevier B.V.).
Abstract | Links | BibTeX | Tags: Annotation performance, Classification (of information), Convolution, Convolution neural network, Convolutional neural nets, Event classification, Event recognition, Image annotation, Image Enhancement, Latent Dirichlet allocation, Multi-label annotation, Neural networks, Probabilistic topic models, Semantics, Statistics, Topic Modeling
@article{laib_probabilistic_2019,
title = {A probabilistic topic model for event-based image classification and multi-label annotation},
author = {L. Laib and M. S. Allili and S. Ait-Aoudia},
url = {https://www.scopus.com/inward/record.uri?eid=2-s2.0-85067936924&doi=10.1016%2fj.image.2019.05.012&partnerID=40&md5=a617885b93f3a931c6b6ce1a165f940b},
doi = {10.1016/j.image.2019.05.012},
issn = {09235965 (ISSN)},
year = {2019},
date = {2019-01-01},
journal = {Signal Processing: Image Communication},
volume = {76},
pages = {283–294},
abstract = {We propose an enhanced latent topic model based on latent Dirichlet allocation and convolutional neural nets for event classification and annotation in images. Our model builds on the semantic structure relating events, objects and scenes in images. Based on initial labels extracted from convolution neural networks (CNNs), and possibly user-defined tags, we estimate the event category and final annotation of an image through a refinement process based on the expectation–maximization (EM)algorithm. The EM steps allow to progressively ascertain the class category and refine the final annotation of the image. Our model can be thought of as a two-level annotation system, where the first level derives the image event from CNN labels and image tags and the second level derives the final annotation consisting of event-related objects/scenes. Experimental results show that the proposed model yields better classification and annotation performance in the two standard datasets: UIUC-Sports and WIDER. © 2019 Elsevier B.V.},
note = {Publisher: Elsevier B.V.},
keywords = {Annotation performance, Classification (of information), Convolution, Convolution neural network, Convolutional neural nets, Event classification, Event recognition, Image annotation, Image Enhancement, Latent Dirichlet allocation, Multi-label annotation, Neural networks, Probabilistic topic models, Semantics, Statistics, Topic Modeling},
pubstate = {published},
tppubtype = {article}
}
Ouyed, O.; Allili, M. S.
Feature weighting for multinomial kernel logistic regression and application to action recognition Journal Article
In: Neurocomputing, vol. 275, pp. 1752–1768, 2018, ISSN: 09252312, (Publisher: Elsevier B.V.).
Abstract | Links | BibTeX | Tags: Action recognition, article, Classification, classification algorithm, Classification performance, Computer applications, controlled study, embedding, Feature relevance, feature relevance for multinomial kernel logistic regression, Feature weighting, Kernel logistic regression, kernel method, Learning, mathematical computing, Multinomial kernels, multinominal kernel logistic regression, Neural networks, priority journal, recognition, regression analysis, simulation, sparse modeling, Sparse models, sparse multinomial logistic regression, sparsity promoting regularization, standard, Supervised classification
@article{ouyed_feature_2018,
title = {Feature weighting for multinomial kernel logistic regression and application to action recognition},
author = {O. Ouyed and M. S. Allili},
url = {https://www.scopus.com/inward/record.uri?eid=2-s2.0-85035104467&doi=10.1016%2fj.neucom.2017.10.024&partnerID=40&md5=09687b392a405be4338799a750932cf3},
doi = {10.1016/j.neucom.2017.10.024},
issn = {09252312},
year = {2018},
date = {2018-01-01},
journal = {Neurocomputing},
volume = {275},
pages = {1752–1768},
abstract = {Multinominal kernel logistic regression (MKLR) is a supervised classification method designed for separating classes with non-linear boundaries. However, it relies on the assumption that all features are equally important, which may decrease classification performance when dealing with high-dimensional and noisy data. We propose an approach for embedding feature relevance in multinomial kernel logistic regression. Our approach, coined fr-MKLR, generalizes MKLR by introducing a feature weighting scheme in the Gaussian kernel and using the so-called ℓ0-“norm” as sparsity-promoting regularization. Therefore, the contribution of each feature is tuned according to its relevance for classification which leads to more generalizable and interpretable sparse models for classification. Application of our approach to several standard datasets and video action recognition has provided very promising results compared to other methods. © 2017 Elsevier B.V.},
note = {Publisher: Elsevier B.V.},
keywords = {Action recognition, article, Classification, classification algorithm, Classification performance, Computer applications, controlled study, embedding, Feature relevance, feature relevance for multinomial kernel logistic regression, Feature weighting, Kernel logistic regression, kernel method, Learning, mathematical computing, Multinomial kernels, multinominal kernel logistic regression, Neural networks, priority journal, recognition, regression analysis, simulation, sparse modeling, Sparse models, sparse multinomial logistic regression, sparsity promoting regularization, standard, Supervised classification},
pubstate = {published},
tppubtype = {article}
}
Lahmiri, S.; Gagnon, S.
A sequential probabilistic system for bankruptcy data classification Book
IGI Global, 2018, ISBN: 978-1-5225-5644-2 1-5225-5643-5 978-1-5225-5643-5, (Publication Title: Intelligent Systems: Concepts, Methodologies, Tools, and Applications).
Abstract | Links | BibTeX | Tags: Bankruptcy prediction, Corporate finance, Data classification, Discriminant analysis, Forecasting, Human resource management, Independent variables, Neural networks, Nonlinear problems, Probabilistic systems, Real-world problem, Soft computing, Softcomputing techniques, Support vector machines
@book{lahmiri_sequential_2018,
title = {A sequential probabilistic system for bankruptcy data classification},
author = {S. Lahmiri and S. Gagnon},
url = {https://www.scopus.com/inward/record.uri?eid=2-s2.0-85059712022&doi=10.4018%2f978-1-5225-5643-5.ch064&partnerID=40&md5=21c0d2da6087916a6c372529e2b13784},
doi = {10.4018/978-1-5225-5643-5.ch064},
isbn = {978-1-5225-5644-2 1-5225-5643-5 978-1-5225-5643-5},
year = {2018},
date = {2018-01-01},
publisher = {IGI Global},
abstract = {In the last decade, the development of bankruptcy prediction models has been one of important issues in accounting and corporate finance research fields. Indeed, bankruptcy is a critical event that yields important loss to management, shareholders, employees, and also to government. Statistical methods such as discriminant analysis, logistic and probit models were widely used for developing bankruptcy prediction systems. However, statistical-based approaches are assumes strong assumptions including linearity of the relationship among dependent and independent variables, normality of the errors which limit their applicability in bankruptcy real world problems. Recently, machine learning and soft computing techniques including artificial neural networks, support vector machines, and evolutionary intelligence have brought forth new alternatives in solving nonlinear problems with applications in bankruptcy prediction. The purpose of this chapter is to present a sequential probabilistic system for bankruptcy data classification to help manager in making decisions. © 2018, IGI Global. All rights reserved.},
note = {Publication Title: Intelligent Systems: Concepts, Methodologies, Tools, and Applications},
keywords = {Bankruptcy prediction, Corporate finance, Data classification, Discriminant analysis, Forecasting, Human resource management, Independent variables, Neural networks, Nonlinear problems, Probabilistic systems, Real-world problem, Soft computing, Softcomputing techniques, Support vector machines},
pubstate = {published},
tppubtype = {book}
}
Chartier, S.; Renaud, P.; Boukadoum, M.
A nonlinear dynamic artificial neural network model of memory Journal Article
In: New Ideas in Psychology, vol. 26, no. 2, pp. 252–277, 2008, ISSN: 0732118X (ISSN).
Abstract | Links | BibTeX | Tags: Chaos theory, Cognitive science, Connectionism, Mathematical modeling, Neural networks
@article{chartier_nonlinear_2008,
title = {A nonlinear dynamic artificial neural network model of memory},
author = {S. Chartier and P. Renaud and M. Boukadoum},
url = {https://www.scopus.com/inward/record.uri?eid=2-s2.0-44949197540&doi=10.1016%2fj.newideapsych.2007.07.005&partnerID=40&md5=87bc824ea3c67259a1a36799ea53f0d8},
doi = {10.1016/j.newideapsych.2007.07.005},
issn = {0732118X (ISSN)},
year = {2008},
date = {2008-01-01},
journal = {New Ideas in Psychology},
volume = {26},
number = {2},
pages = {252–277},
abstract = {Nonlinearity and dynamics in psychology are found in various domains such as neuroscience, cognitive science, human development, etc. However, the models that have been proposed are mostly of a computational nature and ignore dynamics. In those models that do include dynamic properties, only fixed points are used to store and retrieve information, leaving many principles of nonlinear dynamic systems (NDS) aside; for instance, chaos is often perceived as a nuisance. This paper considers a nonlinear dynamic artificial neural network (NDANN) that implements NDS principles while also complying with general neuroscience constraints. After a theoretical presentation, simulation results will show that the model can exhibit multi-valued, fixed-point, region-constrained attractors and aperiodic (including chaotic) behaviors. Because the capabilities of NDANN include the modeling of spatiotemporal chaotic activities, it may be an efficient tool to help bridge the gap between biological memory neural models and behavioral memory models. Crown Copyright © 2007.},
keywords = {Chaos theory, Cognitive science, Connectionism, Mathematical modeling, Neural networks},
pubstate = {published},
tppubtype = {article}
}
Chartier, S.; Giguère, G.; Renaud, P.; Lina, J. -M.; Proulx, R.
FEBAM: A feature-extracting bidirectional associative memory Proceedings Article
In: IEEE International Conference on Neural Networks - Conference Proceedings, pp. 1679–1684, Orlando, FL, 2007, ISBN: 1-4244-1380-X 978-1-4244-1380-5, (ISSN: 10987576).
Abstract | Links | BibTeX | Tags: Artificial intelligence, Associative processing, Bi-directional associative memory, Blind source separation, Computer networks, Data storage equipment, Feature extraction, Financial data processing, Hemodynamics, Image processing, Image reconstruction, Independent component analysis, Joint conference, Neural networks, Separation
@inproceedings{chartier_febam_2007,
title = {FEBAM: A feature-extracting bidirectional associative memory},
author = {S. Chartier and G. Giguère and P. Renaud and J. -M. Lina and R. Proulx},
url = {https://www.scopus.com/inward/record.uri?eid=2-s2.0-51749114880&doi=10.1109%2fIJCNN.2007.4371210&partnerID=40&md5=d929ff11969da516dec3fb7a11e742a1},
doi = {10.1109/IJCNN.2007.4371210},
isbn = {1-4244-1380-X 978-1-4244-1380-5},
year = {2007},
date = {2007-01-01},
booktitle = {IEEE International Conference on Neural Networks - Conference Proceedings},
pages = {1679–1684},
address = {Orlando, FL},
abstract = {In this paper, a new model that can ultimately create its own set of perceptual features is proposed. Using a bidirectional associative memory (BAM)-inspired architecture, the resulting model inherits properties such as attractor-like behavior and successful processing of noisy inputs, while being able to achieve principal component analysis (PCA) tasks such as feature extraction and dimensionality reduction. The model is tested by simulating image reconstruction and blind source separation tasks. Simulations show that the model fares particularly well compared to current neural PCA and independent component analysis (ICA) algorithms. It is argued the model possesses more cognitive explanative power than any other nonlinear/linear PCA and ICA algorithm. ©2007 IEEE.},
note = {ISSN: 10987576},
keywords = {Artificial intelligence, Associative processing, Bi-directional associative memory, Blind source separation, Computer networks, Data storage equipment, Feature extraction, Financial data processing, Hemodynamics, Image processing, Image reconstruction, Independent component analysis, Joint conference, Neural networks, Separation},
pubstate = {published},
tppubtype = {inproceedings}
}
Chartier, S.; Renaud, P.
Eye-tracker data filtering using pulse coupled neural network Proceedings Article
In: Proceedings of the IASTED International Conference on Modelling and Simulation, pp. 91–96, Montreal, QC, 2006, ISBN: 0-88986-594-9 978-0-88986-594-5, (ISSN: 10218181).
Abstract | Links | BibTeX | Tags: Data reduction, Eye trackers, Eye-tracker, Filter, Median, Neural networks, Noise, Nonlinear filtering, Pulse couple neural network, Pulse coupled neural network (PCNN), Signal to noise ratio, Spurious signal noise, Wave filters
@inproceedings{chartier_eye-tracker_2006,
title = {Eye-tracker data filtering using pulse coupled neural network},
author = {S. Chartier and P. Renaud},
url = {https://www.scopus.com/inward/record.uri?eid=2-s2.0-33751247311&partnerID=40&md5=ee3f159e47fa32d2710c97826dccdf52},
isbn = {0-88986-594-9 978-0-88986-594-5},
year = {2006},
date = {2006-01-01},
booktitle = {Proceedings of the IASTED International Conference on Modelling and Simulation},
volume = {2006},
pages = {91–96},
address = {Montreal, QC},
abstract = {Data obtained from eye-tracker are contaminated with noise due to eye blink and hardware failure to detect corneal reflection. One solution is to use a nonlinear filter such as the median. However, median filters modify both noisy and noise free data and they are therefore difficult to use in real time applications. To overcome these limits, a simplified pulse coupled neural network (PCNN) is proposed to correctly detect and remove noisy data while leaving uncorrupted data untouched. Results indicated that a filter based on a PCNN achieved a much better performance than the median filter in peak signal-to-noise ratio (PSNR) and in visual inspection.},
note = {ISSN: 10218181},
keywords = {Data reduction, Eye trackers, Eye-tracker, Filter, Median, Neural networks, Noise, Nonlinear filtering, Pulse couple neural network, Pulse coupled neural network (PCNN), Signal to noise ratio, Spurious signal noise, Wave filters},
pubstate = {published},
tppubtype = {inproceedings}
}