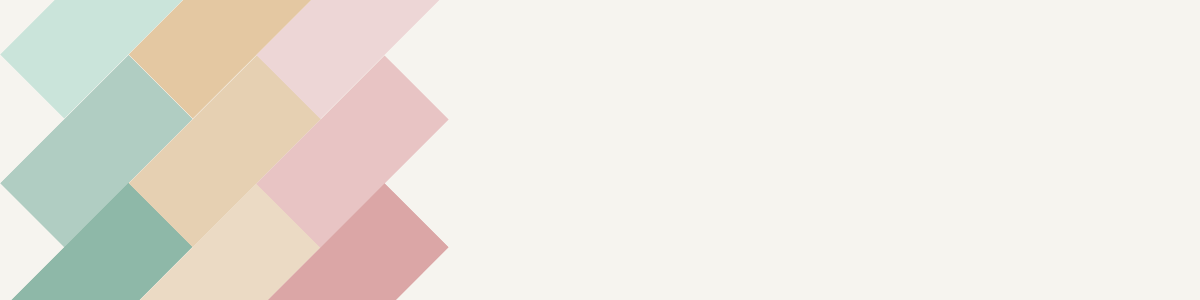
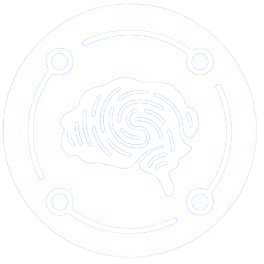
de Recherche et d’Innovation
en Cybersécurité et Société
Canale, N.; Cornil, A.; Giroux, I.; Bouchard, S.; Billieux, J.
Probing gambling urge as a state construct: Evidence from a sample of community gamblers Journal Article
In: Psychology of Addictive Behaviors, vol. 33, no. 2, pp. 154–161, 2019, ISSN: 0893164X, (Publisher: Educational Publishing Foundation).
Abstract | Links | BibTeX | Tags: Adolescent, adult, aged, anticipation, article, case report, clinical article, clinical practice, confirmatory factor analysis, craving, Cross-Sectional Studies, cross-sectional study, devices, Diagnosis, exploratory factor analysis, Factor Analysis, female, Gambling, guided imagery, human, Humans, impulsiveness, male, Middle Aged, procedures, Psychiatric Status Rating Scales, psychological rating scale, Psychometrics, psychometry, reliability, reproducibility, Reproducibility of Results, Statistical, Young Adult
@article{canale_probing_2019,
title = {Probing gambling urge as a state construct: Evidence from a sample of community gamblers},
author = {N. Canale and A. Cornil and I. Giroux and S. Bouchard and J. Billieux},
url = {https://www.scopus.com/inward/record.uri?eid=2-s2.0-85059626617&doi=10.1037%2fadb0000438&partnerID=40&md5=7d3a08b0815d09db467360f3a385f57d},
doi = {10.1037/adb0000438},
issn = {0893164X},
year = {2019},
date = {2019-01-01},
journal = {Psychology of Addictive Behaviors},
volume = {33},
number = {2},
pages = {154–161},
abstract = {Little effort has been made to systematically test the psychometric properties of the Gambling Craving Scale (GACS; Young & Wohl, 2009). The GACS is adapted from the Questionnaire on Smoking Urges (Tiffany & Drobes, 1991) and thus measures gambling-related urge. Crucially, the validation of scales assessing gambling urge is complex because this construct is better conceptualized as a state (a transient and contextdetermined phenomenon). In the present study, we tested the psychometric properties of the French version of the GACS with 2 independent samples of community gamblers following an induction procedure delivered through an audio-guided imagery sequence aimed at promoting gambling urge. This procedure was specifically used to ensure the assessment of gambling urge as a state variable. Participants also completed measures of gambling severity, gambling cognitions and motives, impulsivity, and affect. Confirmatory factor analysis showed that the original 3-factor solution (anticipation, desire, relief) did not fit the data well. Additional exploratory factor analysis suggested instead a 2-factor solution: An intention and desire to gamble dimension and a relief dimension. The factorial structure resulting from the exploratory factor analysis was tested with confirmatory factor analysis in a second independent sample, resulting in an acceptable fit. The 2 dimensions presented good internal reliability and correlated differentially with the other study's variables. The current study showed that, similar to what has been reported for substance-related urges, gambling urges are adequately probed with a bidimensional model. The findings suggest that the French GACS has good psychometric properties, legitimizing its use in research and clinical practice. © 2019 American Psychological Association.},
note = {Publisher: Educational Publishing Foundation},
keywords = {Adolescent, adult, aged, anticipation, article, case report, clinical article, clinical practice, confirmatory factor analysis, craving, Cross-Sectional Studies, cross-sectional study, devices, Diagnosis, exploratory factor analysis, Factor Analysis, female, Gambling, guided imagery, human, Humans, impulsiveness, male, Middle Aged, procedures, Psychiatric Status Rating Scales, psychological rating scale, Psychometrics, psychometry, reliability, reproducibility, Reproducibility of Results, Statistical, Young Adult},
pubstate = {published},
tppubtype = {article}
}
Allili, M. S.
Wavelet modeling using finite mixtures of generalized Gaussian distributions: Application to texture discrimination and retrieval Journal Article
In: IEEE Transactions on Image Processing, vol. 21, no. 4, pp. 1452–1464, 2012, ISSN: 10577149.
Abstract | Links | BibTeX | Tags: algorithm, Algorithms, article, Automated, automated pattern recognition, computer assisted diagnosis, Computer Simulation, Computer-Assisted, Data Interpretation, Finite mixtures, Generalized Gaussian, Generalized Gaussian Distributions, Image Enhancement, Image Interpretation, Image segmentation, Imaging, Kullback Leibler divergence, Marginal distribution, methodology, Mixtures, Models, Monte Carlo methods, Monte Carlo sampling, Normal Distribution, Pattern Recognition, Performance improvements, reproducibility, Reproducibility of Results, Sensitivity and Specificity, Similarity measure, State-of-the-art approach, Statistical, statistical analysis, statistical model, Texture data set, Texture discrimination, Texture modeling, Textures, three dimensional imaging, Three-Dimensional, Wavelet Analysis, Wavelet coefficients, Wavelet decomposition, Wavelet modeling
@article{allili_wavelet_2012,
title = {Wavelet modeling using finite mixtures of generalized Gaussian distributions: Application to texture discrimination and retrieval},
author = {M. S. Allili},
url = {https://www.scopus.com/inward/record.uri?eid=2-s2.0-84859096106&doi=10.1109%2fTIP.2011.2170701&partnerID=40&md5=0420facdc04978ad84bea3126bc1183a},
doi = {10.1109/TIP.2011.2170701},
issn = {10577149},
year = {2012},
date = {2012-01-01},
journal = {IEEE Transactions on Image Processing},
volume = {21},
number = {4},
pages = {1452–1464},
abstract = {This paper addresses statistical-based texture modeling using wavelets. We propose a new approach to represent the marginal distribution of the wavelet coefficients using finite mixtures of generalized Gaussian (MoGG) distributions. The MoGG captures a wide range of histogram shapes, which provides better description and discrimination of texture than using single probability density functions (pdf's), as proposed by recent state-of-the-art approaches. Moreover, we propose a model similarity measure based on Kullback-Leibler divergence (KLD) approximation using Monte Carlo sampling methods. Through experiments on two popular texture data sets, we show that our approach yields significant performance improvements for texture discrimination and retrieval, as compared with recent methods of statistical-based wavelet modeling. © 2011 IEEE.},
keywords = {algorithm, Algorithms, article, Automated, automated pattern recognition, computer assisted diagnosis, Computer Simulation, Computer-Assisted, Data Interpretation, Finite mixtures, Generalized Gaussian, Generalized Gaussian Distributions, Image Enhancement, Image Interpretation, Image segmentation, Imaging, Kullback Leibler divergence, Marginal distribution, methodology, Mixtures, Models, Monte Carlo methods, Monte Carlo sampling, Normal Distribution, Pattern Recognition, Performance improvements, reproducibility, Reproducibility of Results, Sensitivity and Specificity, Similarity measure, State-of-the-art approach, Statistical, statistical analysis, statistical model, Texture data set, Texture discrimination, Texture modeling, Textures, three dimensional imaging, Three-Dimensional, Wavelet Analysis, Wavelet coefficients, Wavelet decomposition, Wavelet modeling},
pubstate = {published},
tppubtype = {article}
}