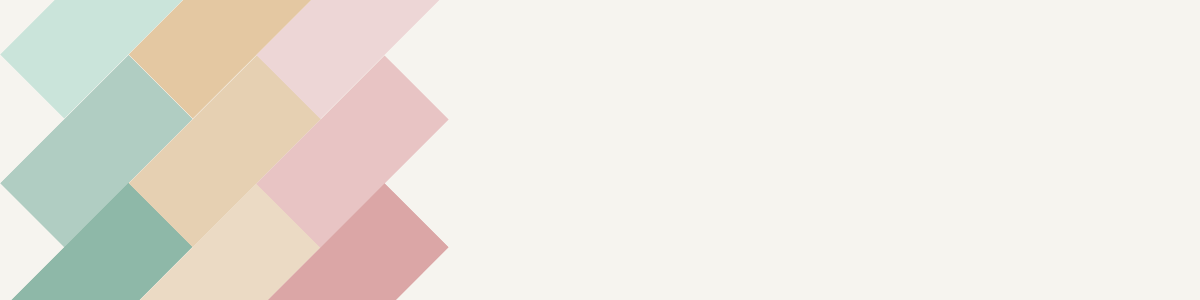
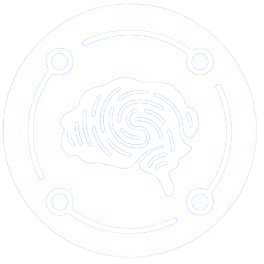
de Recherche et d’Innovation
en Cybersécurité et Société
Yapi, D.; Nouboukpo, A.; Allili, M. S.; Member, IEEE
Mixture of multivariate generalized Gaussians for multi-band texture modeling and representation Article de journal
Dans: Signal Processing, vol. 209, 2023, ISSN: 01651684, (Publisher: Elsevier B.V.).
Résumé | Liens | BibTeX | Étiquettes: Color texture retrieval, Content-based, Content-based color-texture retrieval, Convolution, convolutional neural network, Gaussians, Image retrieval, Image texture, Mixture of multivariate generalized gaussians, Multi-scale Decomposition, Subbands, Texture representation, Textures
@article{yapi_mixture_2023,
title = {Mixture of multivariate generalized Gaussians for multi-band texture modeling and representation},
author = {D. Yapi and A. Nouboukpo and M. S. Allili and IEEE Member},
url = {https://www.scopus.com/inward/record.uri?eid=2-s2.0-85151300047&doi=10.1016%2fj.sigpro.2023.109011&partnerID=40&md5=3bf98e9667eb7b60cb3f59ed1dcb029c},
doi = {10.1016/j.sigpro.2023.109011},
issn = {01651684},
year = {2023},
date = {2023-01-01},
journal = {Signal Processing},
volume = {209},
abstract = {We present a unified statistical model for multivariate and multi-modal texture representation. This model is based on the formalism of finite mixtures of multivariate generalized Gaussians (MoMGG) which enables to build a compact and accurate representation of texture images using multi-resolution texture transforms. The MoMGG model enables to describe the joint statistics of subbands in different scales and orientations, as well as between adjacent locations within the same subband, providing a precise description of the texture layout. It can also combine different multi-scale transforms to build a richer and more representative texture signature for image similarity measurement. We tested our model on both traditional texture transforms (e.g., wavelets, contourlets, maximum response filter) and convolution neural networks (CNNs) features (e.g., ResNet, SqueezeNet). Experiments on color-texture image retrieval have demonstrated the performance of our approach comparatively to state-of-the-art methods. © 2023},
note = {Publisher: Elsevier B.V.},
keywords = {Color texture retrieval, Content-based, Content-based color-texture retrieval, Convolution, convolutional neural network, Gaussians, Image retrieval, Image texture, Mixture of multivariate generalized gaussians, Multi-scale Decomposition, Subbands, Texture representation, Textures},
pubstate = {published},
tppubtype = {article}
}
Yapi, D.; Allili, M. S.
Multi-Band Texture Modeling Using Finite Mixtures of Multivariate Generalized Gaussian Distributions Article d'actes
Dans: Proceedings - International Conference on Pattern Recognition, p. 464–469, Institute of Electrical and Electronics Engineers Inc., 2022, ISBN: 978-1-66549-062-7, (ISSN: 10514651).
Résumé | Liens | BibTeX | Étiquettes: Color texture retrieval, Finite mixtures, Gaussian distribution, Gaussians, Image retrieval, Image texture, Mixture of multivariate generalized gaussians, Multi band, Multi-scale Decomposition, Multivariate generalized gaussian distributions, Statistic modeling, Subbands, Texture models, Textures
@inproceedings{yapi_multi-band_2022,
title = {Multi-Band Texture Modeling Using Finite Mixtures of Multivariate Generalized Gaussian Distributions},
author = {D. Yapi and M. S. Allili},
url = {https://www.scopus.com/inward/record.uri?eid=2-s2.0-85143638804&doi=10.1109%2fICPR56361.2022.9956448&partnerID=40&md5=3fe34da98f314b85014059630e4c8c6c},
doi = {10.1109/ICPR56361.2022.9956448},
isbn = {978-1-66549-062-7},
year = {2022},
date = {2022-01-01},
booktitle = {Proceedings - International Conference on Pattern Recognition},
volume = {2022-August},
pages = {464–469},
publisher = {Institute of Electrical and Electronics Engineers Inc.},
abstract = {We present a unified statistical model for multivariate and multi-modal texture representation. This model is based on the formalism of finite mixtures of multivariate generalized Gaussians (MoMGG) which enables a compact and accurate representation of joint statistics of different sub-bands of multireslotion texture transform. This representation expresses correlation between sub-bands at different scales and orientations, and also between adjacent locations within the same subbands, providing a precise description of the texture layout. It enables also to combine different multi-scale transforms to build a richer and more representative texture signature. We successfully tested the model on traditional texture transforms such as wavelets and contourlets. Experiments on color-texture image retrieval have demonstrated the performance of our approach comparatively to state-of-art methods. © 2022 IEEE.},
note = {ISSN: 10514651},
keywords = {Color texture retrieval, Finite mixtures, Gaussian distribution, Gaussians, Image retrieval, Image texture, Mixture of multivariate generalized gaussians, Multi band, Multi-scale Decomposition, Multivariate generalized gaussian distributions, Statistic modeling, Subbands, Texture models, Textures},
pubstate = {published},
tppubtype = {inproceedings}
}
Yapi, D.; Mejri, M.; Allili, M. S.; Baaziz, N.
A learning-based approach for automatic defect detection in textile images Article d'actes
Dans: A., Zaremba M. Sasiadek J. Dolgui (Ed.): IFAC-PapersOnLine, p. 2423–2428, 2015, ISBN: 24058963 (ISSN), (Issue: 3 Journal Abbreviation: IFAC-PapersOnLine).
Résumé | Liens | BibTeX | Étiquettes: Algorithms, Artificial intelligence, Automatic defect detections, Barium compounds, Bayes Classifier, Computational efficiency, Contourlets, Defect detection, Defect detection algorithm, Defects, Detection problems, Feature extraction, Feature extraction and classification, Gaussians, Image classification, Learning algorithms, Learning systems, Learning-based approach, Machine learning approaches, Mixture of generalized gaussians, Mixtures of generalized Gaussians (MoGG), Textile defect detection, Textile images, Textiles, Textures
@inproceedings{yapi_learning-based_2015,
title = {A learning-based approach for automatic defect detection in textile images},
author = {D. Yapi and M. Mejri and M. S. Allili and N. Baaziz},
editor = {Zaremba M. Sasiadek J. Dolgui A.},
url = {https://www.scopus.com/inward/record.uri?eid=2-s2.0-84953865559&doi=10.1016%2fj.ifacol.2015.06.451&partnerID=40&md5=3dd0ef4c27cbd55700f6511af5f46772},
doi = {10.1016/j.ifacol.2015.06.451},
isbn = {24058963 (ISSN)},
year = {2015},
date = {2015-01-01},
booktitle = {IFAC-PapersOnLine},
volume = {28},
pages = {2423–2428},
abstract = {This paper addresses the textile defect detection problem using a machine-learning approach. We propose a novel algorithm that uses supervised learning to classify textile textures in defect and non-defect classes based on suitable feature extraction and classification. We use statistical modeling of multi-scale contourlet image decomposition to obtain compact and accurate signatures for texture description. Our defect detection algorithm is based on two phases. In the first phase, using a training set of images, we extract reference defect-free signatures for each textile category. Then, we use the Bayes classifier (BC) to learn signatures of defected and non-defected classes. In the second phase, defects are detected on new images using the trained BC and an appropriate decomposition of images into blocks. Our algorithm has the capability to achieve highly accurate defect detection and localisation in textile textures while ensuring an efficient computational time. Compared to recent state-of-the-art methods, our algorithm has yielded better results on the standard TILDA database. © 2015, IFAC (International Federation of Automatic Control) Hosting by Elsevier Ltd. All rights reserved.},
note = {Issue: 3
Journal Abbreviation: IFAC-PapersOnLine},
keywords = {Algorithms, Artificial intelligence, Automatic defect detections, Barium compounds, Bayes Classifier, Computational efficiency, Contourlets, Defect detection, Defect detection algorithm, Defects, Detection problems, Feature extraction, Feature extraction and classification, Gaussians, Image classification, Learning algorithms, Learning systems, Learning-based approach, Machine learning approaches, Mixture of generalized gaussians, Mixtures of generalized Gaussians (MoGG), Textile defect detection, Textile images, Textiles, Textures},
pubstate = {published},
tppubtype = {inproceedings}
}
Allili, M. S.; Bouguila, N.; Ziou, D.
Finite general Gaussian mixture modeling and application to image and video foreground segmentation Article de journal
Dans: Journal of Electronic Imaging, vol. 17, no 1, 2008, ISSN: 10179909.
Résumé | Liens | BibTeX | Étiquettes: Finite mixture models, Foreground segmentation, Gaussian distribution, Gaussian mixture modeling, Gaussian mixtures, Gaussians, General Gaussian distribution, Image segmentation, Information theory, Information-theoretic approach, Maximum likelihood estimation, Mixture model, Mixtures, Noisy data, Overfitting
@article{allili_finite_2008,
title = {Finite general Gaussian mixture modeling and application to image and video foreground segmentation},
author = {M. S. Allili and N. Bouguila and D. Ziou},
url = {https://www.scopus.com/inward/record.uri?eid=2-s2.0-78149489170&doi=10.1117%2f1.2898125&partnerID=40&md5=8a9b3060dda2366f17b22a06606a9f09},
doi = {10.1117/1.2898125},
issn = {10179909},
year = {2008},
date = {2008-01-01},
journal = {Journal of Electronic Imaging},
volume = {17},
number = {1},
abstract = {We propose a new finite mixture model based on the formalism of general Gaussian distribution (GGD). Because it has the flexibility to adapt to the shape of the data better than the Gaussian, the GGD is less prone to overfitting the number of mixture classes when dealing with noisy data. In the first part of this work, we propose a derivation of the maximum likelihood estimation for the parameters of the new mixture model, and elaborate an information-theoretic approach for the selection of the number of classes. In the second part, we validate the proposed model by comparing it to the Gaussian mixture in applications related to image and video foreground segmentation © 2008 SPIE and IS&T.},
keywords = {Finite mixture models, Foreground segmentation, Gaussian distribution, Gaussian mixture modeling, Gaussian mixtures, Gaussians, General Gaussian distribution, Image segmentation, Information theory, Information-theoretic approach, Maximum likelihood estimation, Mixture model, Mixtures, Noisy data, Overfitting},
pubstate = {published},
tppubtype = {article}
}