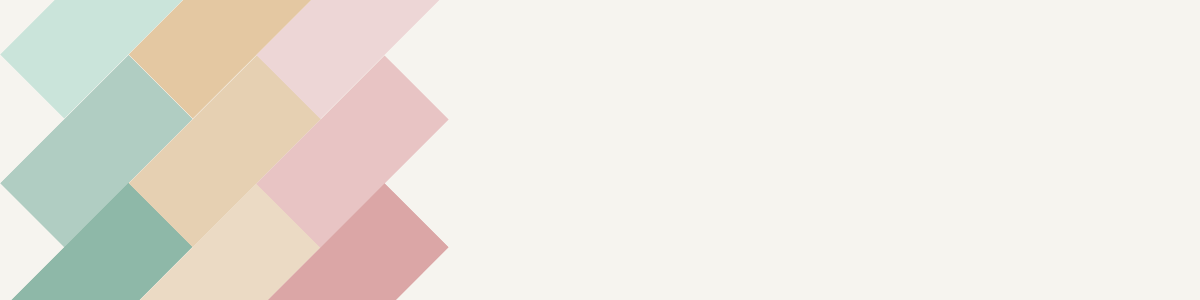
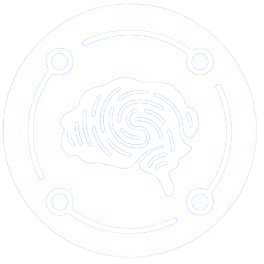
de Recherche et d’Innovation
en Cybersécurité et Société
Amirkhani, D.; Allili, M. S.; Hebbache, L.; Hammouche, N.; Lapointe, J.
Visual Concrete Bridge Defect Classification and Detection Using Deep Learning: A Systematic Review Article de journal
Dans: IEEE Transactions on Intelligent Transportation Systems, p. 1–23, 2024, ISSN: 15249050, (Publisher: Institute of Electrical and Electronics Engineers Inc.).
Résumé | Liens | BibTeX | Étiquettes: Annotation, Annotations, Bridges, Classification, Concrete, Concrete bridge defect, Concrete bridge defects, Concrete bridges, Concrete defects, Concretes, Deep learning, Defect classification, Defect detection, Defects, Detection, Inspection, Reviews, Segmentation, Taxonomies, Visualization
@article{amirkhani_visual_2024,
title = {Visual Concrete Bridge Defect Classification and Detection Using Deep Learning: A Systematic Review},
author = {D. Amirkhani and M. S. Allili and L. Hebbache and N. Hammouche and J. Lapointe},
url = {https://www.scopus.com/inward/record.uri?eid=2-s2.0-85186994244&doi=10.1109%2fTITS.2024.3365296&partnerID=40&md5=a9228252d620ad6d444cc395296ebac2},
doi = {10.1109/TITS.2024.3365296},
issn = {15249050},
year = {2024},
date = {2024-01-01},
journal = {IEEE Transactions on Intelligent Transportation Systems},
pages = {1–23},
abstract = {Visual inspection is an important process for maintaining bridges in road transportation systems, and preventing catastrophic events and tragedies. In this process, accurate and automatic concrete defect classification and detection are major components to ensure early identification of any issue that can compromise the bridge safety and integrity. While a tremendous body of research has been proposed in the last decades for addressing these problems, the advent of deep learning unleashed huge opportunities for building more accurate and efficient methods. Our aim in this survey is to study the recent progress of vision-based concrete bridge defect classification and detection in the deep learning era. Our review encompasses major aspects underlying typical frameworks, which include concrete defect taxonomy, public datasets and evaluation metrics. We provide also a taxonomy of deep-learning-based classification and detection algorithms with a detailed discussion of their advantages and limitations. We also benchmark baseline models for classification and detection, using two popular datasets. We finally discuss important challenges of concrete defect classification and detection, and promising research avenues to build better models and integrate them in real-world visual inspection systems, which warrant further scientific investigation. IEEE},
note = {Publisher: Institute of Electrical and Electronics Engineers Inc.},
keywords = {Annotation, Annotations, Bridges, Classification, Concrete, Concrete bridge defect, Concrete bridge defects, Concrete bridges, Concrete defects, Concretes, Deep learning, Defect classification, Defect detection, Defects, Detection, Inspection, Reviews, Segmentation, Taxonomies, Visualization},
pubstate = {published},
tppubtype = {article}
}
Nouboukpo, A.; Allaoui, M. L.; Allili, M. S.
Multi-scale spatial consistency for deep semi-supervised skin lesion segmentation Article de journal
Dans: Engineering Applications of Artificial Intelligence, vol. 135, 2024, ISSN: 09521976 (ISSN), (Publisher: Elsevier Ltd).
Résumé | Liens | BibTeX | Étiquettes: Deep learning, Dermatology, Image segmentation, Lesion segmentations, Medical imaging, Multi-scales, Semi-supervised, Semi-supervised learning, Skin lesion, Skin lesion segmentation, Spatial consistency, Spatially constrained mixture model, Spatially-constrained mixture models, Supervised learning, UNets, Unlabeled data
@article{nouboukpo_multi-scale_2024,
title = {Multi-scale spatial consistency for deep semi-supervised skin lesion segmentation},
author = {A. Nouboukpo and M. L. Allaoui and M. S. Allili},
url = {https://www.scopus.com/inward/record.uri?eid=2-s2.0-85195700182&doi=10.1016%2fj.engappai.2024.108681&partnerID=40&md5=e1cc2b6a1bb0aed530e8c04583c76167},
doi = {10.1016/j.engappai.2024.108681},
issn = {09521976 (ISSN)},
year = {2024},
date = {2024-01-01},
journal = {Engineering Applications of Artificial Intelligence},
volume = {135},
abstract = {This paper introduces a novel semi-supervised framework, the Multiscale Spatial Consistency Network (MSCNet), for robust semi-supervised skin lesion segmentation. MSCNet uses local and global spatial consistency to leverage a minimal set of labeled data, supplemented by a large number of unlabeled data, to improve segmentation. The model is is based on a single Encoder–Decoder (ED) network, augmented with a Spatially-Constrained Mixture Model (SCMM) to enforce spatial coherence in predictions. To encode the local spatial consistency, a hierarchical superpixel structure is used capture local region context (LRC), bolstering the model capacity to discern fine-grained lesion details. Global consistency is enforced through the SCMM module, which uses a larger context for lesion/background discrimination. In addition, it enables efficient leveraging of the unlabeled data through pseudo-label generation. Experiments demonstrate that the MSCNet outperforms existing state-of-the-art methods in segmenting complex lesions. The MSCNet has an excellent generalization capability, offering a promising direction for semi-supervised medical image segmentation, particularly in scenarios with limited annotated data. The code is available at https://github.com/AdamaTG/MSCNet. © 2024 Elsevier Ltd},
note = {Publisher: Elsevier Ltd},
keywords = {Deep learning, Dermatology, Image segmentation, Lesion segmentations, Medical imaging, Multi-scales, Semi-supervised, Semi-supervised learning, Skin lesion, Skin lesion segmentation, Spatial consistency, Spatially constrained mixture model, Spatially-constrained mixture models, Supervised learning, UNets, Unlabeled data},
pubstate = {published},
tppubtype = {article}
}
Hebbache, L.; Amirkhani, D.; Allili, M. S.; Hammouche, N.; Lapointe, J. -F.
Leveraging Saliency in Single-Stage Multi-Label Concrete Defect Detection Using Unmanned Aerial Vehicle Imagery Article de journal
Dans: Remote Sensing, vol. 15, no. 5, 2023, ISSN: 20724292, (Publisher: MDPI).
Résumé | Liens | BibTeX | Étiquettes: Aerial vehicle, Aircraft detection, Antennas, Computational efficiency, Concrete defects, Deep learning, Defect detection, extraction, Feature extraction, Features extraction, Image acquisition, Image Enhancement, Multi-labels, One-stage concrete defect detection, Saliency, Single stage, Unmanned aerial vehicles (UAV), Unmanned areal vehicle imagery
@article{hebbache_leveraging_2023,
title = {Leveraging Saliency in Single-Stage Multi-Label Concrete Defect Detection Using Unmanned Aerial Vehicle Imagery},
author = {L. Hebbache and D. Amirkhani and M. S. Allili and N. Hammouche and J. -F. Lapointe},
url = {https://www.scopus.com/inward/record.uri?eid=2-s2.0-85149966766&doi=10.3390%2frs15051218&partnerID=40&md5=7bf1cb3353270c696c07ff24dc24655d},
doi = {10.3390/rs15051218},
issn = {20724292},
year = {2023},
date = {2023-01-01},
journal = {Remote Sensing},
volume = {15},
number = {5},
abstract = {Visual inspection of concrete structures using Unmanned Areal Vehicle (UAV) imagery is a challenging task due to the variability of defects’ size and appearance. This paper proposes a high-performance model for automatic and fast detection of bridge concrete defects using UAV-acquired images. Our method, coined the Saliency-based Multi-label Defect Detector (SMDD-Net), combines pyramidal feature extraction and attention through a one-stage concrete defect detection model. The attention module extracts local and global saliency features, which are scaled and integrated with the pyramidal feature extraction module of the network using the max-pooling, multiplication, and residual skip connections operations. This has the effect of enhancing the localisation of small and low-contrast defects, as well as the overall accuracy of detection in varying image acquisition ranges. Finally, a multi-label loss function detection is used to identify and localise overlapping defects. The experimental results on a standard dataset and real-world images demonstrated the performance of SMDD-Net with regard to state-of-the-art techniques. The accuracy and computational efficiency of SMDD-Net make it a suitable method for UAV-based bridge structure inspection. © 2023 by the authors.},
note = {Publisher: MDPI},
keywords = {Aerial vehicle, Aircraft detection, Antennas, Computational efficiency, Concrete defects, Deep learning, Defect detection, extraction, Feature extraction, Features extraction, Image acquisition, Image Enhancement, Multi-labels, One-stage concrete defect detection, Saliency, Single stage, Unmanned aerial vehicles (UAV), Unmanned areal vehicle imagery},
pubstate = {published},
tppubtype = {article}
}
Yapi, D.; Nouboukpo, A.; Allili, M. S.; Member, IEEE
Mixture of multivariate generalized Gaussians for multi-band texture modeling and representation Article de journal
Dans: Signal Processing, vol. 209, 2023, ISSN: 01651684, (Publisher: Elsevier B.V.).
Résumé | Liens | BibTeX | Étiquettes: Color texture retrieval, Content-based, Content-based color-texture retrieval, Convolution, convolutional neural network, Gaussians, Image retrieval, Image texture, Mixture of multivariate generalized gaussians, Multi-scale Decomposition, Subbands, Texture representation, Textures
@article{yapi_mixture_2023,
title = {Mixture of multivariate generalized Gaussians for multi-band texture modeling and representation},
author = {D. Yapi and A. Nouboukpo and M. S. Allili and IEEE Member},
url = {https://www.scopus.com/inward/record.uri?eid=2-s2.0-85151300047&doi=10.1016%2fj.sigpro.2023.109011&partnerID=40&md5=3bf98e9667eb7b60cb3f59ed1dcb029c},
doi = {10.1016/j.sigpro.2023.109011},
issn = {01651684},
year = {2023},
date = {2023-01-01},
journal = {Signal Processing},
volume = {209},
abstract = {We present a unified statistical model for multivariate and multi-modal texture representation. This model is based on the formalism of finite mixtures of multivariate generalized Gaussians (MoMGG) which enables to build a compact and accurate representation of texture images using multi-resolution texture transforms. The MoMGG model enables to describe the joint statistics of subbands in different scales and orientations, as well as between adjacent locations within the same subband, providing a precise description of the texture layout. It can also combine different multi-scale transforms to build a richer and more representative texture signature for image similarity measurement. We tested our model on both traditional texture transforms (e.g., wavelets, contourlets, maximum response filter) and convolution neural networks (CNNs) features (e.g., ResNet, SqueezeNet). Experiments on color-texture image retrieval have demonstrated the performance of our approach comparatively to state-of-the-art methods. © 2023},
note = {Publisher: Elsevier B.V.},
keywords = {Color texture retrieval, Content-based, Content-based color-texture retrieval, Convolution, convolutional neural network, Gaussians, Image retrieval, Image texture, Mixture of multivariate generalized gaussians, Multi-scale Decomposition, Subbands, Texture representation, Textures},
pubstate = {published},
tppubtype = {article}
}
Lapointe, J. -F.; Allili, M. S.; Belliveau, L.; Hebbache, L.; Amirkhani, D.; Sekkati, H.
AI-AR for Bridge Inspection by Drone Article de journal
Dans: Lecture Notes in Computer Science (including subseries Lecture Notes in Artificial Intelligence and Lecture Notes in Bioinformatics), vol. 13318 LNCS, p. 302–313, 2022, ISSN: 03029743, (ISBN: 9783031060144 Publisher: Springer Science and Business Media Deutschland GmbH).
Résumé | Liens | BibTeX | Étiquettes: AR, augmented reality, Bridge inspection, Bridges, Deep learning, Drone, Drones, Human-in-the-loop, Inspection, Regular inspections, Remote guidance, RPAS, Transportation infrastructures, Visual inspection
@article{lapointe_ai-ar_2022,
title = {AI-AR for Bridge Inspection by Drone},
author = {J. -F. Lapointe and M. S. Allili and L. Belliveau and L. Hebbache and D. Amirkhani and H. Sekkati},
editor = {Fragomeni G. Chen J.Y.},
url = {https://www.scopus.com/inward/record.uri?eid=2-s2.0-85131961739&doi=10.1007%2f978-3-031-06015-1_21&partnerID=40&md5=f57dfc1d9207b936684f18893eb5bfa7},
doi = {10.1007/978-3-031-06015-1_21},
issn = {03029743},
year = {2022},
date = {2022-01-01},
journal = {Lecture Notes in Computer Science (including subseries Lecture Notes in Artificial Intelligence and Lecture Notes in Bioinformatics)},
volume = {13318 LNCS},
pages = {302–313},
abstract = {Good and regular inspections of transportation infrastructures such as bridges and overpasses are necessary to maintain the safety of the public who uses them and the integrity of the structures. Until recently, these inspections were done entirely manually by using mainly visual inspection to detect defects on the structure. In the last few years, inspection by drone is an emerging way of achieving inspection that allows more efficient access to the structure. This paper describes a human-in-the-loop system that combines AI and AR for bridge inspection by drone. © 2022, Springer Nature Switzerland AG.},
note = {ISBN: 9783031060144
Publisher: Springer Science and Business Media Deutschland GmbH},
keywords = {AR, augmented reality, Bridge inspection, Bridges, Deep learning, Drone, Drones, Human-in-the-loop, Inspection, Regular inspections, Remote guidance, RPAS, Transportation infrastructures, Visual inspection},
pubstate = {published},
tppubtype = {article}
}
Abdollahzadeh, S.; Proulx, P. -L.; Allili, M. S.; Lapointe, J. -F.
Safe Landing Zones Detection for UAVs Using Deep Regression Proceedings Article
Dans: Proceedings - 2022 19th Conference on Robots and Vision, CRV 2022, p. 213–218, Institute of Electrical and Electronics Engineers Inc., 2022, ISBN: 978-1-66549-774-9.
Résumé | Liens | BibTeX | Étiquettes: Aerial vehicle, Air navigation, Aircraft detection, Antennas, Automatic unmanned aerial vehicle navigation, Deep learning, Deep regression, Landing, Landing zones, Safe landing, Safe landing zone, Semantic segmentation, Semantics, Unmanned aerial vehicles (UAV), Urban areas, Vehicle navigation, Zone detection
@inproceedings{abdollahzadeh_safe_2022,
title = {Safe Landing Zones Detection for UAVs Using Deep Regression},
author = {S. Abdollahzadeh and P. -L. Proulx and M. S. Allili and J. -F. Lapointe},
url = {https://www.scopus.com/inward/record.uri?eid=2-s2.0-85138466098&doi=10.1109%2fCRV55824.2022.00035&partnerID=40&md5=9183f6cd002c8a9068716faf66da72ec},
doi = {10.1109/CRV55824.2022.00035},
isbn = {978-1-66549-774-9},
year = {2022},
date = {2022-01-01},
booktitle = {Proceedings - 2022 19th Conference on Robots and Vision, CRV 2022},
pages = {213–218},
publisher = {Institute of Electrical and Electronics Engineers Inc.},
abstract = {Finding safe landing zones (SLZ) in urban areas and natural scenes is one of the many challenges that must be overcome in automating Unmanned Aerial Vehicles (UAV) navigation. Using passive vision sensors to achieve this objective is a very promising avenue due to their low cost and the potential they provide for performing simultaneous terrain analysis and 3D reconstruction. In this paper, we propose using a deep learning approach on UAV imagery to assess the SLZ. The model is built on a semantic segmentation architecture whereby thematic classes of the terrain are mapped into safety scores for UAV landing. Contrary to past methods, which use hard classification into safe/unsafe landing zones, our approach provides a continuous safety map that is more practical for an emergency landing. Experiments on public datasets have shown promising results. © 2022 IEEE.},
keywords = {Aerial vehicle, Air navigation, Aircraft detection, Antennas, Automatic unmanned aerial vehicle navigation, Deep learning, Deep regression, Landing, Landing zones, Safe landing, Safe landing zone, Semantic segmentation, Semantics, Unmanned aerial vehicles (UAV), Urban areas, Vehicle navigation, Zone detection},
pubstate = {published},
tppubtype = {inproceedings}
}
Yapi, D.; Allili, M. S.
Multi-Band Texture Modeling Using Finite Mixtures of Multivariate Generalized Gaussian Distributions Proceedings Article
Dans: Proceedings - International Conference on Pattern Recognition, p. 464–469, Institute of Electrical and Electronics Engineers Inc., 2022, ISBN: 978-1-66549-062-7, (ISSN: 10514651).
Résumé | Liens | BibTeX | Étiquettes: Color texture retrieval, Finite mixtures, Gaussian distribution, Gaussians, Image retrieval, Image texture, Mixture of multivariate generalized gaussians, Multi band, Multi-scale Decomposition, Multivariate generalized gaussian distributions, Statistic modeling, Subbands, Texture models, Textures
@inproceedings{yapi_multi-band_2022,
title = {Multi-Band Texture Modeling Using Finite Mixtures of Multivariate Generalized Gaussian Distributions},
author = {D. Yapi and M. S. Allili},
url = {https://www.scopus.com/inward/record.uri?eid=2-s2.0-85143638804&doi=10.1109%2fICPR56361.2022.9956448&partnerID=40&md5=3fe34da98f314b85014059630e4c8c6c},
doi = {10.1109/ICPR56361.2022.9956448},
isbn = {978-1-66549-062-7},
year = {2022},
date = {2022-01-01},
booktitle = {Proceedings - International Conference on Pattern Recognition},
volume = {2022-August},
pages = {464–469},
publisher = {Institute of Electrical and Electronics Engineers Inc.},
abstract = {We present a unified statistical model for multivariate and multi-modal texture representation. This model is based on the formalism of finite mixtures of multivariate generalized Gaussians (MoMGG) which enables a compact and accurate representation of joint statistics of different sub-bands of multireslotion texture transform. This representation expresses correlation between sub-bands at different scales and orientations, and also between adjacent locations within the same subbands, providing a precise description of the texture layout. It enables also to combine different multi-scale transforms to build a richer and more representative texture signature. We successfully tested the model on traditional texture transforms such as wavelets and contourlets. Experiments on color-texture image retrieval have demonstrated the performance of our approach comparatively to state-of-art methods. © 2022 IEEE.},
note = {ISSN: 10514651},
keywords = {Color texture retrieval, Finite mixtures, Gaussian distribution, Gaussians, Image retrieval, Image texture, Mixture of multivariate generalized gaussians, Multi band, Multi-scale Decomposition, Multivariate generalized gaussian distributions, Statistic modeling, Subbands, Texture models, Textures},
pubstate = {published},
tppubtype = {inproceedings}
}
Messaoudi, H.; Belaid, A.; Allaoui, M. L.; Zetout, A.; Allili, M. S.; Tliba, S.; Salem, D. Ben; Conze, P. -H.
Efficient Embedding Network for 3D Brain Tumor Segmentation Article de journal
Dans: Lecture Notes in Computer Science (including subseries Lecture Notes in Artificial Intelligence and Lecture Notes in Bioinformatics), vol. 12658 LNCS, p. 252–262, 2021, ISSN: 03029743, (ISBN: 9783030720834 Publisher: Springer Science and Business Media Deutschland GmbH).
Résumé | Liens | BibTeX | Étiquettes: 3D medical image processing, Brain, Brain tumor segmentation, Classification networks, Convolutional neural networks, Deep learning, Embedding network, Image segmentation, Large dataset, Large datasets, Medical imaging, Natural images, Net networks, Semantic segmentation, Semantics, Signal encoding, Tumors
@article{messaoudi_efficient_2021,
title = {Efficient Embedding Network for 3D Brain Tumor Segmentation},
author = {H. Messaoudi and A. Belaid and M. L. Allaoui and A. Zetout and M. S. Allili and S. Tliba and D. Ben Salem and P. -H. Conze},
editor = {Bakas S. Crimi A.},
url = {https://www.scopus.com/inward/record.uri?eid=2-s2.0-85107387134&doi=10.1007%2f978-3-030-72084-1_23&partnerID=40&md5=b3aa3516b0465a1bf5611db4727d95f1},
doi = {10.1007/978-3-030-72084-1_23},
issn = {03029743},
year = {2021},
date = {2021-01-01},
journal = {Lecture Notes in Computer Science (including subseries Lecture Notes in Artificial Intelligence and Lecture Notes in Bioinformatics)},
volume = {12658 LNCS},
pages = {252–262},
abstract = {3D medical image processing with deep learning greatly suffers from a lack of data. Thus, studies carried out in this field are limited compared to works related to 2D natural image analysis, where very large datasets exist. As a result, powerful and efficient 2D convolutional neural networks have been developed and trained. In this paper, we investigate a way to transfer the performance of a two-dimensional classification network for the purpose of three-dimensional semantic segmentation of brain tumors. We propose an asymmetric U-Net network by incorporating the EfficientNet model as part of the encoding branch. As the input data is in 3D, the first layers of the encoder are devoted to the reduction of the third dimension in order to fit the input of the EfficientNet network. Experimental results on validation and test data from the BraTS 2020 challenge demonstrate that the proposed method achieve promising performance. © 2021, Springer Nature Switzerland AG.},
note = {ISBN: 9783030720834
Publisher: Springer Science and Business Media Deutschland GmbH},
keywords = {3D medical image processing, Brain, Brain tumor segmentation, Classification networks, Convolutional neural networks, Deep learning, Embedding network, Image segmentation, Large dataset, Large datasets, Medical imaging, Natural images, Net networks, Semantic segmentation, Semantics, Signal encoding, Tumors},
pubstate = {published},
tppubtype = {article}
}
Nouboukpo, A.; Allili, M. S.
Weakly Semi Supervised learning based Mixture Model With Two-Level Constraints Proceedings Article
Dans: A., Premaratne K. Benferhot S. Antonucci (Ed.): Proceedings of the International Florida Artificial Intelligence Research Society Conference, FLAIRS, Florida Online Journals, University of Florida, 2021, (ISSN: 23340754).
Résumé | Liens | BibTeX | Étiquettes: Classification and clustering, Group structure, Learn+, Mixture components, Mixture modeling, Mixtures, Multilevels, Number of class, Prior-knowledge, Semi-supervised learning, Supervised learning, Unlabeled data
@inproceedings{nouboukpo_weakly_2021,
title = {Weakly Semi Supervised learning based Mixture Model With Two-Level Constraints},
author = {A. Nouboukpo and M. S. Allili},
editor = {Premaratne K. Benferhot S. Antonucci A.},
url = {https://www.scopus.com/inward/record.uri?eid=2-s2.0-85131143535&doi=10.32473%2fflairs.v34i1.128490&partnerID=40&md5=21cda84d36649f4835be079ea2566717},
doi = {10.32473/flairs.v34i1.128490},
year = {2021},
date = {2021-01-01},
booktitle = {Proceedings of the International Florida Artificial Intelligence Research Society Conference, FLAIRS},
volume = {34},
publisher = {Florida Online Journals, University of Florida},
abstract = {We propose a new weakly supervised approach for classification and clustering based on mixture models. Our approach integrates multi-level pairwise group and class constraints between samples to learn the underlying group structure of the data and propagate (scarce) initial labels to unlabelled data. Our algorithm assumes the number of classes is known but does not assume any prior knowledge about the number of mixture components in each class. Therefore, our model: (1) allocates multiple mixture components to individual classes, (2) estimates automatically the number of components of each class, 3) propagates class labels to unlabelled data in a consistent way to predefined constraints. Experiments on several real-world and synthetic data datasets show the robustness and performance of our model over state-of-the-art methods. © 2021 by the authors. All rights reserved.},
note = {ISSN: 23340754},
keywords = {Classification and clustering, Group structure, Learn+, Mixture components, Mixture modeling, Mixtures, Multilevels, Number of class, Prior-knowledge, Semi-supervised learning, Supervised learning, Unlabeled data},
pubstate = {published},
tppubtype = {inproceedings}
}
Saidani, N.; Adi, K.; Allili, M. S.
Semantic Representation Based on Deep Learning for Spam Detection Article de journal
Dans: Lecture Notes in Computer Science (including subseries Lecture Notes in Artificial Intelligence and Lecture Notes in Bioinformatics), vol. 12056 LNCS, p. 72–81, 2020, ISSN: 03029743, (ISBN: 9783030453701 Publisher: Springer).
Résumé | Liens | BibTeX | Étiquettes: Conceptual views, Deep learning, E-mail spam, Electronic mail, Email content, Learning techniques, Second level, Semantic analysis, Semantic representation, Semantics, Spam detection
@article{saidani_semantic_2020,
title = {Semantic Representation Based on Deep Learning for Spam Detection},
author = {N. Saidani and K. Adi and M. S. Allili},
editor = {Barbeau M. Laborde R. Benzekri A.},
url = {https://www.scopus.com/inward/record.uri?eid=2-s2.0-85083960781&doi=10.1007%2f978-3-030-45371-8_5&partnerID=40&md5=95eba44c33557354be0900bfd2565ca9},
doi = {10.1007/978-3-030-45371-8_5},
issn = {03029743},
year = {2020},
date = {2020-01-01},
journal = {Lecture Notes in Computer Science (including subseries Lecture Notes in Artificial Intelligence and Lecture Notes in Bioinformatics)},
volume = {12056 LNCS},
pages = {72–81},
abstract = {This paper addresses the email spam filtering problem by proposing an approach based on two levels text semantic analysis. In the first level, a deep learning technique, based on Word2Vec is used to categorize emails by specific domains (e.g., health, education, finance, etc.). This enables a separate conceptual view for spams in each domain. In the second level, we extract a set of latent topics from email contents and represent them by rules to summarize the email content into compact topics discriminating spam from legitimate emails in an efficient way. The experimental study shows promising results in term of the precision of the spam detection. © 2020, Springer Nature Switzerland AG.},
note = {ISBN: 9783030453701
Publisher: Springer},
keywords = {Conceptual views, Deep learning, E-mail spam, Electronic mail, Email content, Learning techniques, Second level, Semantic analysis, Semantic representation, Semantics, Spam detection},
pubstate = {published},
tppubtype = {article}
}