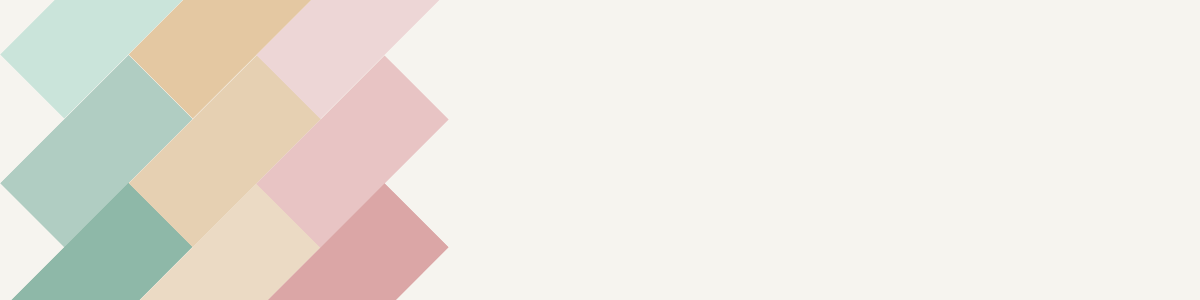
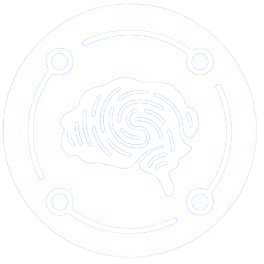
de Recherche et d’Innovation
en Cybersécurité et Société
Boulmerka, A.; Allili, M. S.
Thresholding-based segmentation revisited using mixtures of generalized Gaussian distributions Proceedings Article
In: Proceedings - International Conference on Pattern Recognition, pp. 2894–2897, Tsukuba, 2012, ISBN: 978-4-9906441-0-9, (ISSN: 10514651).
Abstract | Links | BibTeX | Tags: Arbitrary number, Gaussian noise (electronic), Generalized Gaussian Distributions, Heavy-tailed, Image segmentation, Kittler, Minimum error thresholding, Multi-modal, New approaches, Non-Gaussian, Otsu's method, Pattern Recognition, State-of-the-art techniques, Synthetic data
@inproceedings{boulmerka_thresholding-based_2012,
title = {Thresholding-based segmentation revisited using mixtures of generalized Gaussian distributions},
author = {A. Boulmerka and M. S. Allili},
url = {https://www.scopus.com/inward/record.uri?eid=2-s2.0-84874575463&partnerID=40&md5=0665cce9aa19af524d1213c1ff728d94},
isbn = {978-4-9906441-0-9},
year = {2012},
date = {2012-01-01},
booktitle = {Proceedings - International Conference on Pattern Recognition},
pages = {2894–2897},
address = {Tsukuba},
abstract = {This paper presents a new approach to image-thresholding-based segmentation. It considerably improves existing methods by efficiently modeling non-Gaussian and multi-modal class-conditional distributions. The proposed approach seamlessly: 1) extends the Otsu's method to arbitrary numbers of thresholds and 2) extends the Kittler and Illingworth minimum error thresholding to non-Gaussian and multi-modal class-conditional data. We use the recently-proposed mixture of generalized Gaussian distributions (MoGG) modeling, which enables to efficiently represent heavy-tailed data, as well as multi-modal histograms with flat and sharply-shaped peaks. Experiments performed on synthetic data and real-world image segmentation show the performance of the proposed approach with comparison to recent state-of-the-art techniques. © 2012 ICPR Org Committee.},
note = {ISSN: 10514651},
keywords = {Arbitrary number, Gaussian noise (electronic), Generalized Gaussian Distributions, Heavy-tailed, Image segmentation, Kittler, Minimum error thresholding, Multi-modal, New approaches, Non-Gaussian, Otsu's method, Pattern Recognition, State-of-the-art techniques, Synthetic data},
pubstate = {published},
tppubtype = {inproceedings}
}
Allili, M. S.; Ziou, D.
An approach for dynamic combination of region and boundary information in segmentation Proceedings Article
In: Proceedings - International Conference on Pattern Recognition, Institute of Electrical and Electronics Engineers Inc., 2008, ISBN: 10514651 (ISSN); 978-142442175-6 (ISBN), (Journal Abbreviation: Proc. Int. Conf. Pattern Recognit.).
Abstract | Links | BibTeX | Tags: Arbitrary weighting, Bayesian formulation, Bayesian networks, Boundary information, Dynamic combination, Energy functionals, Hyper-parameter, Image segmentation, New approaches, Parameter estimation, Pattern Recognition, Region information
@inproceedings{allili_approach_2008,
title = {An approach for dynamic combination of region and boundary information in segmentation},
author = {M. S. Allili and D. Ziou},
url = {https://www.scopus.com/inward/record.uri?eid=2-s2.0-77957954657&doi=10.1109%2ficpr.2008.4761384&partnerID=40&md5=92c6dc032da4938d5c1c3ced6af1671c},
doi = {10.1109/icpr.2008.4761384},
isbn = {10514651 (ISSN); 978-142442175-6 (ISBN)},
year = {2008},
date = {2008-01-01},
booktitle = {Proceedings - International Conference on Pattern Recognition},
publisher = {Institute of Electrical and Electronics Engineers Inc.},
abstract = {Image segmentation combining boundary and region information has been the subject of numerous research works in the past. This combination is usually subject to arbitrary weighting parameters (hyper-parameters) that control the contribution of boundary and region features during segmentation. In this work, we investigate a new approach for estimating the hyper-parameters adaptively to segmentation. The approach takes its roots from the physical properties of the energy functional controlling segmentation and a Bayesian formulation of segmentation and hyper-parameters estimation. © 2008 IEEE.},
note = {Journal Abbreviation: Proc. Int. Conf. Pattern Recognit.},
keywords = {Arbitrary weighting, Bayesian formulation, Bayesian networks, Boundary information, Dynamic combination, Energy functionals, Hyper-parameter, Image segmentation, New approaches, Parameter estimation, Pattern Recognition, Region information},
pubstate = {published},
tppubtype = {inproceedings}
}