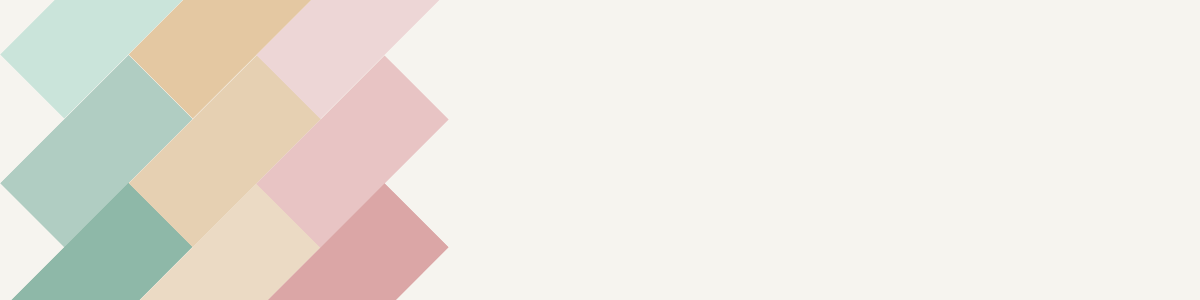
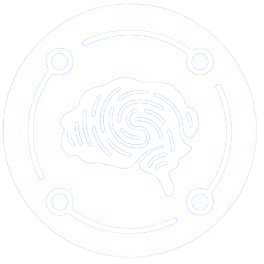
de Recherche et d’Innovation
en Cybersécurité et Société
Yapi, D.; Mejri, M.; Allili, M. S.; Baaziz, N.
A learning-based approach for automatic defect detection in textile images Proceedings Article
In: A., Zaremba M. Sasiadek J. Dolgui (Ed.): IFAC-PapersOnLine, pp. 2423–2428, 2015, ISBN: 24058963 (ISSN), (Issue: 3 Journal Abbreviation: IFAC-PapersOnLine).
Abstract | Links | BibTeX | Tags: Algorithms, Artificial intelligence, Automatic defect detections, Barium compounds, Bayes Classifier, Computational efficiency, Contourlets, Defect detection, Defect detection algorithm, Defects, Detection problems, Feature extraction, Feature extraction and classification, Gaussians, Image classification, Learning algorithms, Learning systems, Learning-based approach, Machine learning approaches, Mixture of generalized gaussians, Mixtures of generalized Gaussians (MoGG), Textile defect detection, Textile images, Textiles, Textures
@inproceedings{yapi_learning-based_2015,
title = {A learning-based approach for automatic defect detection in textile images},
author = {D. Yapi and M. Mejri and M. S. Allili and N. Baaziz},
editor = {Zaremba M. Sasiadek J. Dolgui A.},
url = {https://www.scopus.com/inward/record.uri?eid=2-s2.0-84953865559&doi=10.1016%2fj.ifacol.2015.06.451&partnerID=40&md5=3dd0ef4c27cbd55700f6511af5f46772},
doi = {10.1016/j.ifacol.2015.06.451},
isbn = {24058963 (ISSN)},
year = {2015},
date = {2015-01-01},
booktitle = {IFAC-PapersOnLine},
volume = {28},
pages = {2423–2428},
abstract = {This paper addresses the textile defect detection problem using a machine-learning approach. We propose a novel algorithm that uses supervised learning to classify textile textures in defect and non-defect classes based on suitable feature extraction and classification. We use statistical modeling of multi-scale contourlet image decomposition to obtain compact and accurate signatures for texture description. Our defect detection algorithm is based on two phases. In the first phase, using a training set of images, we extract reference defect-free signatures for each textile category. Then, we use the Bayes classifier (BC) to learn signatures of defected and non-defected classes. In the second phase, defects are detected on new images using the trained BC and an appropriate decomposition of images into blocks. Our algorithm has the capability to achieve highly accurate defect detection and localisation in textile textures while ensuring an efficient computational time. Compared to recent state-of-the-art methods, our algorithm has yielded better results on the standard TILDA database. © 2015, IFAC (International Federation of Automatic Control) Hosting by Elsevier Ltd. All rights reserved.},
note = {Issue: 3
Journal Abbreviation: IFAC-PapersOnLine},
keywords = {Algorithms, Artificial intelligence, Automatic defect detections, Barium compounds, Bayes Classifier, Computational efficiency, Contourlets, Defect detection, Defect detection algorithm, Defects, Detection problems, Feature extraction, Feature extraction and classification, Gaussians, Image classification, Learning algorithms, Learning systems, Learning-based approach, Machine learning approaches, Mixture of generalized gaussians, Mixtures of generalized Gaussians (MoGG), Textile defect detection, Textile images, Textiles, Textures},
pubstate = {published},
tppubtype = {inproceedings}
}
Allili, M. S.; Baaziz, N.
Contourlet-based texture retrieval using a mixture of generalized Gaussian distributions Journal Article
In: Lecture Notes in Computer Science (including subseries Lecture Notes in Artificial Intelligence and Lecture Notes in Bioinformatics), vol. 6855 LNCS, no. PART 2, pp. 446–454, 2011, ISSN: 03029743, (ISBN: 9783642236778 Place: Seville).
Abstract | Links | BibTeX | Tags: Contourlet transform, Contourlets, Distribution modelling, Finite mixtures, Gaussian distribution, Generalized Gaussian Distributions, Image analysis, Kullback-Leibler divergence, Mixtures, Monte-Carlo sampling, Probability density function, Similarity measure, Statistical representations, Texture discrimination, Texture retrieval, Textures
@article{allili_contourlet-based_2011,
title = {Contourlet-based texture retrieval using a mixture of generalized Gaussian distributions},
author = {M. S. Allili and N. Baaziz},
url = {https://www.scopus.com/inward/record.uri?eid=2-s2.0-80052796353&doi=10.1007%2f978-3-642-23678-5_53&partnerID=40&md5=fde8aaeea1609c81747b0ab27a8c78ce},
doi = {10.1007/978-3-642-23678-5_53},
issn = {03029743},
year = {2011},
date = {2011-01-01},
journal = {Lecture Notes in Computer Science (including subseries Lecture Notes in Artificial Intelligence and Lecture Notes in Bioinformatics)},
volume = {6855 LNCS},
number = {PART 2},
pages = {446–454},
abstract = {We address the texture retrieval problem using contourlet-based statistical representation. We propose a new contourlet distribution modelling using finite mixtures of generalized Gaussian distributions (MoGG). The MoGG allows to capture a wide range of contourlet histogram shapes, which provides better description and discrimination of texture than using single probability density functions (pdfs). We propose a model similarity measure based on Kullback-Leibler divergence (KLD) approximation using Monte-Carlo sampling methods. We show that our approach using a redundant contourlet transform yields better texture discrimination and retrieval results than using other methods of statistical-based wavelet/contourlet modelling. © 2011 Springer-Verlag.},
note = {ISBN: 9783642236778
Place: Seville},
keywords = {Contourlet transform, Contourlets, Distribution modelling, Finite mixtures, Gaussian distribution, Generalized Gaussian Distributions, Image analysis, Kullback-Leibler divergence, Mixtures, Monte-Carlo sampling, Probability density function, Similarity measure, Statistical representations, Texture discrimination, Texture retrieval, Textures},
pubstate = {published},
tppubtype = {article}
}