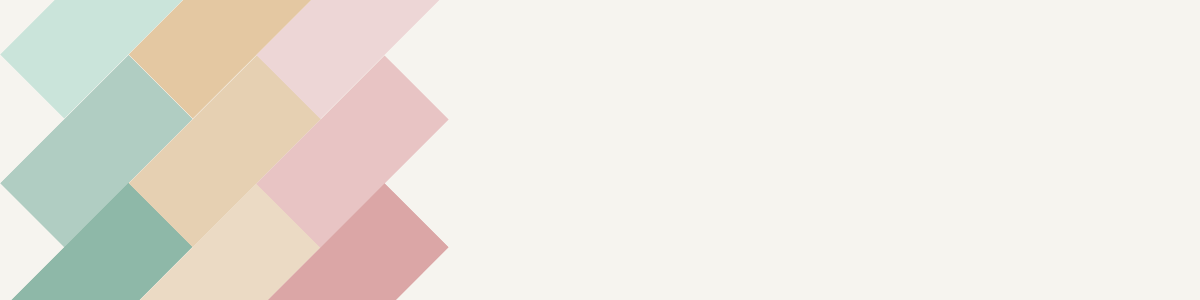
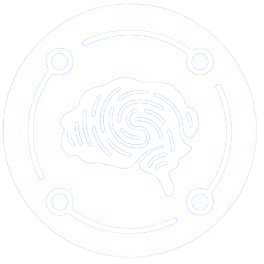
de Recherche et d’Innovation
en Cybersécurité et Société
Allili, M. S.; Baaziz, N.; Mejri, M.
Texture modeling using contourlets and finite mixtures of generalized gaussian distributions and applications Journal Article
In: IEEE Transactions on Multimedia, vol. 16, no. 3, pp. 772–784, 2014, ISSN: 15209210, (Publisher: Institute of Electrical and Electronics Engineers Inc.).
Abstract | Links | BibTeX | Tags: Contourlet coefficients, Contourlet transform, Defects, Directional information, Fabric texture, face recognition, Generalized Gaussian Distributions, Inspection, Mixtures, Probability density function, Probability density functions (PDFs), State-of-the-art methods, Texture retrieval, Textures
@article{allili_texture_2014,
title = {Texture modeling using contourlets and finite mixtures of generalized gaussian distributions and applications},
author = {M. S. Allili and N. Baaziz and M. Mejri},
url = {https://www.scopus.com/inward/record.uri?eid=2-s2.0-84896903467&doi=10.1109%2fTMM.2014.2298832&partnerID=40&md5=16b2fa741e71e1e581f6b0f54c43a676},
doi = {10.1109/TMM.2014.2298832},
issn = {15209210},
year = {2014},
date = {2014-01-01},
journal = {IEEE Transactions on Multimedia},
volume = {16},
number = {3},
pages = {772–784},
abstract = {In this paper, we develop a new framework for contourlet-based statistical modeling using finite Mixtures of Generalized Gaussian distributions (MoGG). On the one hand, given the rich directional information provided by the contourlet transform (CT), we propose to use a redundant version of the CT, which describes texture structures more accurately. On the other hand, we use MoGG modeling of contourlet coefficients distribution, which allows for precise capturing of a wide range of histogram shapes and provides better description and discrimination of texture than single probability density functions (pdfs). Moreover, we propose three applications for the proposed approach, namely: (1) texture retrieval, (2) fabric texture defect detection, and 3) infrared (IR) face recognition. We compare two implementations of the CT: standard CT (SCT) and redundant CT (RCT). We show that the proposed approach yields better results in the applications studied compared to recent state-of-the-art methods. © 2014 IEEE.},
note = {Publisher: Institute of Electrical and Electronics Engineers Inc.},
keywords = {Contourlet coefficients, Contourlet transform, Defects, Directional information, Fabric texture, face recognition, Generalized Gaussian Distributions, Inspection, Mixtures, Probability density function, Probability density functions (PDFs), State-of-the-art methods, Texture retrieval, Textures},
pubstate = {published},
tppubtype = {article}
}
Allili, M. S.; Baaziz, N.
Contourlet-based texture retrieval using a mixture of generalized Gaussian distributions Journal Article
In: Lecture Notes in Computer Science (including subseries Lecture Notes in Artificial Intelligence and Lecture Notes in Bioinformatics), vol. 6855 LNCS, no. PART 2, pp. 446–454, 2011, ISSN: 03029743, (ISBN: 9783642236778 Place: Seville).
Abstract | Links | BibTeX | Tags: Contourlet transform, Contourlets, Distribution modelling, Finite mixtures, Gaussian distribution, Generalized Gaussian Distributions, Image analysis, Kullback-Leibler divergence, Mixtures, Monte-Carlo sampling, Probability density function, Similarity measure, Statistical representations, Texture discrimination, Texture retrieval, Textures
@article{allili_contourlet-based_2011,
title = {Contourlet-based texture retrieval using a mixture of generalized Gaussian distributions},
author = {M. S. Allili and N. Baaziz},
url = {https://www.scopus.com/inward/record.uri?eid=2-s2.0-80052796353&doi=10.1007%2f978-3-642-23678-5_53&partnerID=40&md5=fde8aaeea1609c81747b0ab27a8c78ce},
doi = {10.1007/978-3-642-23678-5_53},
issn = {03029743},
year = {2011},
date = {2011-01-01},
journal = {Lecture Notes in Computer Science (including subseries Lecture Notes in Artificial Intelligence and Lecture Notes in Bioinformatics)},
volume = {6855 LNCS},
number = {PART 2},
pages = {446–454},
abstract = {We address the texture retrieval problem using contourlet-based statistical representation. We propose a new contourlet distribution modelling using finite mixtures of generalized Gaussian distributions (MoGG). The MoGG allows to capture a wide range of contourlet histogram shapes, which provides better description and discrimination of texture than using single probability density functions (pdfs). We propose a model similarity measure based on Kullback-Leibler divergence (KLD) approximation using Monte-Carlo sampling methods. We show that our approach using a redundant contourlet transform yields better texture discrimination and retrieval results than using other methods of statistical-based wavelet/contourlet modelling. © 2011 Springer-Verlag.},
note = {ISBN: 9783642236778
Place: Seville},
keywords = {Contourlet transform, Contourlets, Distribution modelling, Finite mixtures, Gaussian distribution, Generalized Gaussian Distributions, Image analysis, Kullback-Leibler divergence, Mixtures, Monte-Carlo sampling, Probability density function, Similarity measure, Statistical representations, Texture discrimination, Texture retrieval, Textures},
pubstate = {published},
tppubtype = {article}
}
Allili, M. S.
Wavelet-based texture retrieval using a mixture of generalized Gaussian distributions Proceedings Article
In: Proceedings - International Conference on Pattern Recognition, pp. 3143–3146, Istanbul, 2010, ISBN: 978-0-7695-4109-9, (ISSN: 10514651).
Abstract | Links | BibTeX | Tags: Avelet decomposition, Gaussian distribution, Generalized Gaussian Distributions, Image retrieval, KLD, Kullback-Leibler distance, Marginal distribution, Metropolis-Hastings samplings, Mixtures, Pattern Recognition, Probability density function, Probability density function (pdf), Similarity measurements, Statistical methods, Statistical scheme, Texture discrimination, Texture energy, Texture image retrieval, Texture retrieval, Textures, Wavelet coefficients, Wavelet representation
@inproceedings{allili_wavelet-based_2010,
title = {Wavelet-based texture retrieval using a mixture of generalized Gaussian distributions},
author = {M. S. Allili},
url = {https://www.scopus.com/inward/record.uri?eid=2-s2.0-78149489822&doi=10.1109%2fICPR.2010.769&partnerID=40&md5=bf29f6057b57f85a0d83ac16bb4afaf5},
doi = {10.1109/ICPR.2010.769},
isbn = {978-0-7695-4109-9},
year = {2010},
date = {2010-01-01},
booktitle = {Proceedings - International Conference on Pattern Recognition},
pages = {3143–3146},
address = {Istanbul},
abstract = {In this paper, we address the texture retrieval problem using wavelet distribution. We propose a new statistical scheme to represent the marginal distribution of the wavelet coefficients using a mixture of generalized Gaussian distributions (MoGG). The MoGG allows to capture a wide range of histogram shapes, which provides a better description of texture and enhances texture discrimination. We propose a similarity measurement based on Kullback-Leibler distance (KLD), which is calculated using MCMC Metropolis-Hastings sampling algorithm. We show that our approach yields better texture retrieval results than previous methods using only a single probability density function (pdf) for wavelet representation, or texture energy distribution. © 2010 IEEE.},
note = {ISSN: 10514651},
keywords = {Avelet decomposition, Gaussian distribution, Generalized Gaussian Distributions, Image retrieval, KLD, Kullback-Leibler distance, Marginal distribution, Metropolis-Hastings samplings, Mixtures, Pattern Recognition, Probability density function, Probability density function (pdf), Similarity measurements, Statistical methods, Statistical scheme, Texture discrimination, Texture energy, Texture image retrieval, Texture retrieval, Textures, Wavelet coefficients, Wavelet representation},
pubstate = {published},
tppubtype = {inproceedings}
}
Allili, M. S.; Ziou, D.
Automatic change detection and updating of topographic databases by using satellite imagery: A level set approach Journal Article
In: Geomatica, vol. 59, no. 3, pp. 275–281, 2005, ISSN: 11951036 (ISSN).
Abstract | Links | BibTeX | Tags: Adaptive control systems, Adaptive segmentation, Color image processing, Computer Simulation, Image analysis, Image segmentation, Level sets, Mixture analysis, Polarity smoothing, Probability density function
@article{allili_automatic_2005,
title = {Automatic change detection and updating of topographic databases by using satellite imagery: A level set approach},
author = {M. S. Allili and D. Ziou},
url = {https://www.scopus.com/inward/record.uri?eid=2-s2.0-28444481044&partnerID=40&md5=e5d147401a402b14084292a2eeb6a792},
doi = {10.1109/CRV.2005.14},
issn = {11951036 (ISSN)},
year = {2005},
date = {2005-01-01},
booktitle = {Proceedings - 2nd Canadian Conference on Computer and Robot Vision, CRV 2005},
journal = {Geomatica},
volume = {59},
number = {3},
pages = {275–281},
publisher = {Institute of Electrical and Electronics Engineers Inc.},
abstract = {In order to keep up-to-date geospatial data in topographic databases, automatic change detection and data updating is required. In the present paper, we investigate the automatic change detection of geospatial data by using level set active contours. We propose an approach that is based on region comparison between two multi-temporal datasets. Firstly, the regions are extracted from two co-registered images taken apart in time by using level set based active contours segmentation. Then, the change detection is performed by spatially comparing the resulting region segments from the two images. The approach is validated by experiments relating to the change detection of lake surfaces by using Landsat7 multi-spectral imagery.},
keywords = {Adaptive control systems, Adaptive segmentation, Color image processing, Computer Simulation, Image analysis, Image segmentation, Level sets, Mixture analysis, Polarity smoothing, Probability density function},
pubstate = {published},
tppubtype = {article}
}