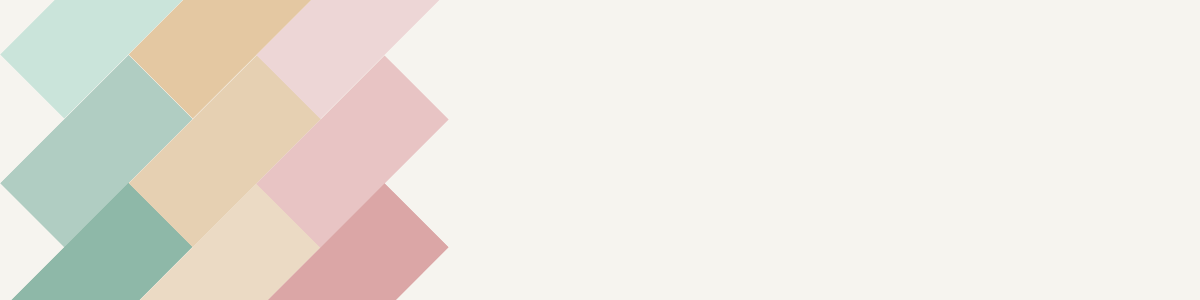
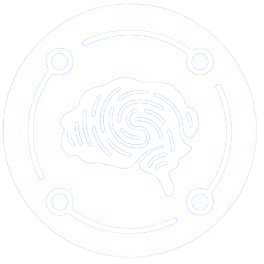
de Recherche et d’Innovation
en Cybersécurité et Société
Ouyed, O.; Allili, M. S.
Group-of-features relevance in multinomial kernel logistic regression and application to human interaction recognition Article de journal
Dans: Expert Systems with Applications, vol. 148, 2020, ISSN: 09574174, (Publisher: Elsevier Ltd).
Résumé | Liens | BibTeX | Étiquettes: Arts computing, Computationally efficient, Gradient descent, Gradient methods, Group sparsities, Group-of-features relevance, Human interaction recognition, Multinomial kernels, regression analysis, Relevance weights, State-of-art methods
@article{ouyed_group–features_2020,
title = {Group-of-features relevance in multinomial kernel logistic regression and application to human interaction recognition},
author = {O. Ouyed and M. S. Allili},
url = {https://www.scopus.com/inward/record.uri?eid=2-s2.0-85078662750&doi=10.1016%2fj.eswa.2020.113247&partnerID=40&md5=8738cfe1a8a6ded1d5f247b8c62de724},
doi = {10.1016/j.eswa.2020.113247},
issn = {09574174},
year = {2020},
date = {2020-01-01},
journal = {Expert Systems with Applications},
volume = {148},
abstract = {We propose an approach for human interaction recognition (HIR) in videos using multinomial kernel logistic regression with group-of-features relevance (GFR-MKLR). Our approach couples kernel and group sparsity modelling to ensure highly precise interaction classification. The group structure in GFR-MKLR is chosen to reflect a representation of interactions at the level of gestures, which ensures more robustness to intra-class variability due to occlusions and changes in subject appearance, body size and viewpoint. The groups consist of motion features extracted from tracking interacting persons joints over time. We encode group sparsity in GFR-MKLR through relevance weights reflecting each group (gesture) discrimination capability between different interaction categories. These weights are automatically estimated during GFR-MKLR training using gradient descent minimisation. Our model is computationally efficient and can be trained on a small training dataset while maintaining a good generalization and interpretation capabilities. Experiments on the well-known UT-Interaction dataset have demonstrated the performance of our approach by comparison with state-of-art methods. © 2020 Elsevier Ltd},
note = {Publisher: Elsevier Ltd},
keywords = {Arts computing, Computationally efficient, Gradient descent, Gradient methods, Group sparsities, Group-of-features relevance, Human interaction recognition, Multinomial kernels, regression analysis, Relevance weights, State-of-art methods},
pubstate = {published},
tppubtype = {article}
}
Ouyed, O.; Allili, M. S.
Recognizing human interactions using group feature relevance in multinomial kernel logistic regression Article d'actes
Dans: K., Rus V. Brawner (Ed.): Proceedings of the 31st International Florida Artificial Intelligence Research Society Conference, FLAIRS 2018, p. 541–545, AAAI press, 2018, ISBN: 978-1-57735-796-4.
Résumé | Liens | BibTeX | Étiquettes: Art methods, Artificial intelligence, Feature relevance, Group sparsities, Human interactions, Image features, Kernel logistic regression, Multinomial kernels, regression analysis, Sparse models
@inproceedings{ouyed_recognizing_2018,
title = {Recognizing human interactions using group feature relevance in multinomial kernel logistic regression},
author = {O. Ouyed and M. S. Allili},
editor = {Rus V. Brawner K.},
url = {https://www.scopus.com/inward/record.uri?eid=2-s2.0-85067977954&partnerID=40&md5=1c8d720de570fc565bca3741c107bc9a},
isbn = {978-1-57735-796-4},
year = {2018},
date = {2018-01-01},
booktitle = {Proceedings of the 31st International Florida Artificial Intelligence Research Society Conference, FLAIRS 2018},
pages = {541–545},
publisher = {AAAI press},
abstract = {We propose a supervised approach incorporating group feature sparsity in multi-class kernel logistic regression (GFR-MKLR). The need for group sparsity arises in several practical situations where a subset of a set of factors can explain a predicted variable and each factor consists of a group of variables. We apply our approach for predicting human interactions based on body parts motion (e.g., hands, legs, head, etc.) where image features are organised in groups corresponding to body parts. Our approach, leads to sparse models by assigning weights to groups of features having the highest discrimination between different types of interactions. Experiments conducted on the UT-Interaction dataset have demonstrated the performance of our method with regard to stat-of-art methods. Copyright © 2017, Association for the Advancement of Artificial Intelligence (www.aaai.org). All rights reserved.},
keywords = {Art methods, Artificial intelligence, Feature relevance, Group sparsities, Human interactions, Image features, Kernel logistic regression, Multinomial kernels, regression analysis, Sparse models},
pubstate = {published},
tppubtype = {inproceedings}
}
Ouyed, O.; Allili, M. S.
Feature weighting for multinomial kernel logistic regression and application to action recognition Article de journal
Dans: Neurocomputing, vol. 275, p. 1752–1768, 2018, ISSN: 09252312, (Publisher: Elsevier B.V.).
Résumé | Liens | BibTeX | Étiquettes: Action recognition, article, Classification, classification algorithm, Classification performance, Computer applications, controlled study, embedding, Feature relevance, feature relevance for multinomial kernel logistic regression, Feature weighting, Kernel logistic regression, kernel method, Learning, mathematical computing, Multinomial kernels, multinominal kernel logistic regression, Neural networks, priority journal, recognition, regression analysis, simulation, sparse modeling, Sparse models, sparse multinomial logistic regression, sparsity promoting regularization, standard, Supervised classification
@article{ouyed_feature_2018,
title = {Feature weighting for multinomial kernel logistic regression and application to action recognition},
author = {O. Ouyed and M. S. Allili},
url = {https://www.scopus.com/inward/record.uri?eid=2-s2.0-85035104467&doi=10.1016%2fj.neucom.2017.10.024&partnerID=40&md5=09687b392a405be4338799a750932cf3},
doi = {10.1016/j.neucom.2017.10.024},
issn = {09252312},
year = {2018},
date = {2018-01-01},
journal = {Neurocomputing},
volume = {275},
pages = {1752–1768},
abstract = {Multinominal kernel logistic regression (MKLR) is a supervised classification method designed for separating classes with non-linear boundaries. However, it relies on the assumption that all features are equally important, which may decrease classification performance when dealing with high-dimensional and noisy data. We propose an approach for embedding feature relevance in multinomial kernel logistic regression. Our approach, coined fr-MKLR, generalizes MKLR by introducing a feature weighting scheme in the Gaussian kernel and using the so-called ℓ0-“norm” as sparsity-promoting regularization. Therefore, the contribution of each feature is tuned according to its relevance for classification which leads to more generalizable and interpretable sparse models for classification. Application of our approach to several standard datasets and video action recognition has provided very promising results compared to other methods. © 2017 Elsevier B.V.},
note = {Publisher: Elsevier B.V.},
keywords = {Action recognition, article, Classification, classification algorithm, Classification performance, Computer applications, controlled study, embedding, Feature relevance, feature relevance for multinomial kernel logistic regression, Feature weighting, Kernel logistic regression, kernel method, Learning, mathematical computing, Multinomial kernels, multinominal kernel logistic regression, Neural networks, priority journal, recognition, regression analysis, simulation, sparse modeling, Sparse models, sparse multinomial logistic regression, sparsity promoting regularization, standard, Supervised classification},
pubstate = {published},
tppubtype = {article}
}
Ouyed, O.; Allili, M. S.
Feature relevance for kernel logistic regression and application to action classification Article d'actes
Dans: Proceedings - International Conference on Pattern Recognition, p. 1325–1329, Institute of Electrical and Electronics Engineers Inc., 2014, ISBN: 978-1-4799-5208-3, (ISSN: 10514651).
Résumé | Liens | BibTeX | Étiquettes: Action classifications, Action recognition, Classification (of information), Classification methods, Classification performance, Feature relevance, Kernel logistic regression, Logistic regression, Multinomial kernels, Pattern Recognition, Supervised classification, Support vector machines
@inproceedings{ouyed_feature_2014,
title = {Feature relevance for kernel logistic regression and application to action classification},
author = {O. Ouyed and M. S. Allili},
url = {https://www.scopus.com/inward/record.uri?eid=2-s2.0-84919884283&doi=10.1109%2fICPR.2014.237&partnerID=40&md5=616038cd7924614fd633061dfed32903},
doi = {10.1109/ICPR.2014.237},
isbn = {978-1-4799-5208-3},
year = {2014},
date = {2014-01-01},
booktitle = {Proceedings - International Conference on Pattern Recognition},
pages = {1325–1329},
publisher = {Institute of Electrical and Electronics Engineers Inc.},
abstract = {An approach is proposed for incorporating feature relevance in mutinomial kernel logistic regression (MKLR) for classification. MKLR is a supervised classification method designed for separating classes with non-linear boundaries. However, it assumes all features are equally important, which may decrease classification performance when dealing with high-dimensional or noisy data. We propose a feature weighting algorithm for MKLR which automatically tunes features contribution according to their relevance for classification and reduces data over-fitting. The proposed algorithm produces more interpretable models and is more generalizable than MKLR, Kernel-SVM and LASSO methods. Application to simulated data and video action classification has provided very promising results compared to the aforementioned classification methods. © 2014 IEEE.},
note = {ISSN: 10514651},
keywords = {Action classifications, Action recognition, Classification (of information), Classification methods, Classification performance, Feature relevance, Kernel logistic regression, Logistic regression, Multinomial kernels, Pattern Recognition, Supervised classification, Support vector machines},
pubstate = {published},
tppubtype = {inproceedings}
}