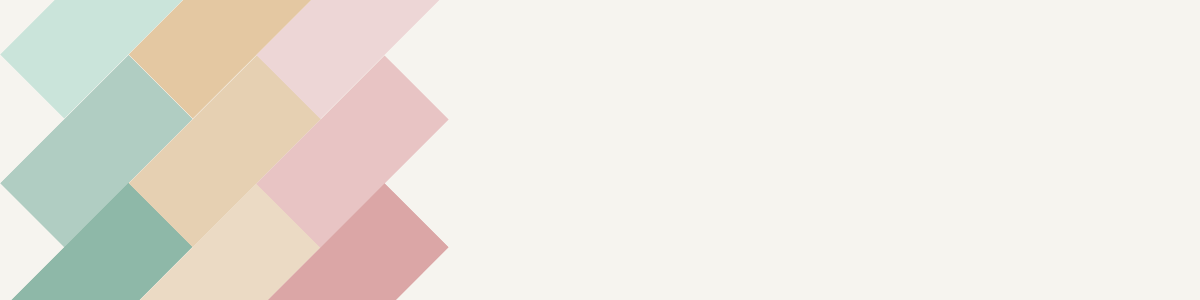
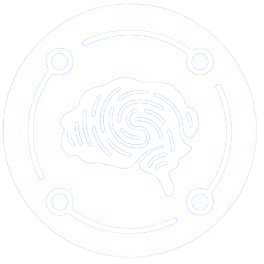
de Recherche et d’Innovation
en Cybersécurité et Société
Abdollahzadeh, S.; Proulx, P. -L.; Allili, M. S.; Lapointe, J. -F.
Safe Landing Zones Detection for UAVs Using Deep Regression Article d'actes
Dans: Proceedings - 2022 19th Conference on Robots and Vision, CRV 2022, p. 213–218, Institute of Electrical and Electronics Engineers Inc., 2022, ISBN: 978-1-66549-774-9.
Résumé | Liens | BibTeX | Étiquettes: Aerial vehicle, Air navigation, Aircraft detection, Antennas, Automatic unmanned aerial vehicle navigation, Deep learning, Deep regression, Landing, Landing zones, Safe landing, Safe landing zone, Semantic segmentation, Semantics, Unmanned aerial vehicles (UAV), Urban areas, Vehicle navigation, Zone detection
@inproceedings{abdollahzadeh_safe_2022,
title = {Safe Landing Zones Detection for UAVs Using Deep Regression},
author = {S. Abdollahzadeh and P. -L. Proulx and M. S. Allili and J. -F. Lapointe},
url = {https://www.scopus.com/inward/record.uri?eid=2-s2.0-85138466098&doi=10.1109%2fCRV55824.2022.00035&partnerID=40&md5=9183f6cd002c8a9068716faf66da72ec},
doi = {10.1109/CRV55824.2022.00035},
isbn = {978-1-66549-774-9},
year = {2022},
date = {2022-01-01},
booktitle = {Proceedings - 2022 19th Conference on Robots and Vision, CRV 2022},
pages = {213–218},
publisher = {Institute of Electrical and Electronics Engineers Inc.},
abstract = {Finding safe landing zones (SLZ) in urban areas and natural scenes is one of the many challenges that must be overcome in automating Unmanned Aerial Vehicles (UAV) navigation. Using passive vision sensors to achieve this objective is a very promising avenue due to their low cost and the potential they provide for performing simultaneous terrain analysis and 3D reconstruction. In this paper, we propose using a deep learning approach on UAV imagery to assess the SLZ. The model is built on a semantic segmentation architecture whereby thematic classes of the terrain are mapped into safety scores for UAV landing. Contrary to past methods, which use hard classification into safe/unsafe landing zones, our approach provides a continuous safety map that is more practical for an emergency landing. Experiments on public datasets have shown promising results. © 2022 IEEE.},
keywords = {Aerial vehicle, Air navigation, Aircraft detection, Antennas, Automatic unmanned aerial vehicle navigation, Deep learning, Deep regression, Landing, Landing zones, Safe landing, Safe landing zone, Semantic segmentation, Semantics, Unmanned aerial vehicles (UAV), Urban areas, Vehicle navigation, Zone detection},
pubstate = {published},
tppubtype = {inproceedings}
}
Messaoudi, H.; Belaid, A.; Allaoui, M. L.; Zetout, A.; Allili, M. S.; Tliba, S.; Salem, D. Ben; Conze, P. -H.
Efficient Embedding Network for 3D Brain Tumor Segmentation Article de journal
Dans: Lecture Notes in Computer Science (including subseries Lecture Notes in Artificial Intelligence and Lecture Notes in Bioinformatics), vol. 12658 LNCS, p. 252–262, 2021, ISSN: 03029743, (ISBN: 9783030720834 Publisher: Springer Science and Business Media Deutschland GmbH).
Résumé | Liens | BibTeX | Étiquettes: 3D medical image processing, Brain, Brain tumor segmentation, Classification networks, Convolutional neural networks, Deep learning, Embedding network, Image segmentation, Large dataset, Large datasets, Medical imaging, Natural images, Net networks, Semantic segmentation, Semantics, Signal encoding, Tumors
@article{messaoudi_efficient_2021,
title = {Efficient Embedding Network for 3D Brain Tumor Segmentation},
author = {H. Messaoudi and A. Belaid and M. L. Allaoui and A. Zetout and M. S. Allili and S. Tliba and D. Ben Salem and P. -H. Conze},
editor = {Bakas S. Crimi A.},
url = {https://www.scopus.com/inward/record.uri?eid=2-s2.0-85107387134&doi=10.1007%2f978-3-030-72084-1_23&partnerID=40&md5=b3aa3516b0465a1bf5611db4727d95f1},
doi = {10.1007/978-3-030-72084-1_23},
issn = {03029743},
year = {2021},
date = {2021-01-01},
journal = {Lecture Notes in Computer Science (including subseries Lecture Notes in Artificial Intelligence and Lecture Notes in Bioinformatics)},
volume = {12658 LNCS},
pages = {252–262},
abstract = {3D medical image processing with deep learning greatly suffers from a lack of data. Thus, studies carried out in this field are limited compared to works related to 2D natural image analysis, where very large datasets exist. As a result, powerful and efficient 2D convolutional neural networks have been developed and trained. In this paper, we investigate a way to transfer the performance of a two-dimensional classification network for the purpose of three-dimensional semantic segmentation of brain tumors. We propose an asymmetric U-Net network by incorporating the EfficientNet model as part of the encoding branch. As the input data is in 3D, the first layers of the encoder are devoted to the reduction of the third dimension in order to fit the input of the EfficientNet network. Experimental results on validation and test data from the BraTS 2020 challenge demonstrate that the proposed method achieve promising performance. © 2021, Springer Nature Switzerland AG.},
note = {ISBN: 9783030720834
Publisher: Springer Science and Business Media Deutschland GmbH},
keywords = {3D medical image processing, Brain, Brain tumor segmentation, Classification networks, Convolutional neural networks, Deep learning, Embedding network, Image segmentation, Large dataset, Large datasets, Medical imaging, Natural images, Net networks, Semantic segmentation, Semantics, Signal encoding, Tumors},
pubstate = {published},
tppubtype = {article}
}