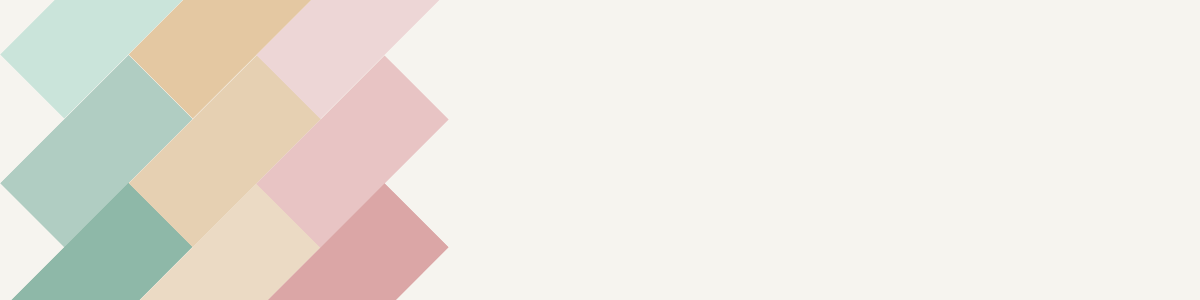
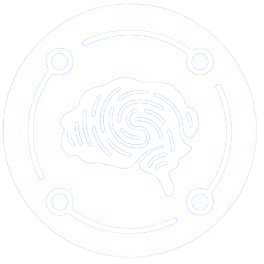
de Recherche et d’Innovation
en Cybersécurité et Société
Allili, M. S.; Ziou, D.; Bouguila, N.; Boutemedjet, S.
Image and video segmentation by combining unsupervised generalized Gaussian mixture modeling and feature selection Article de journal
Dans: IEEE Transactions on Circuits and Systems for Video Technology, vol. 20, no 10, p. 1373–1377, 2010, ISSN: 10518215.
Résumé | Liens | BibTeX | Étiquettes: Clustering model, Feature extraction, Feature selection, Gaussian distribution, Generalized Gaussian, Heavy-tailed, High dimensional spaces, Image and video segmentation, Image segmentation, image/video segmentation, Minimum message lengths, Real-world image, Video cameras
@article{allili_image_2010,
title = {Image and video segmentation by combining unsupervised generalized Gaussian mixture modeling and feature selection},
author = {M. S. Allili and D. Ziou and N. Bouguila and S. Boutemedjet},
url = {https://www.scopus.com/inward/record.uri?eid=2-s2.0-77957964550&doi=10.1109%2fTCSVT.2010.2077483&partnerID=40&md5=d888c7fe52eff37a5744bccd6a4d3d9e},
doi = {10.1109/TCSVT.2010.2077483},
issn = {10518215},
year = {2010},
date = {2010-01-01},
journal = {IEEE Transactions on Circuits and Systems for Video Technology},
volume = {20},
number = {10},
pages = {1373–1377},
abstract = {In this letter, we propose a clustering model that efficiently mitigates image and video under/over-segmentation by combining generalized Gaussian mixture modeling and feature selection. The model has flexibility to accurately represent heavy-tailed image/video histograms, while automatically discarding uninformative features, leading to better discrimination and localization of regions in high-dimensional spaces. Experimental results on a database of real-world images and videos showed us the effectiveness of the proposed approach. © 2010 IEEE.},
keywords = {Clustering model, Feature extraction, Feature selection, Gaussian distribution, Generalized Gaussian, Heavy-tailed, High dimensional spaces, Image and video segmentation, Image segmentation, image/video segmentation, Minimum message lengths, Real-world image, Video cameras},
pubstate = {published},
tppubtype = {article}
}
Ziou, D.; Bouguila, N.; Allili, M. S.; El-Zaart, A.
Finite Gamma mixture modelling using minimum message length inference: Application to SAR image analysis Article de journal
Dans: International Journal of Remote Sensing, vol. 30, no 3, p. 771–792, 2009, ISSN: 01431161, (Publisher: Taylor and Francis Ltd.).
Résumé | Liens | BibTeX | Étiquettes: Change detection, Determining the number of clusters, estimation method, finite element method, Finite mixtures, Gamma distribution, Gamma mixtures, Image analysis, Image processing, Image segmentation, Minimum message lengths, Mixtures, Number of clusters, numerical model, Probability distributions, Radar imaging, SAR image segmentation, Synthetic aperture radar, Unsupervised learning
@article{ziou_finite_2009,
title = {Finite Gamma mixture modelling using minimum message length inference: Application to SAR image analysis},
author = {D. Ziou and N. Bouguila and M. S. Allili and A. El-Zaart},
url = {https://www.scopus.com/inward/record.uri?eid=2-s2.0-67650686123&doi=10.1080%2f01431160802392646&partnerID=40&md5=901ea39ad806dcb62cd630585469af60},
doi = {10.1080/01431160802392646},
issn = {01431161},
year = {2009},
date = {2009-01-01},
journal = {International Journal of Remote Sensing},
volume = {30},
number = {3},
pages = {771–792},
abstract = {This paper discusses the unsupervised learning problem for finite mixtures of Gamma distributions. An important part of this problem is determining the number of clusters which best describes a set of data. We apply the Minimum Message Length (MML) criterion to the unsupervised learning problem in the case of finite mixtures of Gamma distributions. The MML and other criteria in the literature are compared in terms of their ability to estimate the number of clusters in a data set. The comparison utilizes synthetic and RADARSAT SAR images. The performance of our method is also tested by contextual evaluations involving SAR image segmentation and change detection.},
note = {Publisher: Taylor and Francis Ltd.},
keywords = {Change detection, Determining the number of clusters, estimation method, finite element method, Finite mixtures, Gamma distribution, Gamma mixtures, Image analysis, Image processing, Image segmentation, Minimum message lengths, Mixtures, Number of clusters, numerical model, Probability distributions, Radar imaging, SAR image segmentation, Synthetic aperture radar, Unsupervised learning},
pubstate = {published},
tppubtype = {article}
}