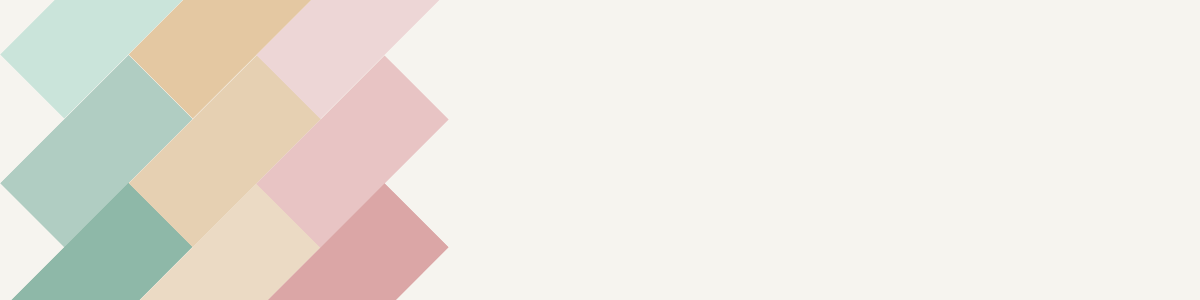
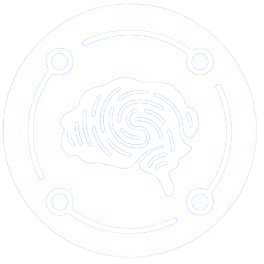
de Recherche et d’Innovation
en Cybersécurité et Société
Joudeh, I. O.; Cretu, A. -M.; Bouchard, S.
Predicting the Arousal and Valence Values of Emotional States Using Learned, Predesigned, and Deep Visual Features † Journal Article
In: Sensors, vol. 24, no. 13, 2024, ISSN: 14248220 (ISSN), (Publisher: Multidisciplinary Digital Publishing Institute (MDPI)).
Abstract | Links | BibTeX | Tags: adult, Affective interaction, Arousal, artificial neural network, Cognitive state, Cognitive/emotional state, Collaborative interaction, computer, Convolutional neural networks, correlation coefficient, Deep learning, emotion, Emotional state, Emotions, female, Forecasting, Helmet mounted displays, human, Humans, Learning algorithms, Learning systems, Long short-term memory, Machine learning, Machine-learning, male, Mean square error, Neural networks, physiology, Regression, Root mean squared errors, Video recording, virtual reality, Visual feature, visual features
@article{joudeh_predicting_2024,
title = {Predicting the Arousal and Valence Values of Emotional States Using Learned, Predesigned, and Deep Visual Features †},
author = {I. O. Joudeh and A. -M. Cretu and S. Bouchard},
url = {https://www.scopus.com/inward/record.uri?eid=2-s2.0-85198382238&doi=10.3390%2fs24134398&partnerID=40&md5=cefa8b2e2c044d02f99662af350007db},
doi = {10.3390/s24134398},
issn = {14248220 (ISSN)},
year = {2024},
date = {2024-01-01},
journal = {Sensors},
volume = {24},
number = {13},
abstract = {The cognitive state of a person can be categorized using the circumplex model of emotional states, a continuous model of two dimensions: arousal and valence. The purpose of this research is to select a machine learning model(s) to be integrated into a virtual reality (VR) system that runs cognitive remediation exercises for people with mental health disorders. As such, the prediction of emotional states is essential to customize treatments for those individuals. We exploit the Remote Collaborative and Affective Interactions (RECOLA) database to predict arousal and valence values using machine learning techniques. RECOLA includes audio, video, and physiological recordings of interactions between human participants. To allow learners to focus on the most relevant data, features are extracted from raw data. Such features can be predesigned, learned, or extracted implicitly using deep learners. Our previous work on video recordings focused on predesigned and learned visual features. In this paper, we extend our work onto deep visual features. Our deep visual features are extracted using the MobileNet-v2 convolutional neural network (CNN) that we previously trained on RECOLA’s video frames of full/half faces. As the final purpose of our work is to integrate our solution into a practical VR application using head-mounted displays, we experimented with half faces as a proof of concept. The extracted deep features were then used to predict arousal and valence values via optimizable ensemble regression. We also fused the extracted visual features with the predesigned visual features and predicted arousal and valence values using the combined feature set. In an attempt to enhance our prediction performance, we further fused the predictions of the optimizable ensemble model with the predictions of the MobileNet-v2 model. After decision fusion, we achieved a root mean squared error (RMSE) of 0.1140, a Pearson’s correlation coefficient (PCC) of 0.8000, and a concordance correlation coefficient (CCC) of 0.7868 on arousal predictions. We achieved an RMSE of 0.0790, a PCC of 0.7904, and a CCC of 0.7645 on valence predictions. © 2024 by the authors.},
note = {Publisher: Multidisciplinary Digital Publishing Institute (MDPI)},
keywords = {adult, Affective interaction, Arousal, artificial neural network, Cognitive state, Cognitive/emotional state, Collaborative interaction, computer, Convolutional neural networks, correlation coefficient, Deep learning, emotion, Emotional state, Emotions, female, Forecasting, Helmet mounted displays, human, Humans, Learning algorithms, Learning systems, Long short-term memory, Machine learning, Machine-learning, male, Mean square error, Neural networks, physiology, Regression, Root mean squared errors, Video recording, virtual reality, Visual feature, visual features},
pubstate = {published},
tppubtype = {article}
}
Yapi, D.; Mejri, M.; Allili, M. S.; Baaziz, N.
A learning-based approach for automatic defect detection in textile images Proceedings Article
In: A., Zaremba M. Sasiadek J. Dolgui (Ed.): IFAC-PapersOnLine, pp. 2423–2428, 2015, ISBN: 24058963 (ISSN), (Issue: 3 Journal Abbreviation: IFAC-PapersOnLine).
Abstract | Links | BibTeX | Tags: Algorithms, Artificial intelligence, Automatic defect detections, Barium compounds, Bayes Classifier, Computational efficiency, Contourlets, Defect detection, Defect detection algorithm, Defects, Detection problems, Feature extraction, Feature extraction and classification, Gaussians, Image classification, Learning algorithms, Learning systems, Learning-based approach, Machine learning approaches, Mixture of generalized gaussians, Mixtures of generalized Gaussians (MoGG), Textile defect detection, Textile images, Textiles, Textures
@inproceedings{yapi_learning-based_2015,
title = {A learning-based approach for automatic defect detection in textile images},
author = {D. Yapi and M. Mejri and M. S. Allili and N. Baaziz},
editor = {Zaremba M. Sasiadek J. Dolgui A.},
url = {https://www.scopus.com/inward/record.uri?eid=2-s2.0-84953865559&doi=10.1016%2fj.ifacol.2015.06.451&partnerID=40&md5=3dd0ef4c27cbd55700f6511af5f46772},
doi = {10.1016/j.ifacol.2015.06.451},
isbn = {24058963 (ISSN)},
year = {2015},
date = {2015-01-01},
booktitle = {IFAC-PapersOnLine},
volume = {28},
pages = {2423–2428},
abstract = {This paper addresses the textile defect detection problem using a machine-learning approach. We propose a novel algorithm that uses supervised learning to classify textile textures in defect and non-defect classes based on suitable feature extraction and classification. We use statistical modeling of multi-scale contourlet image decomposition to obtain compact and accurate signatures for texture description. Our defect detection algorithm is based on two phases. In the first phase, using a training set of images, we extract reference defect-free signatures for each textile category. Then, we use the Bayes classifier (BC) to learn signatures of defected and non-defected classes. In the second phase, defects are detected on new images using the trained BC and an appropriate decomposition of images into blocks. Our algorithm has the capability to achieve highly accurate defect detection and localisation in textile textures while ensuring an efficient computational time. Compared to recent state-of-the-art methods, our algorithm has yielded better results on the standard TILDA database. © 2015, IFAC (International Federation of Automatic Control) Hosting by Elsevier Ltd. All rights reserved.},
note = {Issue: 3
Journal Abbreviation: IFAC-PapersOnLine},
keywords = {Algorithms, Artificial intelligence, Automatic defect detections, Barium compounds, Bayes Classifier, Computational efficiency, Contourlets, Defect detection, Defect detection algorithm, Defects, Detection problems, Feature extraction, Feature extraction and classification, Gaussians, Image classification, Learning algorithms, Learning systems, Learning-based approach, Machine learning approaches, Mixture of generalized gaussians, Mixtures of generalized Gaussians (MoGG), Textile defect detection, Textile images, Textiles, Textures},
pubstate = {published},
tppubtype = {inproceedings}
}