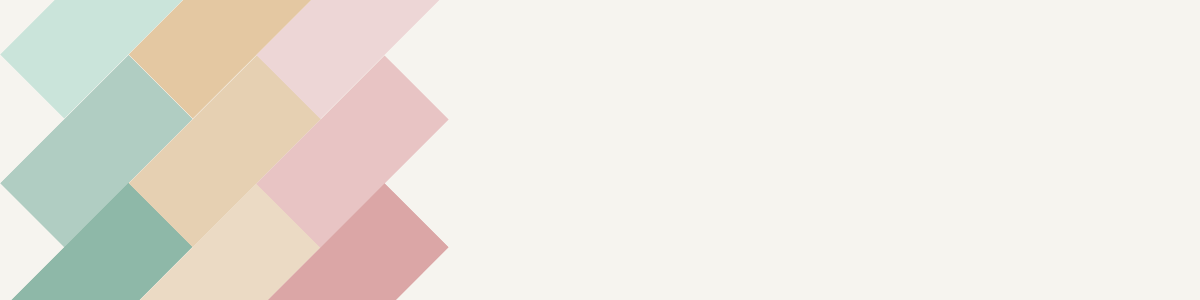
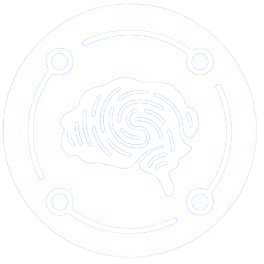
de Recherche et d’Innovation
en Cybersécurité et Société
Yapi, D.; Allili, M. S.
Multi-Band Texture Modeling Using Finite Mixtures of Multivariate Generalized Gaussian Distributions Proceedings Article
In: Proceedings - International Conference on Pattern Recognition, pp. 464–469, Institute of Electrical and Electronics Engineers Inc., 2022, ISBN: 978-1-66549-062-7, (ISSN: 10514651).
Abstract | Links | BibTeX | Tags: Color texture retrieval, Finite mixtures, Gaussian distribution, Gaussians, Image retrieval, Image texture, Mixture of multivariate generalized gaussians, Multi band, Multi-scale Decomposition, Multivariate generalized gaussian distributions, Statistic modeling, Subbands, Texture models, Textures
@inproceedings{yapi_multi-band_2022,
title = {Multi-Band Texture Modeling Using Finite Mixtures of Multivariate Generalized Gaussian Distributions},
author = {D. Yapi and M. S. Allili},
url = {https://www.scopus.com/inward/record.uri?eid=2-s2.0-85143638804&doi=10.1109%2fICPR56361.2022.9956448&partnerID=40&md5=3fe34da98f314b85014059630e4c8c6c},
doi = {10.1109/ICPR56361.2022.9956448},
isbn = {978-1-66549-062-7},
year = {2022},
date = {2022-01-01},
booktitle = {Proceedings - International Conference on Pattern Recognition},
volume = {2022-August},
pages = {464–469},
publisher = {Institute of Electrical and Electronics Engineers Inc.},
abstract = {We present a unified statistical model for multivariate and multi-modal texture representation. This model is based on the formalism of finite mixtures of multivariate generalized Gaussians (MoMGG) which enables a compact and accurate representation of joint statistics of different sub-bands of multireslotion texture transform. This representation expresses correlation between sub-bands at different scales and orientations, and also between adjacent locations within the same subbands, providing a precise description of the texture layout. It enables also to combine different multi-scale transforms to build a richer and more representative texture signature. We successfully tested the model on traditional texture transforms such as wavelets and contourlets. Experiments on color-texture image retrieval have demonstrated the performance of our approach comparatively to state-of-art methods. © 2022 IEEE.},
note = {ISSN: 10514651},
keywords = {Color texture retrieval, Finite mixtures, Gaussian distribution, Gaussians, Image retrieval, Image texture, Mixture of multivariate generalized gaussians, Multi band, Multi-scale Decomposition, Multivariate generalized gaussian distributions, Statistic modeling, Subbands, Texture models, Textures},
pubstate = {published},
tppubtype = {inproceedings}
}
Allili, M. S.
Wavelet modeling using finite mixtures of generalized Gaussian distributions: Application to texture discrimination and retrieval Journal Article
In: IEEE Transactions on Image Processing, vol. 21, no. 4, pp. 1452–1464, 2012, ISSN: 10577149.
Abstract | Links | BibTeX | Tags: algorithm, Algorithms, article, Automated, automated pattern recognition, computer assisted diagnosis, Computer Simulation, Computer-Assisted, Data Interpretation, Finite mixtures, Generalized Gaussian, Generalized Gaussian Distributions, Image Enhancement, Image Interpretation, Image segmentation, Imaging, Kullback Leibler divergence, Marginal distribution, methodology, Mixtures, Models, Monte Carlo methods, Monte Carlo sampling, Normal Distribution, Pattern Recognition, Performance improvements, reproducibility, Reproducibility of Results, Sensitivity and Specificity, Similarity measure, State-of-the-art approach, Statistical, statistical analysis, statistical model, Texture data set, Texture discrimination, Texture modeling, Textures, three dimensional imaging, Three-Dimensional, Wavelet Analysis, Wavelet coefficients, Wavelet decomposition, Wavelet modeling
@article{allili_wavelet_2012,
title = {Wavelet modeling using finite mixtures of generalized Gaussian distributions: Application to texture discrimination and retrieval},
author = {M. S. Allili},
url = {https://www.scopus.com/inward/record.uri?eid=2-s2.0-84859096106&doi=10.1109%2fTIP.2011.2170701&partnerID=40&md5=0420facdc04978ad84bea3126bc1183a},
doi = {10.1109/TIP.2011.2170701},
issn = {10577149},
year = {2012},
date = {2012-01-01},
journal = {IEEE Transactions on Image Processing},
volume = {21},
number = {4},
pages = {1452–1464},
abstract = {This paper addresses statistical-based texture modeling using wavelets. We propose a new approach to represent the marginal distribution of the wavelet coefficients using finite mixtures of generalized Gaussian (MoGG) distributions. The MoGG captures a wide range of histogram shapes, which provides better description and discrimination of texture than using single probability density functions (pdf's), as proposed by recent state-of-the-art approaches. Moreover, we propose a model similarity measure based on Kullback-Leibler divergence (KLD) approximation using Monte Carlo sampling methods. Through experiments on two popular texture data sets, we show that our approach yields significant performance improvements for texture discrimination and retrieval, as compared with recent methods of statistical-based wavelet modeling. © 2011 IEEE.},
keywords = {algorithm, Algorithms, article, Automated, automated pattern recognition, computer assisted diagnosis, Computer Simulation, Computer-Assisted, Data Interpretation, Finite mixtures, Generalized Gaussian, Generalized Gaussian Distributions, Image Enhancement, Image Interpretation, Image segmentation, Imaging, Kullback Leibler divergence, Marginal distribution, methodology, Mixtures, Models, Monte Carlo methods, Monte Carlo sampling, Normal Distribution, Pattern Recognition, Performance improvements, reproducibility, Reproducibility of Results, Sensitivity and Specificity, Similarity measure, State-of-the-art approach, Statistical, statistical analysis, statistical model, Texture data set, Texture discrimination, Texture modeling, Textures, three dimensional imaging, Three-Dimensional, Wavelet Analysis, Wavelet coefficients, Wavelet decomposition, Wavelet modeling},
pubstate = {published},
tppubtype = {article}
}
Allili, M. S.; Baaziz, N.
Contourlet-based texture retrieval using a mixture of generalized Gaussian distributions Journal Article
In: Lecture Notes in Computer Science (including subseries Lecture Notes in Artificial Intelligence and Lecture Notes in Bioinformatics), vol. 6855 LNCS, no. PART 2, pp. 446–454, 2011, ISSN: 03029743, (ISBN: 9783642236778 Place: Seville).
Abstract | Links | BibTeX | Tags: Contourlet transform, Contourlets, Distribution modelling, Finite mixtures, Gaussian distribution, Generalized Gaussian Distributions, Image analysis, Kullback-Leibler divergence, Mixtures, Monte-Carlo sampling, Probability density function, Similarity measure, Statistical representations, Texture discrimination, Texture retrieval, Textures
@article{allili_contourlet-based_2011,
title = {Contourlet-based texture retrieval using a mixture of generalized Gaussian distributions},
author = {M. S. Allili and N. Baaziz},
url = {https://www.scopus.com/inward/record.uri?eid=2-s2.0-80052796353&doi=10.1007%2f978-3-642-23678-5_53&partnerID=40&md5=fde8aaeea1609c81747b0ab27a8c78ce},
doi = {10.1007/978-3-642-23678-5_53},
issn = {03029743},
year = {2011},
date = {2011-01-01},
journal = {Lecture Notes in Computer Science (including subseries Lecture Notes in Artificial Intelligence and Lecture Notes in Bioinformatics)},
volume = {6855 LNCS},
number = {PART 2},
pages = {446–454},
abstract = {We address the texture retrieval problem using contourlet-based statistical representation. We propose a new contourlet distribution modelling using finite mixtures of generalized Gaussian distributions (MoGG). The MoGG allows to capture a wide range of contourlet histogram shapes, which provides better description and discrimination of texture than using single probability density functions (pdfs). We propose a model similarity measure based on Kullback-Leibler divergence (KLD) approximation using Monte-Carlo sampling methods. We show that our approach using a redundant contourlet transform yields better texture discrimination and retrieval results than using other methods of statistical-based wavelet/contourlet modelling. © 2011 Springer-Verlag.},
note = {ISBN: 9783642236778
Place: Seville},
keywords = {Contourlet transform, Contourlets, Distribution modelling, Finite mixtures, Gaussian distribution, Generalized Gaussian Distributions, Image analysis, Kullback-Leibler divergence, Mixtures, Monte-Carlo sampling, Probability density function, Similarity measure, Statistical representations, Texture discrimination, Texture retrieval, Textures},
pubstate = {published},
tppubtype = {article}
}
Ziou, D.; Bouguila, N.; Allili, M. S.; El-Zaart, A.
Finite Gamma mixture modelling using minimum message length inference: Application to SAR image analysis Journal Article
In: International Journal of Remote Sensing, vol. 30, no. 3, pp. 771–792, 2009, ISSN: 01431161, (Publisher: Taylor and Francis Ltd.).
Abstract | Links | BibTeX | Tags: Change detection, Determining the number of clusters, estimation method, finite element method, Finite mixtures, Gamma distribution, Gamma mixtures, Image analysis, Image processing, Image segmentation, Minimum message lengths, Mixtures, Number of clusters, numerical model, Probability distributions, Radar imaging, SAR image segmentation, Synthetic aperture radar, Unsupervised learning
@article{ziou_finite_2009,
title = {Finite Gamma mixture modelling using minimum message length inference: Application to SAR image analysis},
author = {D. Ziou and N. Bouguila and M. S. Allili and A. El-Zaart},
url = {https://www.scopus.com/inward/record.uri?eid=2-s2.0-67650686123&doi=10.1080%2f01431160802392646&partnerID=40&md5=901ea39ad806dcb62cd630585469af60},
doi = {10.1080/01431160802392646},
issn = {01431161},
year = {2009},
date = {2009-01-01},
journal = {International Journal of Remote Sensing},
volume = {30},
number = {3},
pages = {771–792},
abstract = {This paper discusses the unsupervised learning problem for finite mixtures of Gamma distributions. An important part of this problem is determining the number of clusters which best describes a set of data. We apply the Minimum Message Length (MML) criterion to the unsupervised learning problem in the case of finite mixtures of Gamma distributions. The MML and other criteria in the literature are compared in terms of their ability to estimate the number of clusters in a data set. The comparison utilizes synthetic and RADARSAT SAR images. The performance of our method is also tested by contextual evaluations involving SAR image segmentation and change detection.},
note = {Publisher: Taylor and Francis Ltd.},
keywords = {Change detection, Determining the number of clusters, estimation method, finite element method, Finite mixtures, Gamma distribution, Gamma mixtures, Image analysis, Image processing, Image segmentation, Minimum message lengths, Mixtures, Number of clusters, numerical model, Probability distributions, Radar imaging, SAR image segmentation, Synthetic aperture radar, Unsupervised learning},
pubstate = {published},
tppubtype = {article}
}