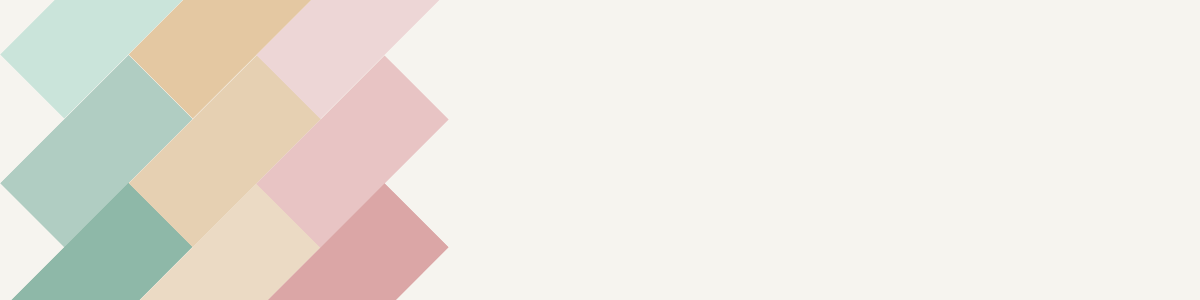
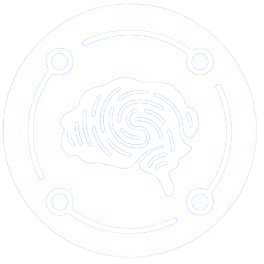
de Recherche et d’Innovation
en Cybersécurité et Société
Ouyed, O.; Allili, M. S.
Feature weighting for multinomial kernel logistic regression and application to action recognition Journal Article
In: Neurocomputing, vol. 275, pp. 1752–1768, 2018, ISSN: 09252312, (Publisher: Elsevier B.V.).
Abstract | Links | BibTeX | Tags: Action recognition, article, Classification, classification algorithm, Classification performance, Computer applications, controlled study, embedding, Feature relevance, feature relevance for multinomial kernel logistic regression, Feature weighting, Kernel logistic regression, kernel method, Learning, mathematical computing, Multinomial kernels, multinominal kernel logistic regression, Neural networks, priority journal, recognition, regression analysis, simulation, sparse modeling, Sparse models, sparse multinomial logistic regression, sparsity promoting regularization, standard, Supervised classification
@article{ouyed_feature_2018,
title = {Feature weighting for multinomial kernel logistic regression and application to action recognition},
author = {O. Ouyed and M. S. Allili},
url = {https://www.scopus.com/inward/record.uri?eid=2-s2.0-85035104467&doi=10.1016%2fj.neucom.2017.10.024&partnerID=40&md5=09687b392a405be4338799a750932cf3},
doi = {10.1016/j.neucom.2017.10.024},
issn = {09252312},
year = {2018},
date = {2018-01-01},
journal = {Neurocomputing},
volume = {275},
pages = {1752–1768},
abstract = {Multinominal kernel logistic regression (MKLR) is a supervised classification method designed for separating classes with non-linear boundaries. However, it relies on the assumption that all features are equally important, which may decrease classification performance when dealing with high-dimensional and noisy data. We propose an approach for embedding feature relevance in multinomial kernel logistic regression. Our approach, coined fr-MKLR, generalizes MKLR by introducing a feature weighting scheme in the Gaussian kernel and using the so-called ℓ0-“norm” as sparsity-promoting regularization. Therefore, the contribution of each feature is tuned according to its relevance for classification which leads to more generalizable and interpretable sparse models for classification. Application of our approach to several standard datasets and video action recognition has provided very promising results compared to other methods. © 2017 Elsevier B.V.},
note = {Publisher: Elsevier B.V.},
keywords = {Action recognition, article, Classification, classification algorithm, Classification performance, Computer applications, controlled study, embedding, Feature relevance, feature relevance for multinomial kernel logistic regression, Feature weighting, Kernel logistic regression, kernel method, Learning, mathematical computing, Multinomial kernels, multinominal kernel logistic regression, Neural networks, priority journal, recognition, regression analysis, simulation, sparse modeling, Sparse models, sparse multinomial logistic regression, sparsity promoting regularization, standard, Supervised classification},
pubstate = {published},
tppubtype = {article}
}
Allili, M. S.
Object contour tracking using foreground and background distribution matching Journal Article
In: Lecture Notes in Computer Science (including subseries Lecture Notes in Artificial Intelligence and Lecture Notes in Bioinformatics), vol. 5856 LNCS, pp. 954–961, 2009, ISSN: 03029743, (ISBN: 3642102670; 9783642102677 Place: Guadalajara, Jalisco).
Abstract | Links | BibTeX | Tags: Active contours, Computer applications, Computer vision, Distribution matching, Distribution parameters, Image matching, Object contour, Tracked objects
@article{allili_object_2009,
title = {Object contour tracking using foreground and background distribution matching},
author = {M. S. Allili},
url = {https://www.scopus.com/inward/record.uri?eid=2-s2.0-78651256419&doi=10.1007%2f978-3-642-10268-4_111&partnerID=40&md5=0852d2cf799d98cff187d1b10b2e5c34},
doi = {10.1007/978-3-642-10268-4_111},
issn = {03029743},
year = {2009},
date = {2009-01-01},
journal = {Lecture Notes in Computer Science (including subseries Lecture Notes in Artificial Intelligence and Lecture Notes in Bioinformatics)},
volume = {5856 LNCS},
pages = {954–961},
abstract = {In this paper, we propose an effective approach for tracking distribution of objects. The approach uses a competition between a tracked object and background distributions using active contours. Only the segmentation of the object in the first frame is required for initialization. The object contour is tracked by assigning pixels in a way that maximizes the likelihood of the object versus the background. We implement the approach using an EM-like algorithm which evolves the object contour exactly to its boundaries and adapts the distribution parameters of the object and the background to data. © 2009 Springer-Verlag Berlin Heidelberg.},
note = {ISBN: 3642102670; 9783642102677
Place: Guadalajara, Jalisco},
keywords = {Active contours, Computer applications, Computer vision, Distribution matching, Distribution parameters, Image matching, Object contour, Tracked objects},
pubstate = {published},
tppubtype = {article}
}