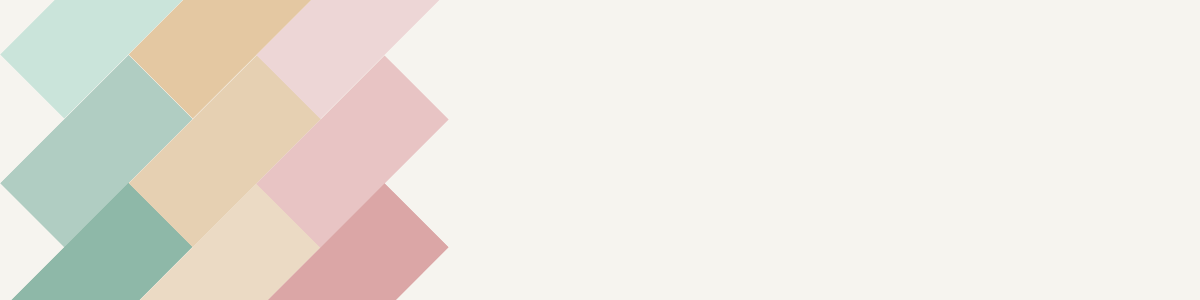
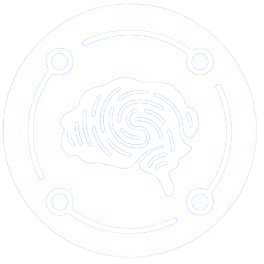
de Recherche et d’Innovation
en Cybersécurité et Société
Ouyed, O.; Allili, M. S.
Recognizing human interactions using group feature relevance in multinomial kernel logistic regression Article d'actes
Dans: K., Rus V. Brawner (Ed.): Proceedings of the 31st International Florida Artificial Intelligence Research Society Conference, FLAIRS 2018, p. 541–545, AAAI press, 2018, ISBN: 978-1-57735-796-4.
Résumé | Liens | BibTeX | Étiquettes: Art methods, Artificial intelligence, Feature relevance, Group sparsities, Human interactions, Image features, Kernel logistic regression, Multinomial kernels, regression analysis, Sparse models
@inproceedings{ouyed_recognizing_2018,
title = {Recognizing human interactions using group feature relevance in multinomial kernel logistic regression},
author = {O. Ouyed and M. S. Allili},
editor = {Rus V. Brawner K.},
url = {https://www.scopus.com/inward/record.uri?eid=2-s2.0-85067977954&partnerID=40&md5=1c8d720de570fc565bca3741c107bc9a},
isbn = {978-1-57735-796-4},
year = {2018},
date = {2018-01-01},
booktitle = {Proceedings of the 31st International Florida Artificial Intelligence Research Society Conference, FLAIRS 2018},
pages = {541–545},
publisher = {AAAI press},
abstract = {We propose a supervised approach incorporating group feature sparsity in multi-class kernel logistic regression (GFR-MKLR). The need for group sparsity arises in several practical situations where a subset of a set of factors can explain a predicted variable and each factor consists of a group of variables. We apply our approach for predicting human interactions based on body parts motion (e.g., hands, legs, head, etc.) where image features are organised in groups corresponding to body parts. Our approach, leads to sparse models by assigning weights to groups of features having the highest discrimination between different types of interactions. Experiments conducted on the UT-Interaction dataset have demonstrated the performance of our method with regard to stat-of-art methods. Copyright © 2017, Association for the Advancement of Artificial Intelligence (www.aaai.org). All rights reserved.},
keywords = {Art methods, Artificial intelligence, Feature relevance, Group sparsities, Human interactions, Image features, Kernel logistic regression, Multinomial kernels, regression analysis, Sparse models},
pubstate = {published},
tppubtype = {inproceedings}
}
Ouyed, O.; Allili, M. S.
Feature weighting for multinomial kernel logistic regression and application to action recognition Article de journal
Dans: Neurocomputing, vol. 275, p. 1752–1768, 2018, ISSN: 09252312, (Publisher: Elsevier B.V.).
Résumé | Liens | BibTeX | Étiquettes: Action recognition, article, Classification, classification algorithm, Classification performance, Computer applications, controlled study, embedding, Feature relevance, feature relevance for multinomial kernel logistic regression, Feature weighting, Kernel logistic regression, kernel method, Learning, mathematical computing, Multinomial kernels, multinominal kernel logistic regression, Neural networks, priority journal, recognition, regression analysis, simulation, sparse modeling, Sparse models, sparse multinomial logistic regression, sparsity promoting regularization, standard, Supervised classification
@article{ouyed_feature_2018,
title = {Feature weighting for multinomial kernel logistic regression and application to action recognition},
author = {O. Ouyed and M. S. Allili},
url = {https://www.scopus.com/inward/record.uri?eid=2-s2.0-85035104467&doi=10.1016%2fj.neucom.2017.10.024&partnerID=40&md5=09687b392a405be4338799a750932cf3},
doi = {10.1016/j.neucom.2017.10.024},
issn = {09252312},
year = {2018},
date = {2018-01-01},
journal = {Neurocomputing},
volume = {275},
pages = {1752–1768},
abstract = {Multinominal kernel logistic regression (MKLR) is a supervised classification method designed for separating classes with non-linear boundaries. However, it relies on the assumption that all features are equally important, which may decrease classification performance when dealing with high-dimensional and noisy data. We propose an approach for embedding feature relevance in multinomial kernel logistic regression. Our approach, coined fr-MKLR, generalizes MKLR by introducing a feature weighting scheme in the Gaussian kernel and using the so-called ℓ0-“norm” as sparsity-promoting regularization. Therefore, the contribution of each feature is tuned according to its relevance for classification which leads to more generalizable and interpretable sparse models for classification. Application of our approach to several standard datasets and video action recognition has provided very promising results compared to other methods. © 2017 Elsevier B.V.},
note = {Publisher: Elsevier B.V.},
keywords = {Action recognition, article, Classification, classification algorithm, Classification performance, Computer applications, controlled study, embedding, Feature relevance, feature relevance for multinomial kernel logistic regression, Feature weighting, Kernel logistic regression, kernel method, Learning, mathematical computing, Multinomial kernels, multinominal kernel logistic regression, Neural networks, priority journal, recognition, regression analysis, simulation, sparse modeling, Sparse models, sparse multinomial logistic regression, sparsity promoting regularization, standard, Supervised classification},
pubstate = {published},
tppubtype = {article}
}
Filali, I.; Allili, M. S.; Benblidia, N.
Multi-scale salient object detection using graph ranking and global–local saliency refinement Article de journal
Dans: Signal Processing: Image Communication, vol. 47, p. 380–401, 2016, ISSN: 09235965, (Publisher: Elsevier B.V.).
Résumé | Liens | BibTeX | Étiquettes: Algorithms, Boundary information, Decision trees, Feature relevance, Iterative methods, Multi-layer graphs, Object detection, Object recognition, Random forests, Salient object detection
@article{filali_multi-scale_2016,
title = {Multi-scale salient object detection using graph ranking and global–local saliency refinement},
author = {I. Filali and M. S. Allili and N. Benblidia},
url = {https://www.scopus.com/inward/record.uri?eid=2-s2.0-84982091007&doi=10.1016%2fj.image.2016.07.007&partnerID=40&md5=60dabe68b5cff4b5d00216d6a632e1cd},
doi = {10.1016/j.image.2016.07.007},
issn = {09235965},
year = {2016},
date = {2016-01-01},
journal = {Signal Processing: Image Communication},
volume = {47},
pages = {380–401},
abstract = {We propose an algorithm for salient object detection (SOD) based on multi-scale graph ranking and iterative local–global object refinement. Starting from a set of multi-scale image decompositions using superpixels, we propose an objective function which is optimized on a multi-layer graph structure to diffuse saliency from image borders to salient objects. This step aims at roughly estimating the location and extent of salient objects in the image. We then enhance the object saliency through an iterative process employing random forests and local boundary refinement using color, texture and edge information. We also use a feature weighting scheme to ensure optimal object/background discrimination. Our algorithm yields very accurate saliency maps for SOD while maintaining a reasonable computational time. Experiments on several standard datasets have shown that our approach outperforms several recent methods dealing with SOD. © 2016 Elsevier B.V.},
note = {Publisher: Elsevier B.V.},
keywords = {Algorithms, Boundary information, Decision trees, Feature relevance, Iterative methods, Multi-layer graphs, Object detection, Object recognition, Random forests, Salient object detection},
pubstate = {published},
tppubtype = {article}
}
Bacha, S.; Allili, M. S.; Benblidia, N.
Event recognition in photo albums using probabilistic graphical models and feature relevance Article de journal
Dans: Journal of Visual Communication and Image Representation, vol. 40, no Part B, p. 546–558, 2016, ISSN: 10473203 (ISSN), (Publisher: Academic Press Inc.).
Résumé | Liens | BibTeX | Étiquettes: Event prediction, Event recognition, Feature relevance, Graphic methods, Object/scene relevance, Photo album, Photo albums, Probabilistic graphical models, Probabilistic graphical models (PGM), Speech recognition, Visual feature
@article{bacha_event_2016,
title = {Event recognition in photo albums using probabilistic graphical models and feature relevance},
author = {S. Bacha and M. S. Allili and N. Benblidia},
url = {https://www.scopus.com/inward/record.uri?eid=2-s2.0-84992111208&doi=10.1016%2fj.jvcir.2016.07.021&partnerID=40&md5=20ebb156819c8fcae6e28949046ceb6e},
doi = {10.1016/j.jvcir.2016.07.021},
issn = {10473203 (ISSN)},
year = {2016},
date = {2016-01-01},
booktitle = {Proceedings - International Conference on Pattern Recognition},
journal = {Journal of Visual Communication and Image Representation},
volume = {40},
number = {Part B},
pages = {546–558},
publisher = {Institute of Electrical and Electronics Engineers Inc.},
abstract = {This paper proposes a method for event recognition in photo albums which aims at predicting the event categories of groups of photos. We propose a probabilistic graphical model (PGM) for event prediction based on high-level visual features consisting of objects and scenes, which are extracted directly from images. For better discrimination between different event categories, we develop a scheme to integrate feature relevance in our model which yields a more powerful inference when album images exhibit a large number of objects and scenes. It allows also to mitigate the influence of non-informative images usually contained in the albums. The performance of the proposed method is validated using extensive experiments on the recently-proposed PEC dataset containing over 61 000 images. Our method obtained the highest accuracy which outperforms previous work. © 2016 Elsevier Inc.},
note = {Publisher: Academic Press Inc.},
keywords = {Event prediction, Event recognition, Feature relevance, Graphic methods, Object/scene relevance, Photo album, Photo albums, Probabilistic graphical models, Probabilistic graphical models (PGM), Speech recognition, Visual feature},
pubstate = {published},
tppubtype = {article}
}
Bacha, S.; Allili, M. S.; Benblidia, N.
Event recognition in photo albums using probabilistic graphical model and feature relevance Article d'actes
Dans: Proceedings - International Conference on Pattern Recognition, p. 2819–2823, Institute of Electrical and Electronics Engineers Inc., 2016, ISBN: 10514651 (ISSN); 978-150904847-2 (ISBN), (Journal Abbreviation: Proc. Int. Conf. Pattern Recognit.).
Résumé | Liens | BibTeX | Étiquettes: Discriminative power, Event recognition, Feature relevance, Graphic methods, Image features, Object detection, Photo album, Probabilistic graphical models, Probabilistic graphical models (PGM), Semantic event, Semantics, Speech recognition
@inproceedings{bacha_event_2016-1,
title = {Event recognition in photo albums using probabilistic graphical model and feature relevance},
author = {S. Bacha and M. S. Allili and N. Benblidia},
url = {https://www.scopus.com/inward/record.uri?eid=2-s2.0-85019068722&doi=10.1109%2fICPR.2016.7900063&partnerID=40&md5=7aaf779f00590113afa31fde63016619},
doi = {10.1109/ICPR.2016.7900063},
isbn = {10514651 (ISSN); 978-150904847-2 (ISBN)},
year = {2016},
date = {2016-01-01},
booktitle = {Proceedings - International Conference on Pattern Recognition},
volume = {0},
pages = {2819–2823},
publisher = {Institute of Electrical and Electronics Engineers Inc.},
abstract = {The exponential use of digital cameras has raised a new problem: how to store/retrieve images/albums in very large photo databases that correspond to special events. In this paper, we propose a new probabilistic graphical model (PGM) to recognize events in photo albums stored by users. The PGM combines high-level image features consisting of scenes and objects detected in images. To consider the discriminative power of features, our model integrates the object/scene relevance for more precise prediction of semantic events in photo albums. Experimental results carried out on the challenging PEC dataset with 807 photo albums are presented. © 2016 IEEE.},
note = {Journal Abbreviation: Proc. Int. Conf. Pattern Recognit.},
keywords = {Discriminative power, Event recognition, Feature relevance, Graphic methods, Image features, Object detection, Photo album, Probabilistic graphical models, Probabilistic graphical models (PGM), Semantic event, Semantics, Speech recognition},
pubstate = {published},
tppubtype = {inproceedings}
}
Allili, M. S.; Ziou, D.
Likelihood-based feature relevance for figure-ground segmentation in images and videos Article de journal
Dans: Neurocomputing, vol. 167, p. 658–670, 2015, ISSN: 09252312, (Publisher: Elsevier).
Résumé | Liens | BibTeX | Étiquettes: accuracy, algorithm, article, calculation, Feature relevance, Figure-ground segmentations, Gaussian mixture model (GMMs), Image analysis, Image Enhancement, image quality, Image segmentation, Level Set, linear system, mathematical analysis, mathematical model, Negative examples, priority journal, Video cameras, videorecording
@article{allili_likelihood-based_2015,
title = {Likelihood-based feature relevance for figure-ground segmentation in images and videos},
author = {M. S. Allili and D. Ziou},
url = {https://www.scopus.com/inward/record.uri?eid=2-s2.0-84952631642&doi=10.1016%2fj.neucom.2015.04.015&partnerID=40&md5=833948d0784e0dc42c2245b9343971dd},
doi = {10.1016/j.neucom.2015.04.015},
issn = {09252312},
year = {2015},
date = {2015-01-01},
journal = {Neurocomputing},
volume = {167},
pages = {658–670},
abstract = {We propose an efficient method for image/video figure-ground segmentation using feature relevance (FR) and active contours. Given a set of positive and negative examples of a specific foreground (an object of interest (OOI) in an image or a tracked objet in a video), we first learn the foreground distribution model and its characteristic features that best discriminate it from its contextual background. For this goal, an objective function based on feature likelihood ratio is proposed for supervised FR computation. FR is then incorporated in foreground segmentation of new images and videos using level sets and energy minimization. We show the effectiveness of our approach on several examples of image/video figure-ground segmentation. © 2015 Elsevier B.V.},
note = {Publisher: Elsevier},
keywords = {accuracy, algorithm, article, calculation, Feature relevance, Figure-ground segmentations, Gaussian mixture model (GMMs), Image analysis, Image Enhancement, image quality, Image segmentation, Level Set, linear system, mathematical analysis, mathematical model, Negative examples, priority journal, Video cameras, videorecording},
pubstate = {published},
tppubtype = {article}
}
Ouyed, O.; Allili, M. S.
Feature relevance for kernel logistic regression and application to action classification Article d'actes
Dans: Proceedings - International Conference on Pattern Recognition, p. 1325–1329, Institute of Electrical and Electronics Engineers Inc., 2014, ISBN: 978-1-4799-5208-3, (ISSN: 10514651).
Résumé | Liens | BibTeX | Étiquettes: Action classifications, Action recognition, Classification (of information), Classification methods, Classification performance, Feature relevance, Kernel logistic regression, Logistic regression, Multinomial kernels, Pattern Recognition, Supervised classification, Support vector machines
@inproceedings{ouyed_feature_2014,
title = {Feature relevance for kernel logistic regression and application to action classification},
author = {O. Ouyed and M. S. Allili},
url = {https://www.scopus.com/inward/record.uri?eid=2-s2.0-84919884283&doi=10.1109%2fICPR.2014.237&partnerID=40&md5=616038cd7924614fd633061dfed32903},
doi = {10.1109/ICPR.2014.237},
isbn = {978-1-4799-5208-3},
year = {2014},
date = {2014-01-01},
booktitle = {Proceedings - International Conference on Pattern Recognition},
pages = {1325–1329},
publisher = {Institute of Electrical and Electronics Engineers Inc.},
abstract = {An approach is proposed for incorporating feature relevance in mutinomial kernel logistic regression (MKLR) for classification. MKLR is a supervised classification method designed for separating classes with non-linear boundaries. However, it assumes all features are equally important, which may decrease classification performance when dealing with high-dimensional or noisy data. We propose a feature weighting algorithm for MKLR which automatically tunes features contribution according to their relevance for classification and reduces data over-fitting. The proposed algorithm produces more interpretable models and is more generalizable than MKLR, Kernel-SVM and LASSO methods. Application to simulated data and video action classification has provided very promising results compared to the aforementioned classification methods. © 2014 IEEE.},
note = {ISSN: 10514651},
keywords = {Action classifications, Action recognition, Classification (of information), Classification methods, Classification performance, Feature relevance, Kernel logistic regression, Logistic regression, Multinomial kernels, Pattern Recognition, Supervised classification, Support vector machines},
pubstate = {published},
tppubtype = {inproceedings}
}
Allili, M. S.; Ziou, D.
Using feature selection for object segmentation and tracking Article d'actes
Dans: Proceedings - Fourth Canadian Conference on Computer and Robot Vision, CRV 2007, p. 191–198, Montreal, QC, 2007, ISBN: 0-7695-2786-8 978-0-7695-2786-4.
Résumé | Liens | BibTeX | Étiquettes: Active contours, Algorithms, Feature extraction, Feature relevance, Image segmentation, Maximum likelihood, Mixture models, Negative examples, Object of interest (OOI), Optimization, Target tracking
@inproceedings{allili_using_2007,
title = {Using feature selection for object segmentation and tracking},
author = {M. S. Allili and D. Ziou},
url = {https://www.scopus.com/inward/record.uri?eid=2-s2.0-34548781938&doi=10.1109%2fCRV.2007.67&partnerID=40&md5=3fb26f3fcc7a6f55f705255758fef582},
doi = {10.1109/CRV.2007.67},
isbn = {0-7695-2786-8 978-0-7695-2786-4},
year = {2007},
date = {2007-01-01},
booktitle = {Proceedings - Fourth Canadian Conference on Computer and Robot Vision, CRV 2007},
pages = {191–198},
address = {Montreal, QC},
abstract = {Most image segmentation algorithms in the past are based on optimizing an objective function that aims to achieve the similarity between several low-level features to build a partition of the image into homogeneous regions. In the present paper, we propose to incorporate the relevance (selection) of the grouping features to enforce the segmentation toward the capturing of objects of interest. The relevance of the features is determined through a set of positive and negative examples of a specific object defined a priori by the user. The calculation of the relevance of the features is performed by maximizing an objective function defined on the mixture likelihoods of the positive and negative object examples sets. The incorporation of the features relevance in the object segmentation is formulated through an energy functional which is minimized by using level set active contours. We show the efficiency of the approach on several examples of object of interest segmentation and tracking where the features relevance was used. © 2007 IEEE.},
keywords = {Active contours, Algorithms, Feature extraction, Feature relevance, Image segmentation, Maximum likelihood, Mixture models, Negative examples, Object of interest (OOI), Optimization, Target tracking},
pubstate = {published},
tppubtype = {inproceedings}
}